Incentivize Crowd Labeling Under Budget Constraint
2015 IEEE Conference on Computer Communications (INFOCOM)(2015)
摘要
Crowdsourcing systems allocate tasks to a group of workers over the Internet, which have become an effective paradigm for human-powered problem solving such as image classification, optical character recognition and proofreading. In this paper, we focus on incentivizing crowd workers to label a set of binary tasks under strict budget constraint. We properly profile the tasks' difficulty levels and workers' quality in crowdsourcing systems, where the collected labels are aggregated with sequential Bayesian approach. To stimulate workers to undertake crowd labeling tasks, the interaction between workers and the platform is modeled as a reverse auction. We reveal that the platform utility maximization could be intractable, for which an incentive mechanism that determines the winning bid and payments with polynomial-time computation complexity is developed. Moreover, we theoretically prove that our mechanism is truthful, individually rational and budget feasible. Through extensive simulations, we demonstrate that our mechanism utilizes budget efficiently to achieve high platform utility with polynomial computation complexity.
更多查看译文
关键词
polynomial-time computation complexity,incentive mechanism,platform utility maximization,crowd labeling task,sequential Bayesian approach,Internet,crowdsourcing system,budget constraint,incentivize crowd labeling
AI 理解论文
溯源树
样例
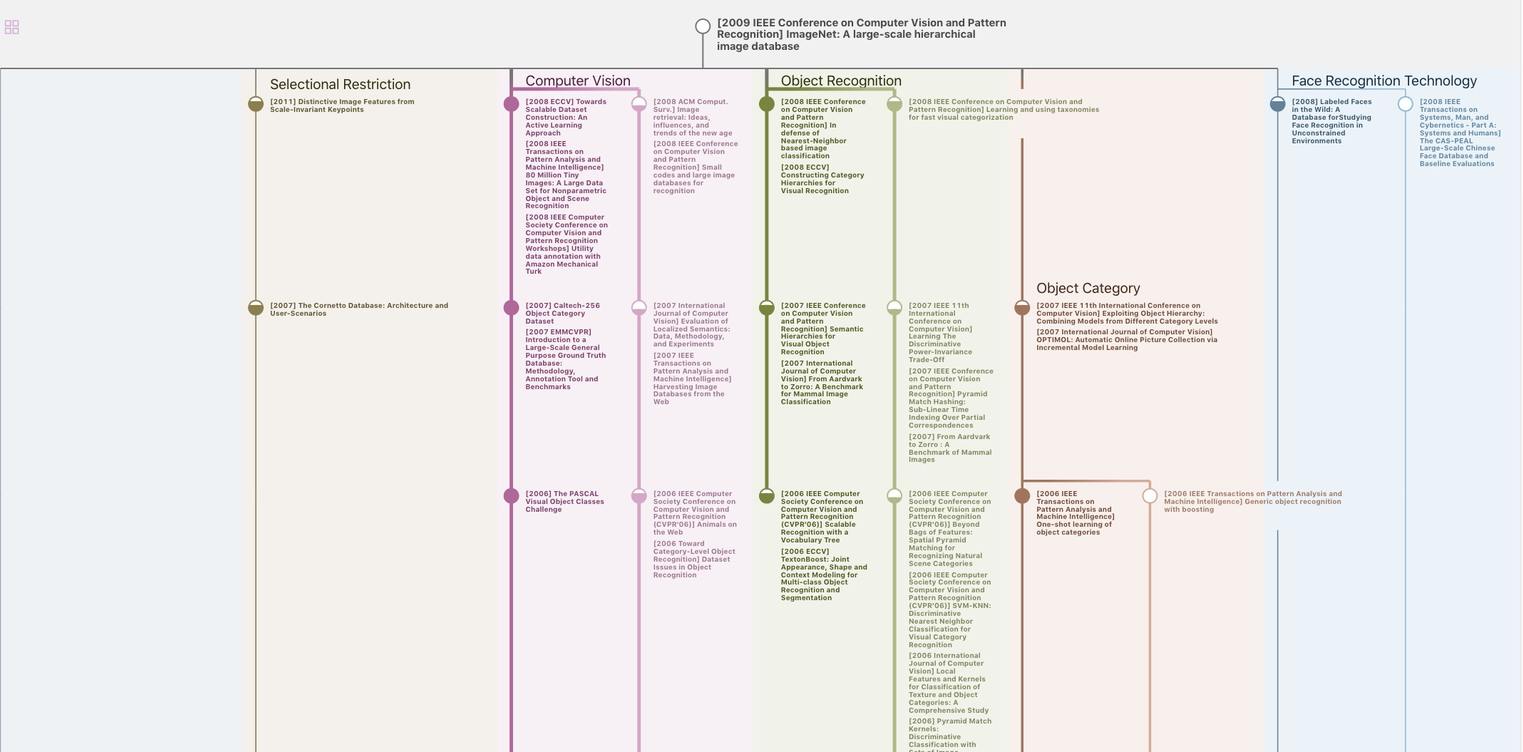
生成溯源树,研究论文发展脉络
Chat Paper
正在生成论文摘要