Efficient and Accurate Approximations of Nonlinear Convolutional Networks
2015 IEEE Conference on Computer Vision and Pattern Recognition (CVPR)(2014)
摘要
This paper aims to accelerate the test-time computation of deep convolutional neural networks (CNNs). Unlike existing methods that are designed for approximating linear filters or linear responses, our method takes the nonlinear units into account. We minimize the reconstruction error of the nonlinear responses, subject to a low-rank constraint which helps to reduce the complexity of filters. We develop an effective solution to this constrained nonlinear optimization problem. An algorithm is also presented for reducing the accumulated error when multiple layers are approximated. A whole-model speedup ratio of 4x is demonstrated on a large network trained for ImageNet, while the top-5 error rate is only increased by 0.9%. Our accelerated model has a comparably fast speed as the "AlexNet", but is 4.7% more accurate.
更多查看译文
关键词
approximations,nonlinear convolutional networks,test-time computation,deep convolutional neural networks,CNN,nonlinear units,reconstruction error minimization,nonlinear responses,low-rank constraint,filters complexity,constrained nonlinear optimization problem,ImageNet,error rate,AlexNet
AI 理解论文
溯源树
样例
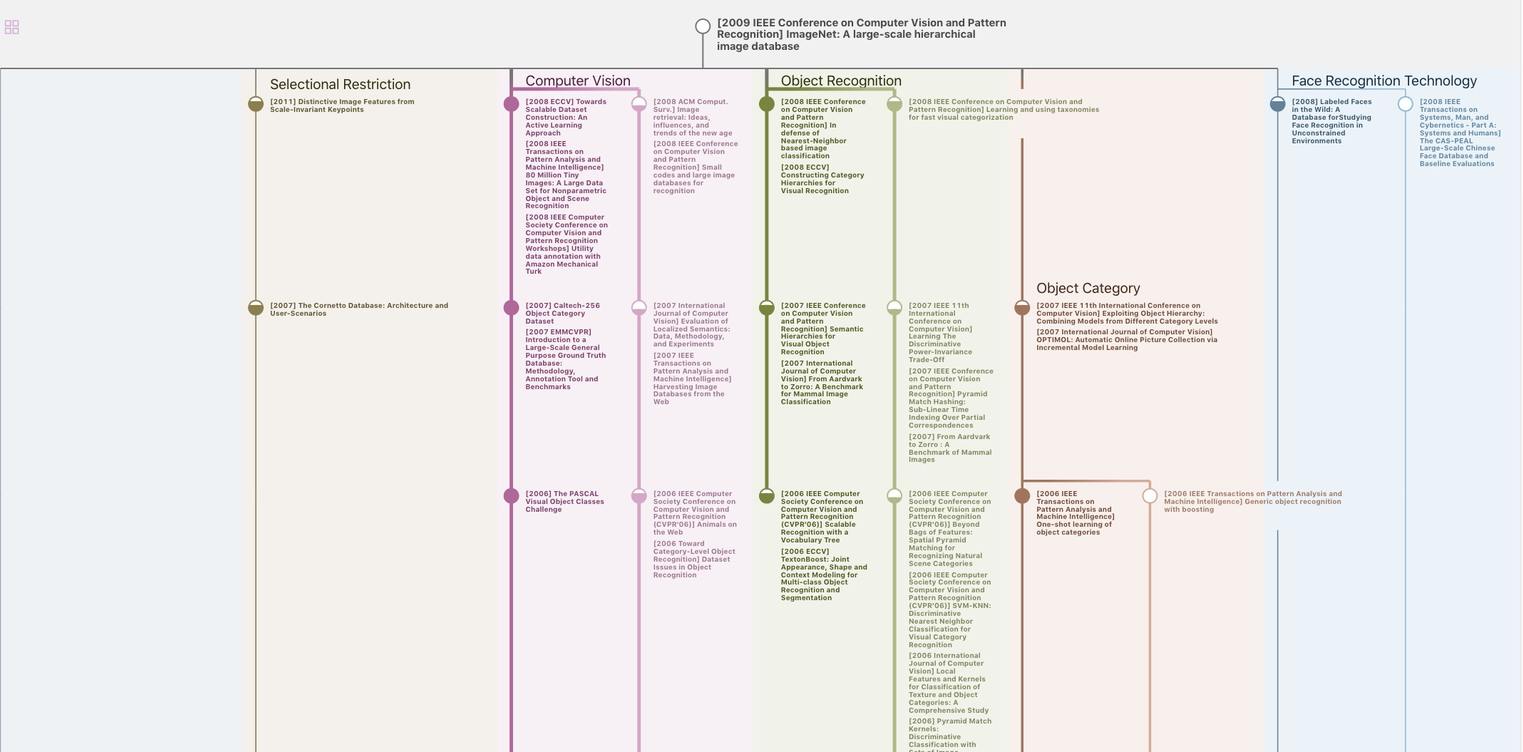
生成溯源树,研究论文发展脉络
Chat Paper
正在生成论文摘要