A Large-to-Fine-Scale Shape Prior for Probabilistic Segmentations Using A Deformable M-rep
CVPR Workshops(2008)
Abstract
Training a shape prior has been potent scheme for anatomical object segmentations, especially for images with noisy or weak intensity patterns. When the shape representation lives in a high dimensional space, principal component analysis is often used to calculate a low dimen- sional variation subspace from frequently limited number of training samples. However, the eigenmodes of the sub- space tend to keep the large-scale variation of the shape only, losing the detailed localized variability which is cru- cial to accurate segmentations. In this paper, we propose a large-to-fine-scale shape prior for probabilistic segmenta- tion to enable local refinement, using a deformable medial representation, called the m-rep. Tests on the goodness of the shape prior are carried out on large simulated data sets of a) 1000 deformed ellipsoids with mixed global deformations and local perturbation; b) 500 simulated hippocampus models. The predictabil- ity of the shape priors are evaluated and compared by a squared correlations metric and the volume overlap mea- surement against different training sample sizes. The im- proved robustness achieved by the large-to-fine-scale strat- egy is demonstrated, especially for low sample size appli- cations. Finally, posterior 3D segmentations of the bladder from CT images from multiple patients in day-to-day adap- tive radiation therapy demonstrate that the local residual statistics introduced by this method improve the segmenta- tion accuracy.
MoreTranslated text
Key words
image representation,image segmentation,medical image processing,optimisation,statistical distributions,statistical testing,anatomical object segmentations,deformable m-rep,deformable medial representation,goodness test,large-to-fine-scale shape prior,medical image segmentation,multiscale posterior optimization segmentation framework,probabilistic segmentations,probability distribution,shape representation,
AI Read Science
Must-Reading Tree
Example
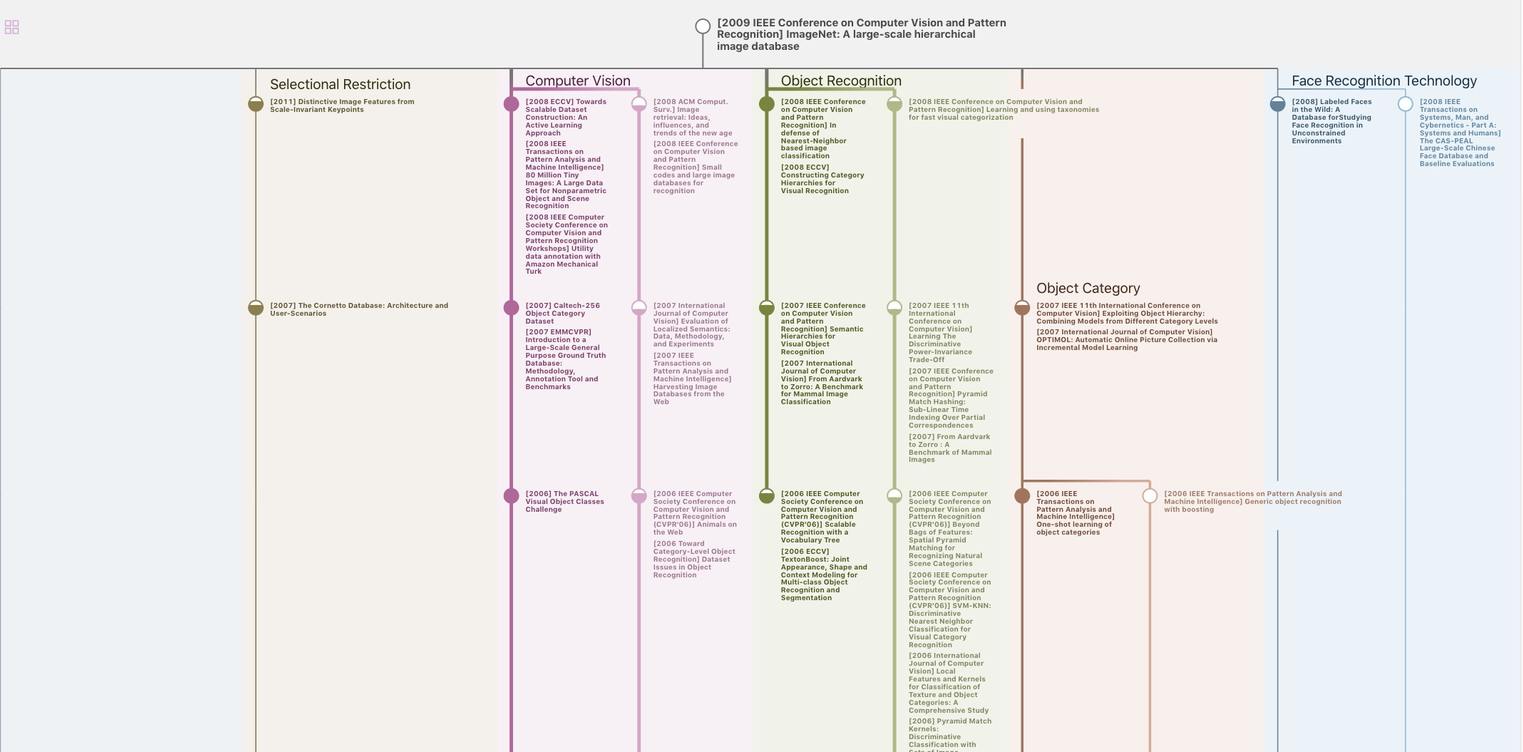
Generate MRT to find the research sequence of this paper
Chat Paper
Summary is being generated by the instructions you defined