Topic2Vec: Learning distributed representations of topics
2015 International Conference on Asian Language Processing (IALP)(2015)
摘要
Latent Dirichlet Allocation (LDA) mining thematic structure of documents plays an important role in nature language processing and machine learning areas. However, the probability distribution from LDA only describes the statistical relationship of occurrences in the corpus and usually in practice, probability is not the best choice for feature representations. Recently, embedding methods have been proposed to represent words and documents by learning essential concepts and representations, such as Word2Vec and Doc2Vec. The embedded representations have shown more effectiveness than LDA-style representations in many tasks. In this paper, we propose the Topic2Vec approach which can learn topic representations in the same semantic vector space with words, as an alternative to probability distribution. The experimental results show that Topic2Vec achieves interesting and meaningful results.
更多查看译文
关键词
learning,topic,embedding
AI 理解论文
溯源树
样例
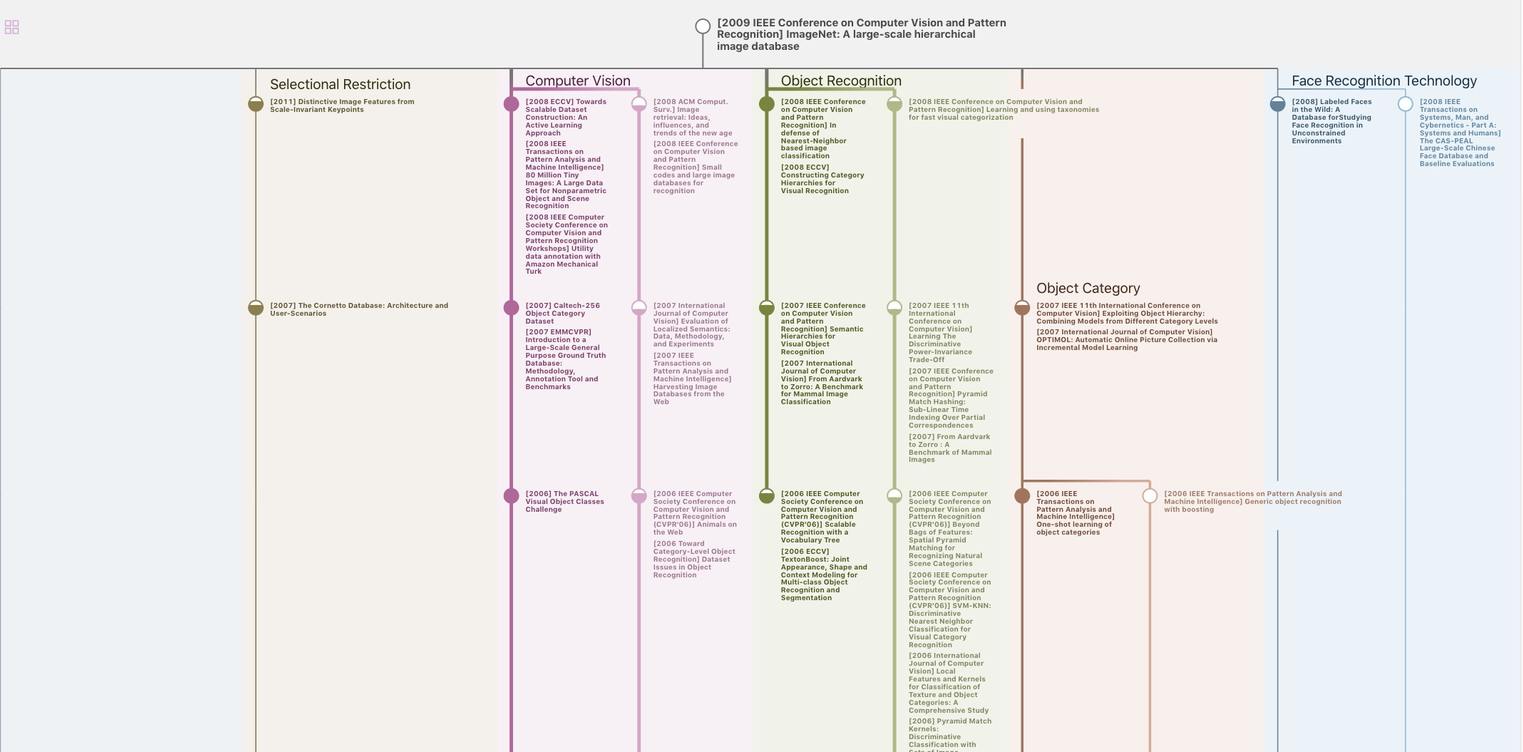
生成溯源树,研究论文发展脉络
Chat Paper
正在生成论文摘要