Improving Backfilling By Using Machine Learning To Predict Running Times
SC(2015)
摘要
The job management system is the HPC middleware responsible for distributing computing power to applications. While such systems generate an ever increasing amount of data, they are characterized by uncertainties on some parameters like the job running times. The question raised in this work is: To what extent is it possible/useful to take into account predictions on the job running times for improving the global scheduling?We present a comprehensive study for answering this question assuming the popular EASY backfilling policy. More precisely, we rely on some classical methods in machine learning and propose new cost functions well-adapted to the problem. Then, we assess our proposed solutions through intensive simulations using several production logs. Finally, we propose a new scheduling algorithm that outperforms the popular EASY backfilling algorithm by 28% considering the average bounded slowdown objective.
更多查看译文
关键词
High Performance Computing,Running Time Estimation,Scheduling,Machine Learning
AI 理解论文
溯源树
样例
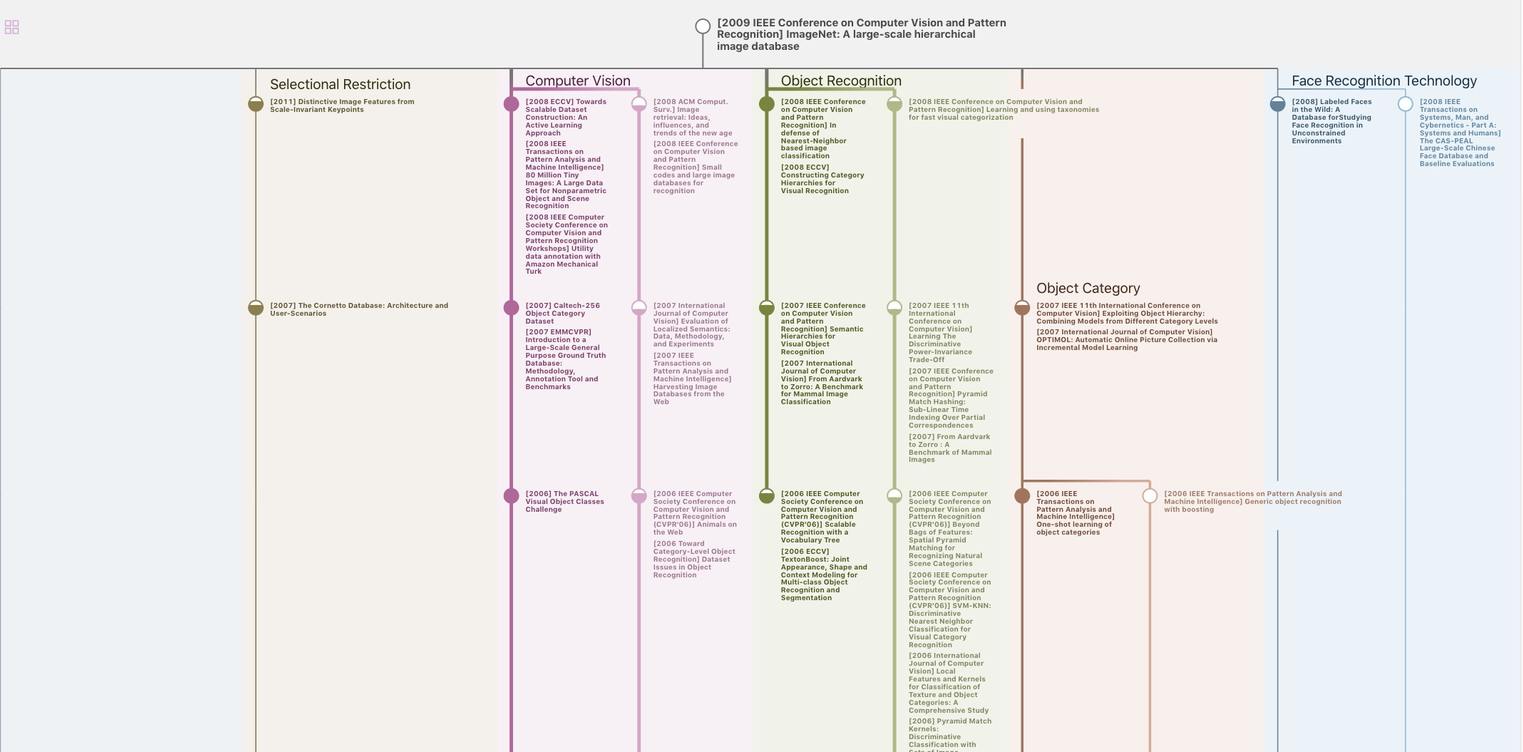
生成溯源树,研究论文发展脉络
Chat Paper
正在生成论文摘要