Learning Acoustic Frame Labeling For Speech Recognition With Recurrent Neural Networks
2015 IEEE International Conference on Acoustics, Speech and Signal Processing (ICASSP)(2015)
摘要
We explore alternative acoustic modeling techniques for large vocabulary speech recognition using Long Short-Term Memory recurrent neural networks. For an acoustic frame labeling task, we compare the conventional approach of cross-entropy (CE) training using fixed forced-alignments of frames and labels, with the Connectionist Temporal Classification (CTC) method proposed for labeling unsegmented sequence data. We demonstrate that the latter can be implemented with finite state transducers. We experiment with phones and context dependent HMM states as acoustic modeling units. We also investigate the effect of context in acoustic input by training unidirectional and bidirectional LSTM RNN models. We show that a bidirectional LSTM RNN CTC model using phone units can perform as well as an LSTM RNN model trained with CE using HMM state alignments. Finally, we also show the effect of sequence discriminative training on these models and show the first results for sMBR training of CTC models.
更多查看译文
关键词
LSTM,CTC,RNN,acoustic modeling
AI 理解论文
溯源树
样例
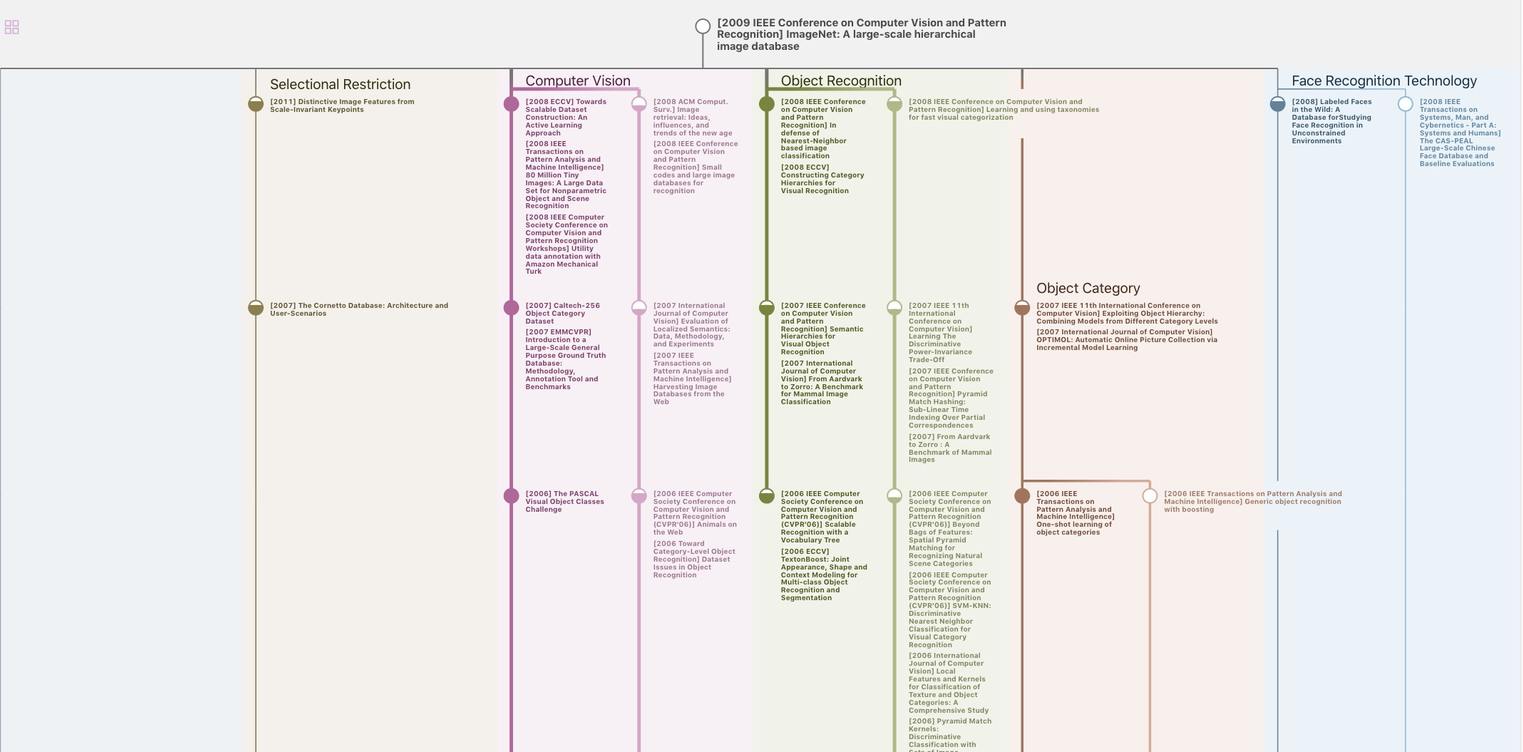
生成溯源树,研究论文发展脉络
Chat Paper
正在生成论文摘要