Geodesic Weighted Bayesian Model For Salient Object Detection
2015 IEEE INTERNATIONAL CONFERENCE ON IMAGE PROCESSING (ICIP)(2015)
摘要
In recent years, a variety of salient object detection methods under Bayesian framework have been proposed and many achieved state of the art. However, those ignore spatial relationships and thus background regions similar to the objects are also highlighted. In this paper, we propose a novel geodesic weighted Bayesian model to address this issue. We consider spatial relationships by attaching more importance to regions which are more likely to be parts of a salient object, thus suppressing background regions. First, we learn a combined similarity via multiple features to measure similarity of adjacent regions. Then, we apply the combined similarity as edge weight to construct an undirected weighted graph and compute geodesic distance. Last, we utilize the geodesic distance to weight the observation likelihood to infer a more precise saliency map. Experiments on several benchmark datasets demonstrate the effectiveness of our model.
更多查看译文
关键词
Salient object detection,geodesic weight,Bayesian framework,superpixel
AI 理解论文
溯源树
样例
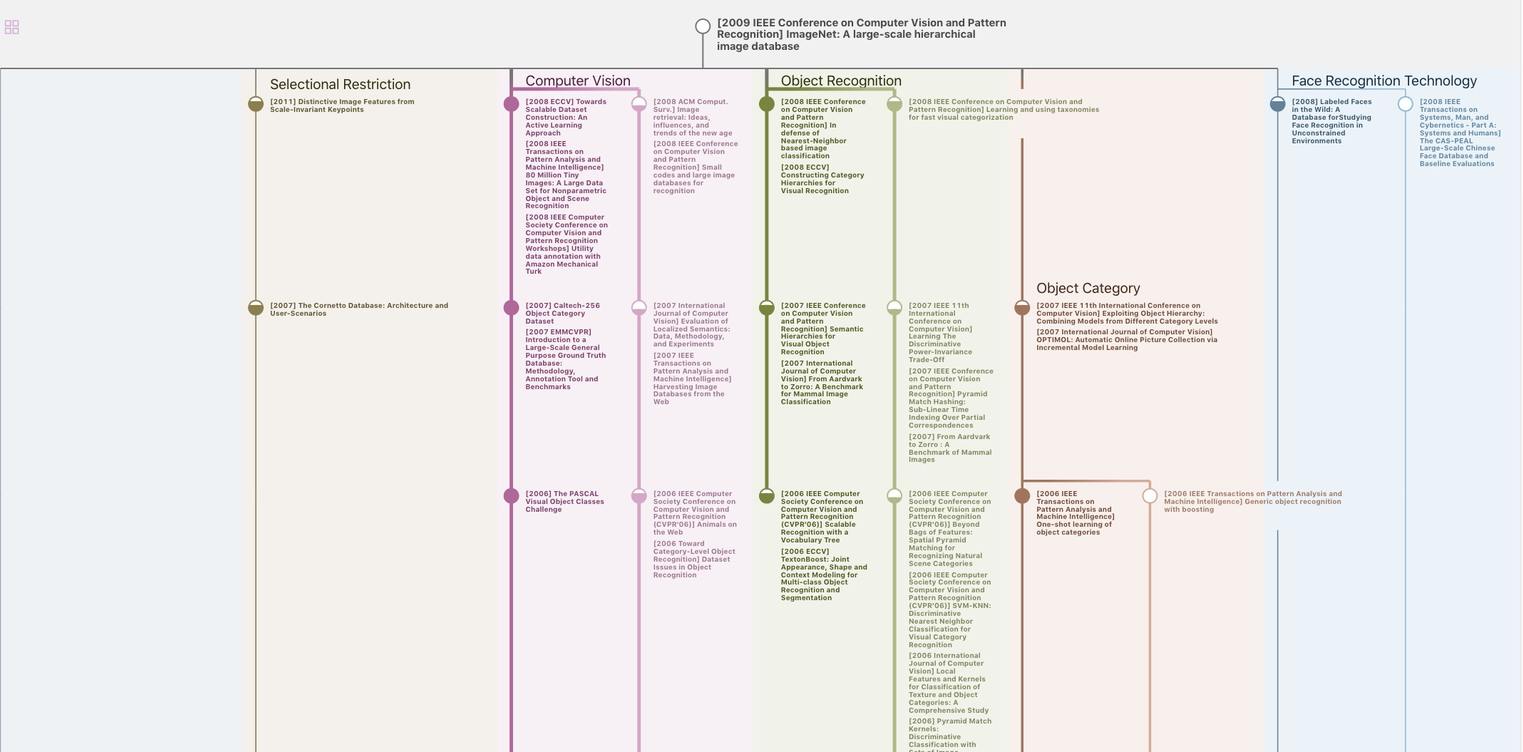
生成溯源树,研究论文发展脉络
Chat Paper
正在生成论文摘要