Protecting Locations with Differential Privacy under Temporal Correlations
CCS(2015)
摘要
Concerns on location privacy frequently arise with the rapid development of GPS enabled devices and location-based applications. While spatial transformation techniques such as location perturbation or generalization have been studied extensively, most techniques rely on syntactic privacy models without rigorous privacy guarantee. Many of them only consider static scenarios or perturb the location at single timestamps without considering temporal correlations of a moving user's locations, and hence are vulnerable to various inference attacks. While differential privacy has been accepted as a standard for privacy protection, applying differential privacy in location based applications presents new challenges, as the protection needs to be enforced on the fly for a single user and needs to incorporate temporal correlations between a user's locations. In this paper, we propose a systematic solution to preserve location privacy with rigorous privacy guarantee. First, we propose a new definition, "$\delta$-location set" based differential privacy, to account for the temporal correlations in location data. Second, we show that the well known $\ell_1$-norm sensitivity fails to capture the geometric sensitivity in multidimensional space and propose a new notion, sensitivity hull, based on which the error of differential privacy is bounded. Third, to obtain the optimal utility we present a planar isotropic mechanism (PIM) for location perturbation, which is the first mechanism achieving the lower bound of differential privacy. Experiments on real-world datasets also demonstrate that PIM significantly outperforms baseline approaches in data utility.
更多查看译文
关键词
Location privacy,Location-based services,Differential privacy,Sensitivity hull,Planar isotropic mechanism
AI 理解论文
溯源树
样例
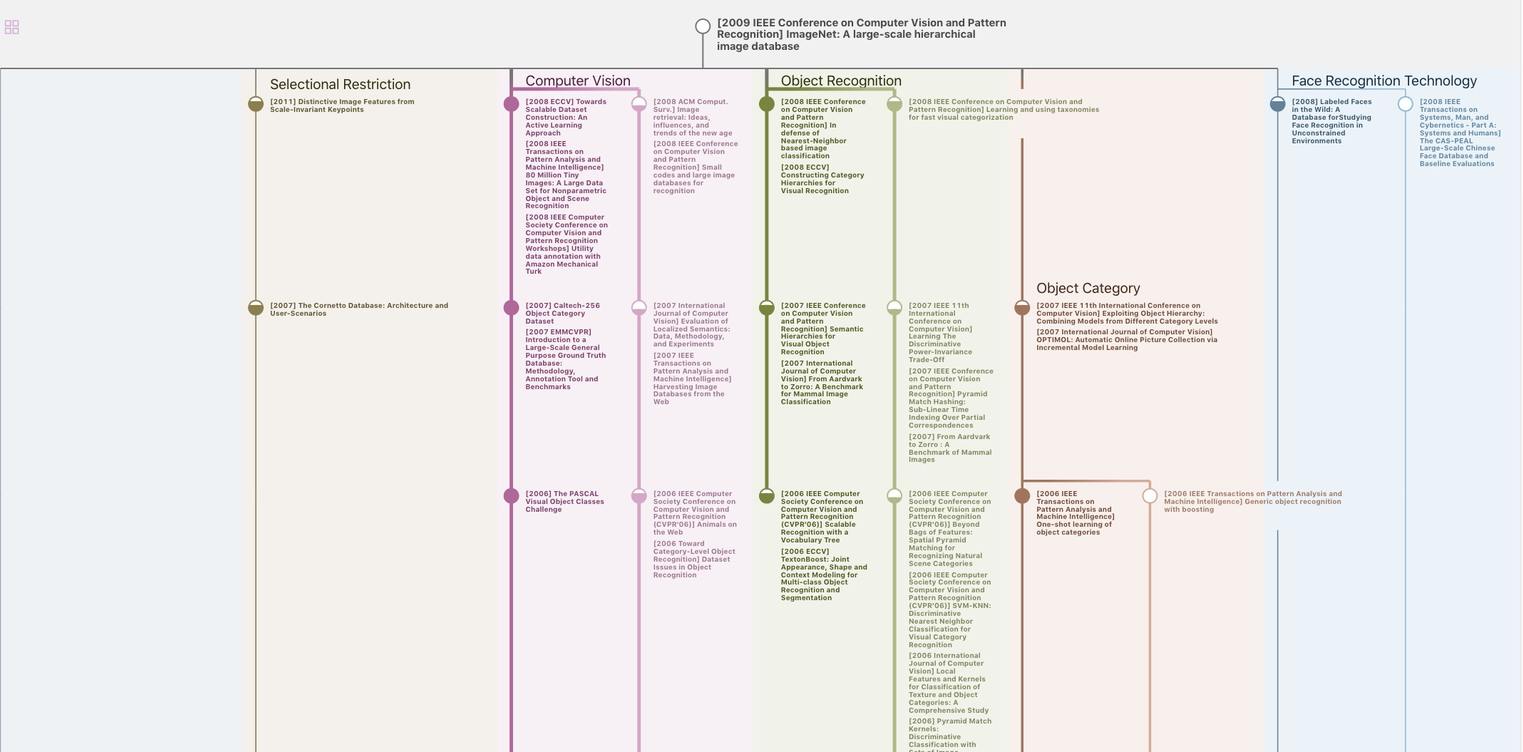
生成溯源树,研究论文发展脉络
Chat Paper
正在生成论文摘要