A Hybrid Approach Based on Particle Swarm Optimization for Echo State Network Initialization
2015 IEEE INTERNATIONAL CONFERENCE ON SYSTEMS, MAN, AND CYBERNETICS (SMC 2015): BIG DATA ANALYTICS FOR HUMAN-CENTRIC SYSTEMS(2015)
摘要
Echo state networks (ESNs) fulfill considerable promises for topology fine-tuning in supervised training. However the randomness of the setting of ESN weights initialization affects badly the learning performance. On the other side, Particle Swarm Optimization (PSO) has proven its efficiency as an optimization tool to puzzle out optimal solutions in complex space. In this work, we present an ESN architecture to which we associate a PSO algorithm to pre-train the weights within the network layers. A random distribution of the weights matrices is firstly performed. Then, these weights are pre-trained in order to fit the application requirements. Once optimized, they are re-injected into the ESN model which, in its turn, undergoes a training process followed by a test phase. A comparison between the network performances before and after optimization process is performed. Empirical results show a reduction of learning errors in the case of PSO use.
更多查看译文
关键词
Echo State Network, supervised training, Reservoir Computing, Particle Swarm Optimization
AI 理解论文
溯源树
样例
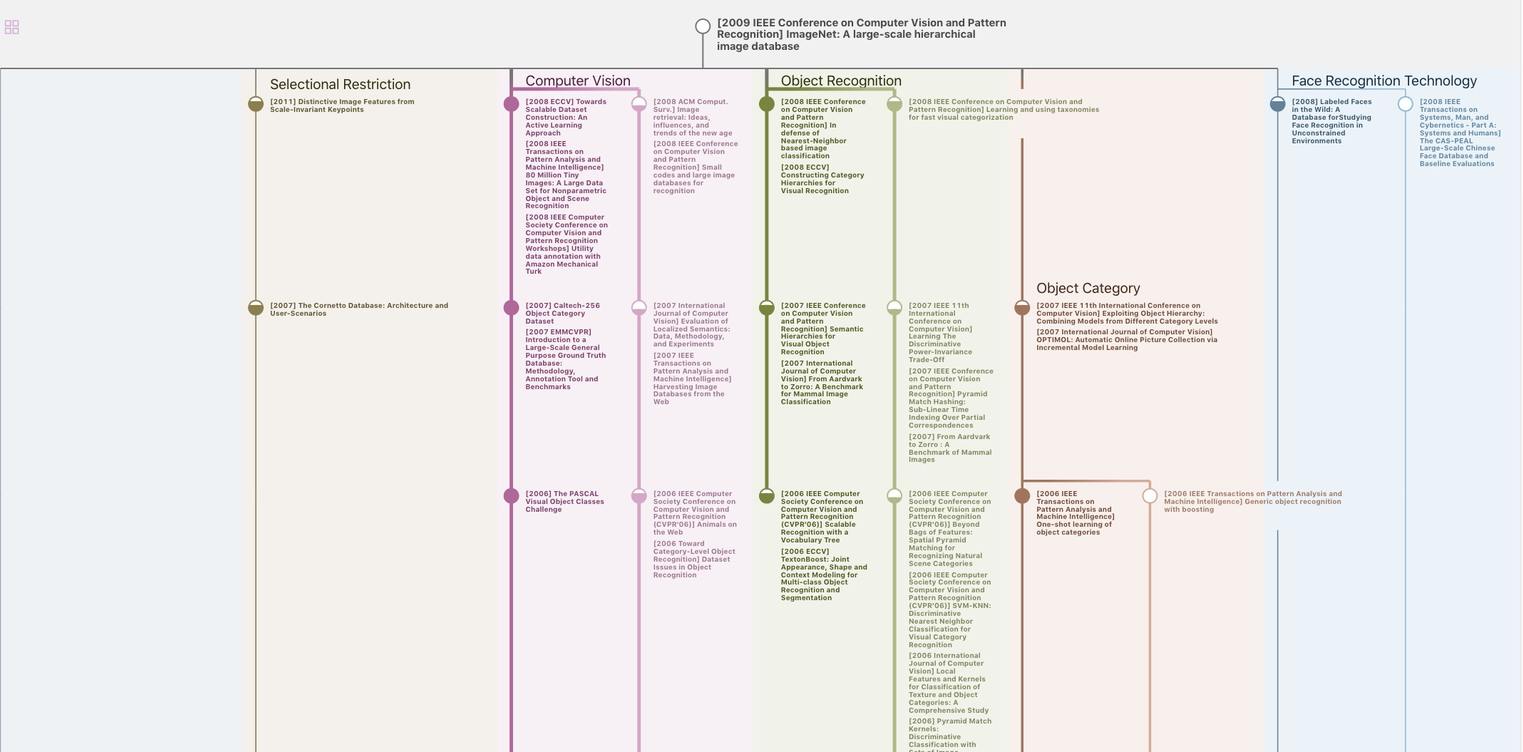
生成溯源树,研究论文发展脉络
Chat Paper
正在生成论文摘要