Convolutional Fisher Kernels for RGB-D Object Recognition
3DV(2015)
摘要
This paper studies the problem of improving object recognition using the novel RGB-D data. To address the problem, a new convolutional Fisher Kernels (CFK) method is proposed to represent RGB-D objects powerfully yet efficiently. The core idea of our approach is to integrate the both advantages of the convolutional neural networks (CNN) and Fisher Kernel encoding (FK): CNN model is flexible to adapt to new data sources, but requires for large amounts of training data with significant computational resources for good generalization, In comparison, FK encoding is able to represent objects powerfully and efficiently with small training data, however, its success highly depends on the well-designed SIFT features in literature, which may not be suitable for the new depth data. CFK can be interpreted as a two-layer feature learning structure to bridge the two models. The first layer employs a single-layer CNN to learn low-level translation ally invariant features for both RGB and depth data efficiently. The second layer aggregates the convolutional responses by FK encoding. Here 2D and 3D spatial pyramids are applied to further improve the Fisher vector representation of each modality. Experiments on RGB-D object recognition benchmarks demonstrate that our approach can achieve the state-of-the-art results.
更多查看译文
关键词
RGB-D,Recognition,Fisher Kernel,CNN
AI 理解论文
溯源树
样例
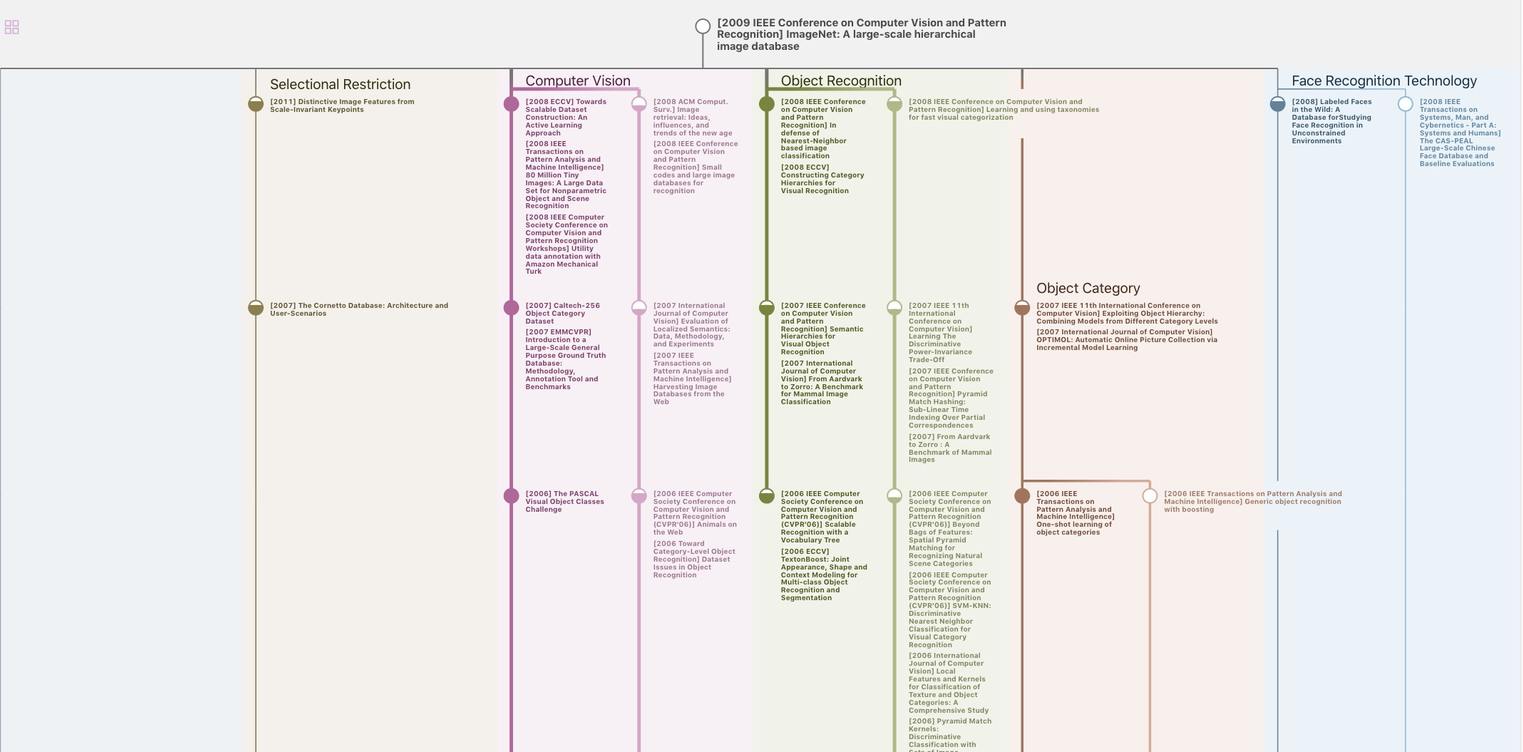
生成溯源树,研究论文发展脉络
Chat Paper
正在生成论文摘要