Robust and Discriminative Concept Factorization for Image Representation
ICMR(2015)
摘要
Concept Factorization (CF), as a variant of Nonnegative Matrix Factorization (NMF), has been widely used for learning compact representation for images because of its psychological and physiological interpretation of naturally occurring data. And graph regularization has been incorporated into the objective function of CF to exploit the intrinsic low-dimensional manifold structure, leading to better performance. But some shortcomings are shared by existing CF methods. 1) The squared loss used to measure the data reconstruction quality is sensitive to noise in image data. 2) The graph regularization may lead to trivial solution and scale transfer problems for CF such that the learned representation is meaningless. 3) Existing methods mostly ignore the discriminative information in image data. In this paper, we propose a novel method, called Robust and Discriminative Concept Factorization (RDCF) for image representation. Specifically, RDCF explicitly considers the influence of noise by imposing a sparse error matrix, and exploits the discriminative information by approximate orthogonal constraints which can also lead to nontrivial solution. We propose an iterative multiplicative updating rule for the optimization of RDCF and prove the convergence. Experiments on 5 benchmark image datasets show that RDCF can significantly out-perform several state-of-the-art related methods, which validates the effectiveness of RDCF.
更多查看译文
关键词
Concept Factorization, Graph Regularization, Discriminability, Noise, Image Representation
AI 理解论文
溯源树
样例
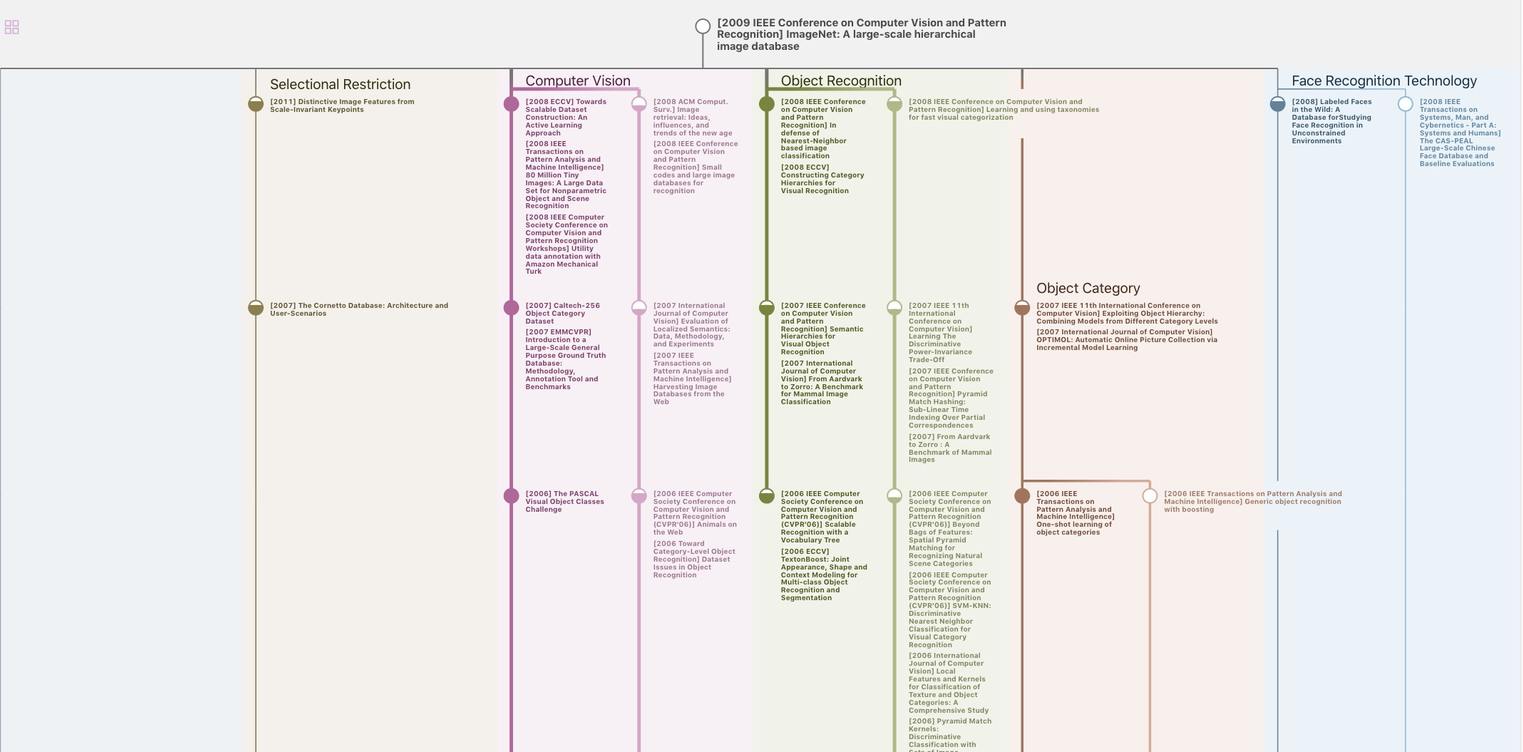
生成溯源树,研究论文发展脉络
Chat Paper
正在生成论文摘要