Predicting Drug-Target Interactions Between New Drugs and New Targets via Pairwise K-nearest Neighbor and Automatic Similarity Selection.
IScIDE(2015)
摘要
Predicting drug-target interaction DTI by computational methods has gained more and more concerns in both drug discovery and repositioning. However, several inherent difficulties in DTI data have not yet been addressed appropriately, including the powerless prediction of interactions for new drugs and/or new targets, the biased predicting model derived from imbalanced samples and the inadequate solution to missing interactions and multiple similarities. Moreover, assessed on inappropriate scenarios, existing methods may generate over-optimistic predictions. In this paper, we predict the potential interactions between new drugs and new targets based on pairwise K-nearest neighbor. With lower computational complexity, the proposed approach is able to obtain the less biased prediction and to relax the difficulty caused by missing interactions. Moreover, we develop a strategy to automatically select the best among multiple similarities to train classifiers. Based on four benchmark datasets, the effectiveness of our approach is demonstrated by an appropriate cross validation.
更多查看译文
关键词
Drug-target interaction, K nearest neighbor, Similarity selection, Missing data
AI 理解论文
溯源树
样例
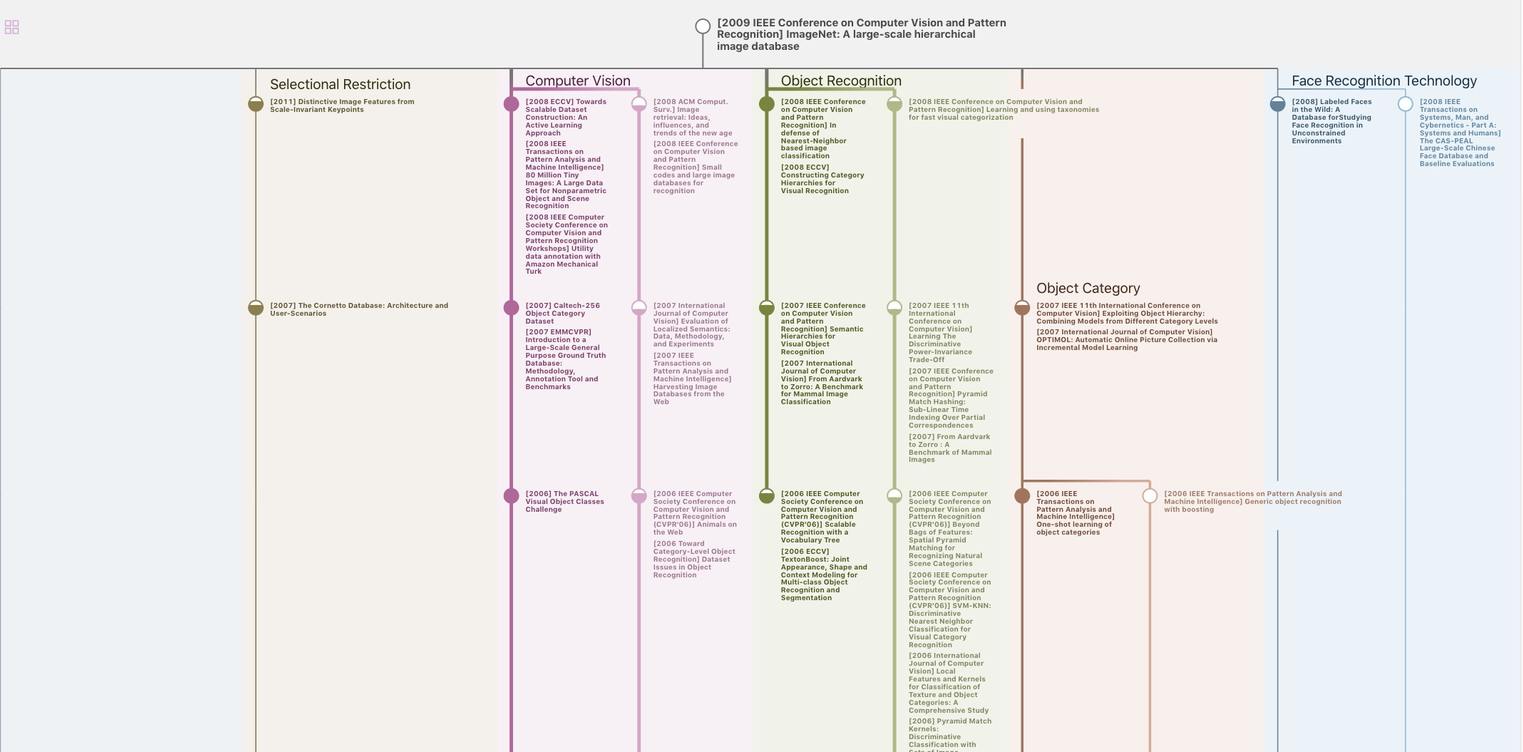
生成溯源树,研究论文发展脉络
Chat Paper
正在生成论文摘要