Ln-Annote: An Alternative Approach To Information Extraction From Emails Using Locally-Customized Named-Entity Recognition
WWW '15: 24th International World Wide Web Conference Florence Italy May, 2015(2015)
摘要
Personal mobile devices offer a growing variety of personalized services that enrich considerably the user experience. This is made possible by increased access to personal information, which to a large extent is extracted from user email messages and archives. There are, however, two main issues. First, currently these services can be offered only by large web-service companies that can also deploy email services. Second, keeping a large amount of structured personal information on the cloud raises privacy concerns. To address these problems, we propose LN-Annote, a new method to extract personal information from the email that is locally available on mobile devices (without remote access to the cloud). LN-Annote enables third-party service providers to build a question-answering system on top of the local personal information without having to own the user data. In addition, LN-Annote mitigates the privacy concerns by keeping the structured personal information directly on the personal device. Our method is based on a named-entity recognizer trained in two separate steps: first using a common dataset on the cloud and then using a personal dataset in the mobile device at hand. Our contributions include also the optimization of the implementation of LN-Annote: in particular, we implemented an OpenCL version of the custom-training algorithm to leverage the Graphic Processing Unit (GPU) available on the mobile device. We present an extensive set of experiment results: beside proving the feasibility of our approach, they demonstrate its efficiency in terms of the named-entity extraction performance as well as the execution speed and the energy consumption spent in mobile devices.
更多查看译文
关键词
NER,SNS,Personal Search,Information Extraction,Neural Network,OpenCL
AI 理解论文
溯源树
样例
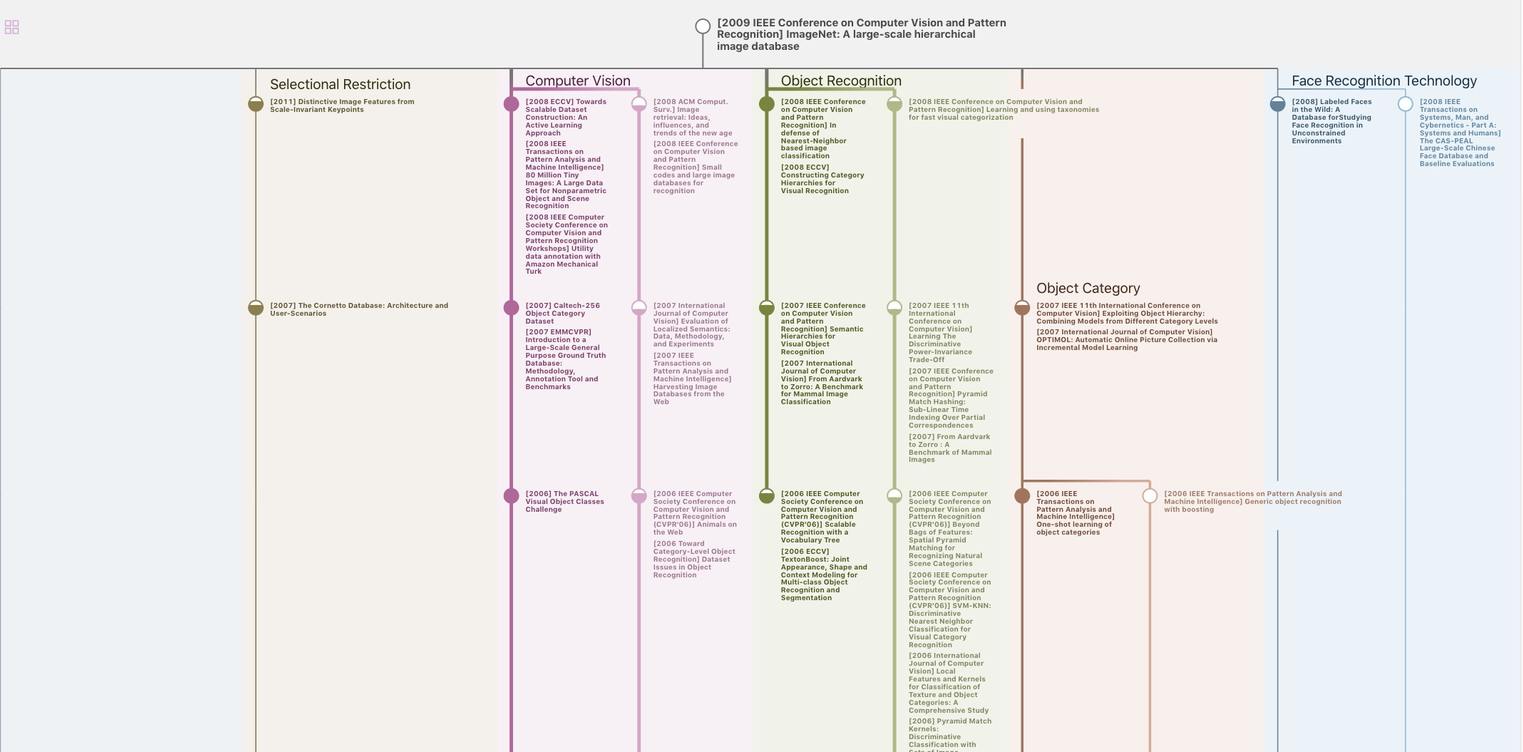
生成溯源树,研究论文发展脉络
Chat Paper
正在生成论文摘要