Graphreduce: Processing Large-Scale Graphs On Accelerator-Based Systems
SC(2015)
摘要
Recent work on real-world graph analytics has sought to leverage the massive amount of parallelism offered by GPU devices, but challenges remain due to the inherent irregularity of graph algorithms and limitations in GPU-resident memory for storing large graphs. We present GraphReduce, a highly efficient and scalable GPU-based framework that operates on graphs that exceed the device's internal memory capacity. GraphReduce adopts a combination of edge-and vertex-centric implementations of the Gather-Apply-Scatter programming model and operates on multiple asynchronous GPU streams to fully exploit the high degrees of parallelism in GPUs with efficient graph data movement between the host and device. GraphReduce-based programming is performed via device functions that include gatherMap, gatherReduce, apply, and scatter, implemented by programmers for the graph algorithms they wish to realize. Extensive experimental evaluations for a wide variety of graph inputs and algorithms demonstrate that GraphReduce significantly outperforms other competing out-of-memory approaches.
更多查看译文
关键词
Graph Analytics,Big Data,GPGPU,Performance Optimization,Data Movement Optimization
AI 理解论文
溯源树
样例
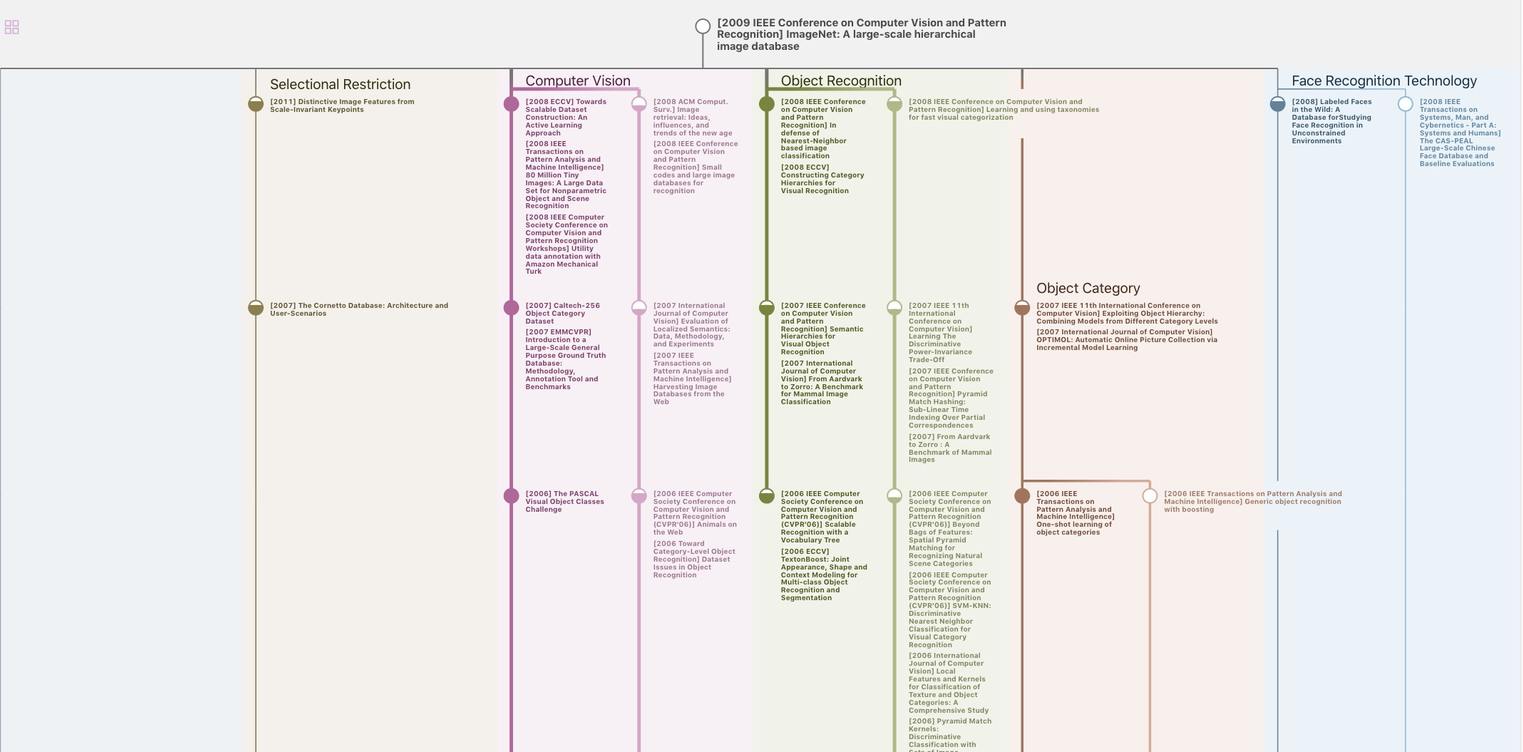
生成溯源树,研究论文发展脉络
Chat Paper
正在生成论文摘要