A Fishervoice based feature fusion method for short utterance speaker recognition
ChinaSIP(2013)
摘要
For GMM-UBM based text-independent speaker recognition, the performance decreases significantly when the utterance is getting too short, and that is mostly due to the lack of distinguishable information from a single kind of feature. Fusion of different features followed by a dimensionality reduction process has been proved useful to provide a satisfying solution. However, some fusion methods based on the traditional Linear Discriminant Analysis (LDA) may cause the singular matrix problem. Therefore, a Fishervoice based feature fusion method incorporating with the Principal Component Analysis (PCA) and the LDA is proposed, where several features, such as MFCC, PLAR and LPCC, which are commonly used, are concatenated, and then projected into a lower-dimensional subspace. Compared with the baseline GMM-UBM systems using any single feature and using the LDA based fusion method, the proposed one can effectively reduce the equal error rate and give the best performance for text-independent speaker recognition for utterances as short as about 2 seconds. © 2013 IEEE.
更多查看译文
关键词
matrix algebra,principal component analysis,sensor fusion,speaker recognition,Fishervoice based feature fusion method,GMM-UBM,LDA,PCA,linear discriminant analysis,principal component analysis,short utterance speaker recognition,singular matrix problem,text-independent speaker recognition,Feature fusion,Fishervoice,LDA,PCA,Short utterance speaker recognition,
AI 理解论文
溯源树
样例
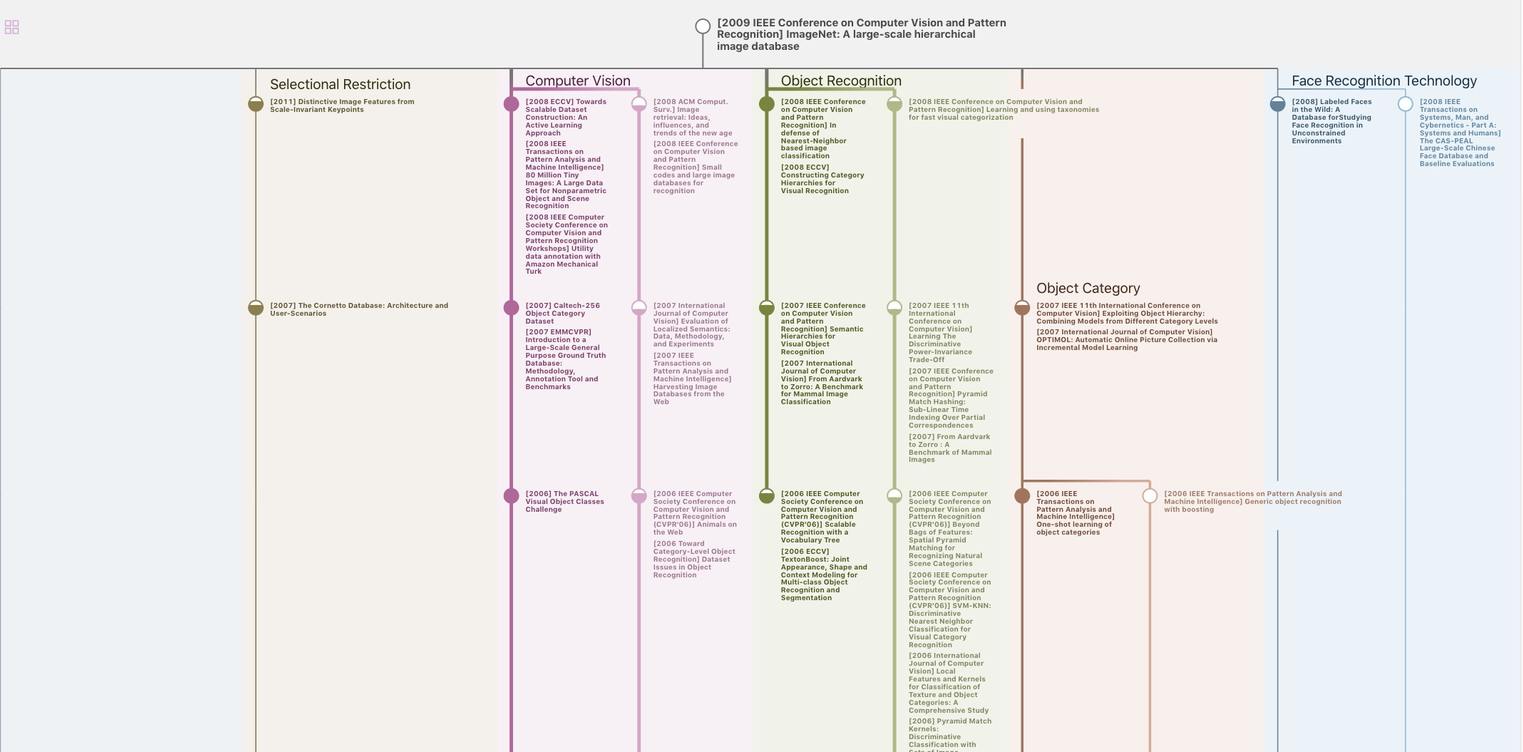
生成溯源树,研究论文发展脉络
Chat Paper
正在生成论文摘要