Sequential Model-Free Hyperparameter Tuning
2015 IEEE International Conference on Data Mining(2015)
摘要
Hyperparameter tuning is often done manually but current research has proven that automatic tuning yields effective hyperparameter configurations even faster and does not require any expertise. To further improve the search, recent publications propose transferring knowledge from previous experiments to new experiments. We adapt the sequential model-based optimization by replacing its surrogate model and acquisition function with one policy that is optimized for the task of hyperparameter tuning. This policy generalizes over previous experiments but neither uses a model nor uses meta-features, nevertheless, outperforms the state of the art. We show that a static ranking of hyperparameter combinations yields competitive results and substantially outperforms a random hyperparameter search. Thus, it is a fast and easy alternative to complex hyperparameter tuning strategies and allows practitioners to tune their hyperparameters by simply using a look-up table. We made look-up tables for two classifiers publicly available: SVM and AdaBoost. Furthermore, we propose a similarity measure for data sets that yields more comprehensible results than those using meta-features. We show how this similarity measure can be applied to surrogate models in the SMBO framework and empirically show that this change leads to better hyperparameter configurations in less trials.
更多查看译文
关键词
hyperparameter optimization,meta-learning,transfer learning
AI 理解论文
溯源树
样例
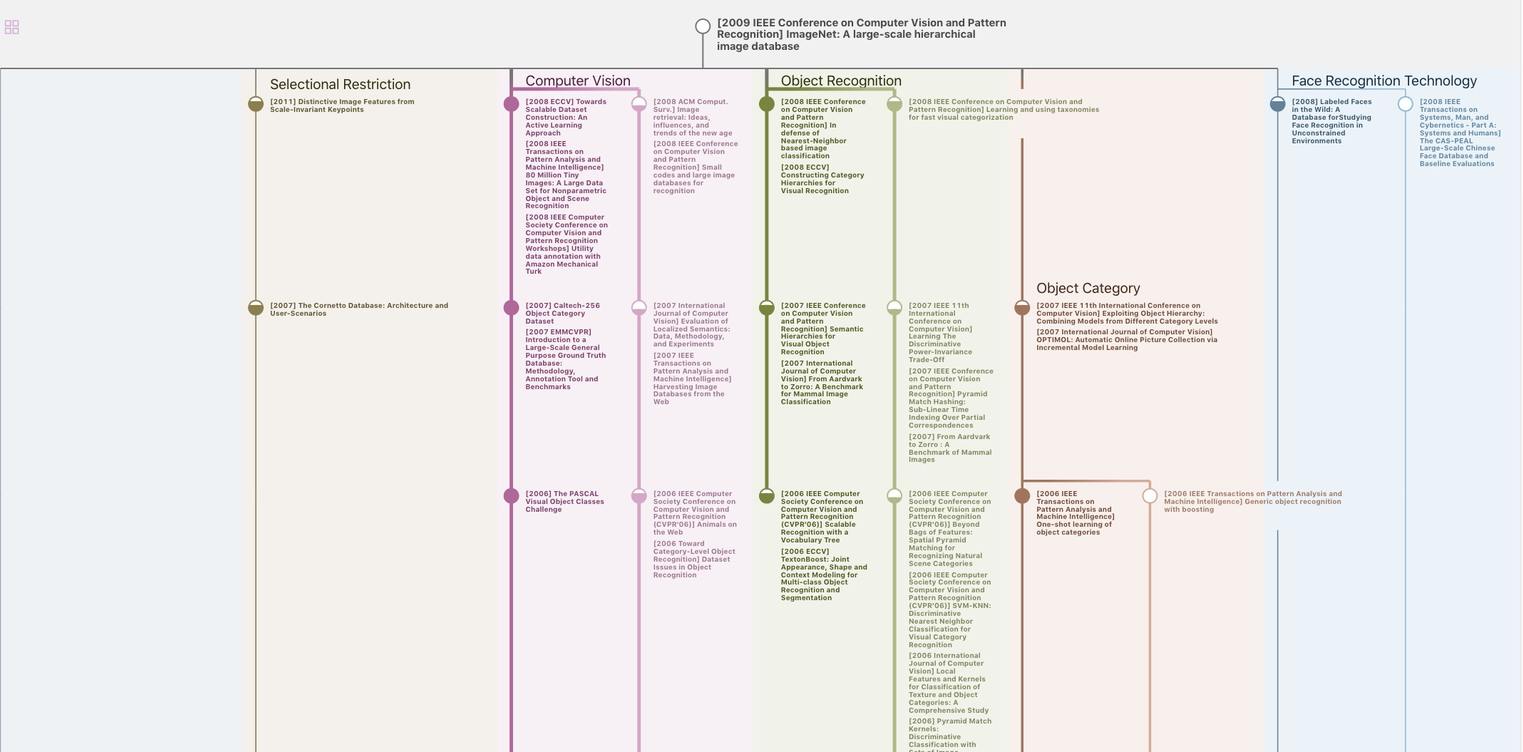
生成溯源树,研究论文发展脉络
Chat Paper
正在生成论文摘要