Approximate 3D Partial Symmetry Detection Using Co-occurrence Analysis
3DV(2015)
摘要
This paper addresses approximate partial symmetry detection in 3D point clouds, a classical and foundational tool for analyzing geometry. We present a novel, fully unsupervised method that detects partial symmetry under significant geometric variability, and without constraints on the number and arrangement of instances. The core idea is a matching scheme that finds consistent co-occurrence patterns in a frame-invariant way. We obtain a canonical partition of the input shape into building blocks and can handle ambiguous data by aggregating co-occurrence information across both all building block instances and the area they cover. We evaluate our method on several benchmark data sets and demonstrate its significant improvements in handling geometric variability, including scanning noise, irregular patterns, appearance variation and shape deformation.
更多查看译文
关键词
approximate 3D partial symmetry detection,cooccurrence analysis,3D point cloud,frame-invariant way,canonical partition,cooccurrence information aggregation,geometric variability handling,scanning noise,shape deformation
AI 理解论文
溯源树
样例
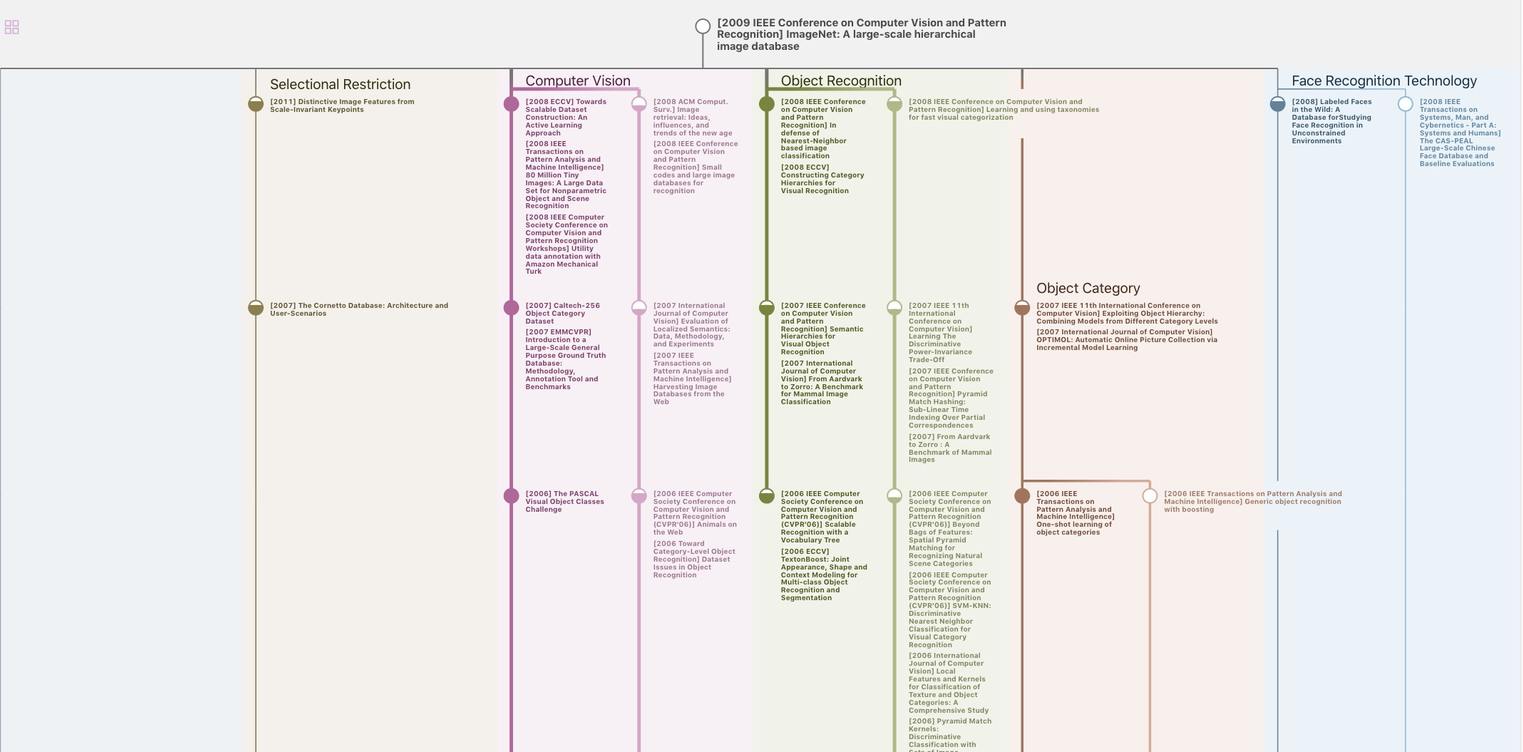
生成溯源树,研究论文发展脉络
Chat Paper
正在生成论文摘要