Niched evolutionary techniques for performance, energy, and temperature optimized scheduling in multi-core systems.
IGSC(2015)
摘要
Multi-objective evolutionary algorithms (MOEAs) are effective techniques for solving the DVFS-enabled performance (P), energy (E), and temperature (T) optimized scheduling (PETOS) problem. There are several MOEA techniques proposed in the literature for general multi-objective optimization. For example, SPEA-II is efficient for solving the PETOS problem. Another technique is the NSGA-II algorithm that has been shown to generate very good solutions. This paper proposes a novel approach that, instead of using one particular optimization, uses a hybrid of these techniques. The goal is to come up with a scheme that will take the advantages of the strength of each baseline technique to obtain a more accurate Pareto-optimal front with higher accuracy and better quality of solutions. We design five such techniques, which include designing a niched fitness assignment strategy, combining populations from multiple MOEAs, and incorporating the problem knowledge into the conventional SPEA-II approach. By comparing these techniques using a well-known metric for MOEAs as well as a modified metric to estimate the quality of Pareto fronts, we show that the proposed approach is a better optimization technique for the PETOS problem.
更多查看译文
关键词
Evolutionary algorithms, energy-efficient computing, thermal-efficient computing, task allocation, task graphs, static scheduling
AI 理解论文
溯源树
样例
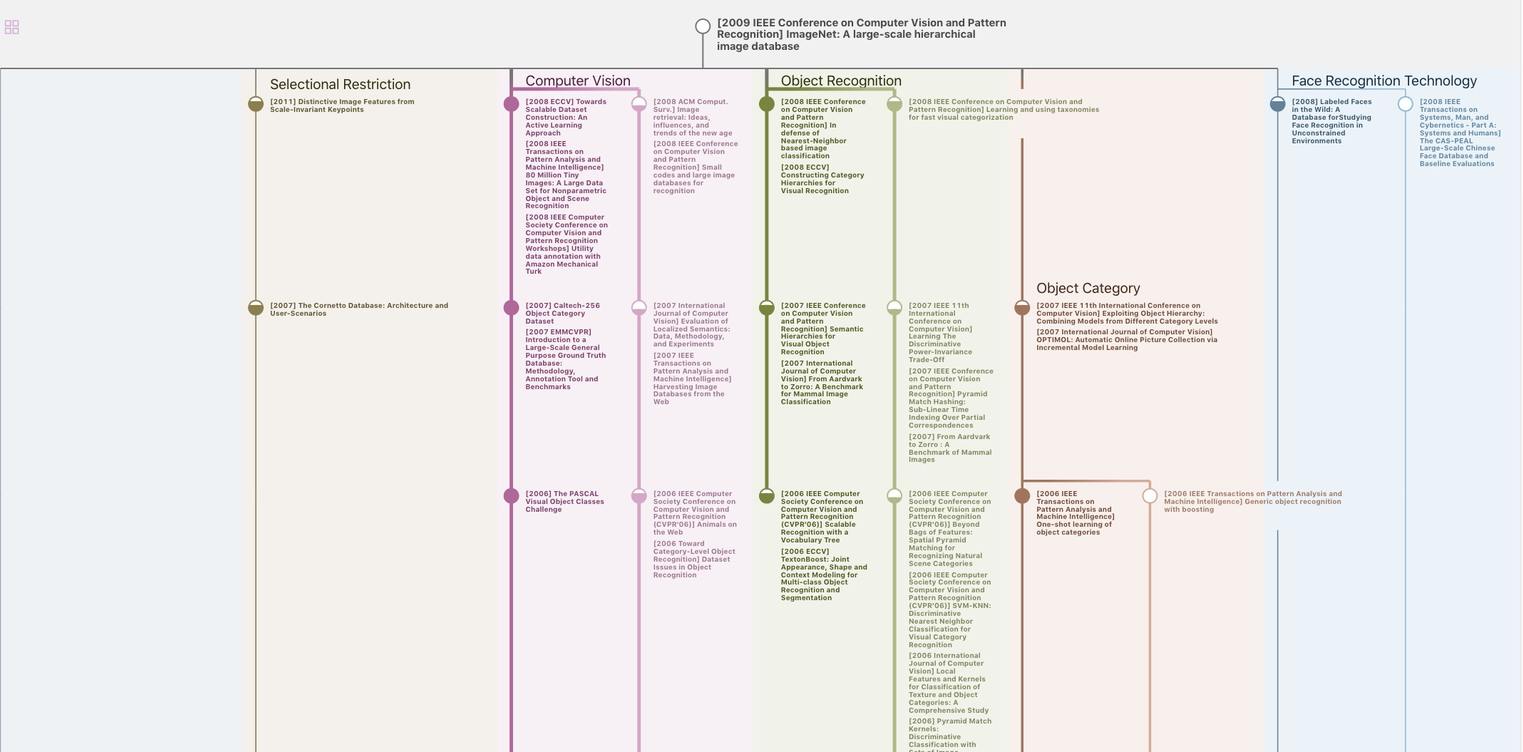
生成溯源树,研究论文发展脉络
Chat Paper
正在生成论文摘要