Integrated Analysis Of Multiple High-Dimensional Data Sets By Joint Rank-1 Matrix Approximations
2015 54TH IEEE CONFERENCE ON DECISION AND CONTROL (CDC)(2015)
摘要
In this work, we developed an algorithm for the integrated analysis of multiple high-dimensional data matrices based on sparse rank-one matrix approximations. The algorithm approximates multiple data matrices with rank one outer products composed of sparse left singular-vectors that are unique to each matrix and a right singular-vector that is shared by all of the data matrices. The right-singular vector represents a signal we wish to detect in the row-space of each matrix. The non-zero components of the resulting left-singular vectors identify rows of each matrix that in aggregate provide a sparse linear representation of the shared right-singular vector. This sparse representation facilitates downstream interpretation and validation of the resulting model based on the rows selected from each matrix. False discovery rate is used to select an appropriate l(1) penalty parameter that imposes sparsity on the left singular-vector but not the common right singular-vector of the joint approximation. Since a given multi-modal data set (MMDS) may contain multiple signals of interest the algorithm is iteratively applied to the residualized version of original data to sequentially capture and model each distinct signal in terms of rows from the different matrices. We show that the algorithm outperforms standard singular value decomposition over a wide range of simulation scenarios in terms of detection accuracy. Analysis of real data for ovarian and liver cancer resulted in compact gene expression signatures that were predictive of clinical outcomes and highly enriched for cancer related biology.
更多查看译文
关键词
Sparse matrices,Detectors,Standards,Approximation algorithms,Matrix decomposition,Algorithm design and analysis,Cancer
AI 理解论文
溯源树
样例
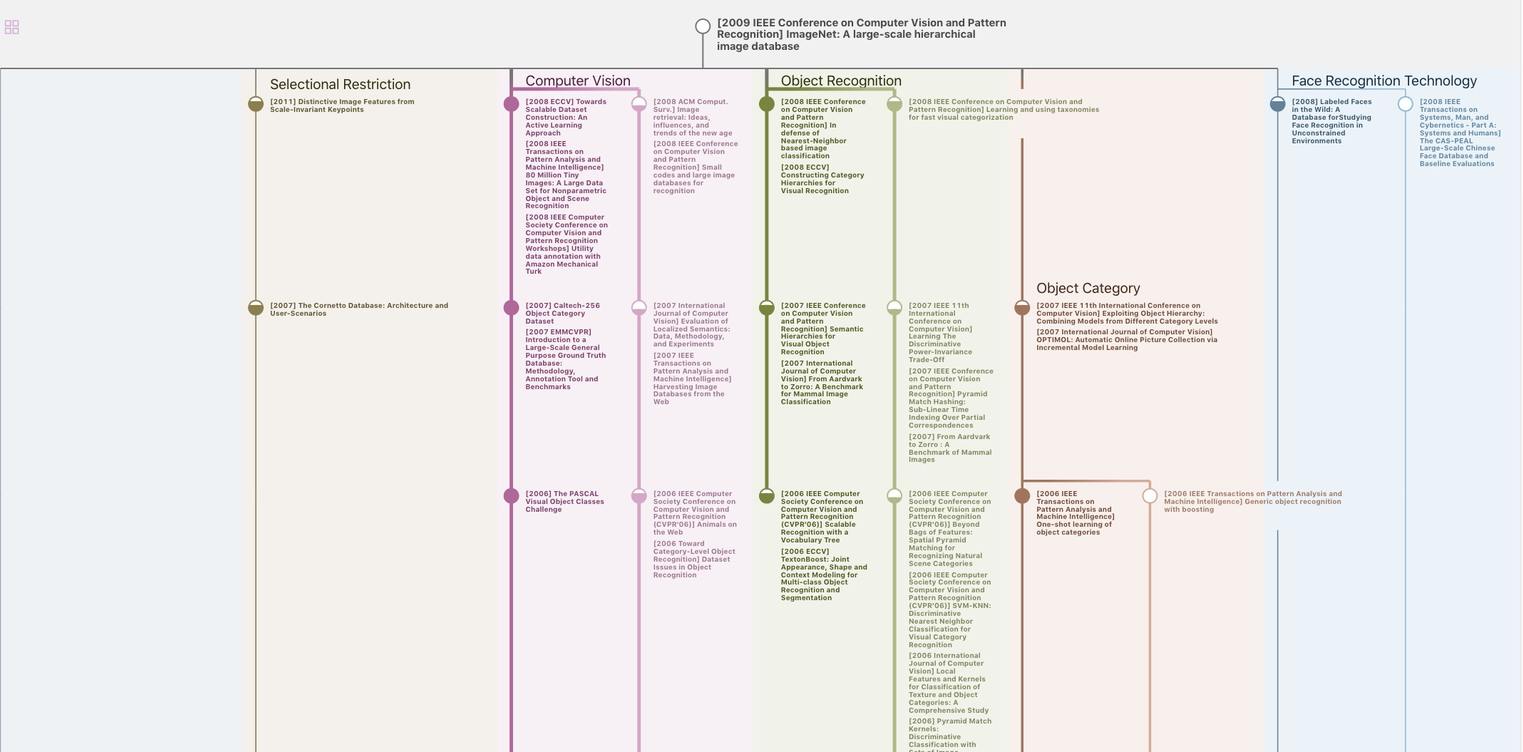
生成溯源树,研究论文发展脉络
Chat Paper
正在生成论文摘要