Siena's Clinical Decision Assistant.
TREC(2015)
摘要
This paper discusses Siena’s Clinical Decision Assistant’s (SCDA) system and its participation in the Text Retrieval Conference (TREC) Clinical Decision Support Track (CDST) of 2015. The overall goal of the 2015 track is to link medical cases to information that is pertinent to patient care. Participants were given a set of 30 topics in the form of medical case narratives and a snapshot of 733,138 articles from PubMed Central (PMC). The 30 topics were annotated into three major subsets: diagnosis, test and treatment, with ten of each type. Each topic serves as an idealized representation of actual medical records and includes both a description, which contains a complete account of the patient visit, and a summary, which is typically a one or two sentence summary of the main points in the description. SCDA used several methods to attempt improve the accuracy of medical cases retrieved. SCDA used the metathesaurus Unified Medical Language System (UMLS) that was implemented using MetaMap (NIH, 2013), query and document framing (Small and Stzalkowski 2004), a ranked fusion of document lists and Lucene for initial document indexing and retrieval. The track received a total of 178 runs from 36 different groups. We submitted three task A runs where our highest P(10) run was 0.3767 and two task B runs where our highest P(10) run was 0.4167. The highest P(10) from CDST TREC 2014 was 0.39. The word described here was performed by, and the entire SCDA system built by a team of undergraduate researchers working together for just ten weeks during the summer of 2015. The team was funded under the Siena College Institute for Artificial Intelligence’s National Science Foundation’s Research Experience for Undergraduates Grant.
更多查看译文
AI 理解论文
溯源树
样例
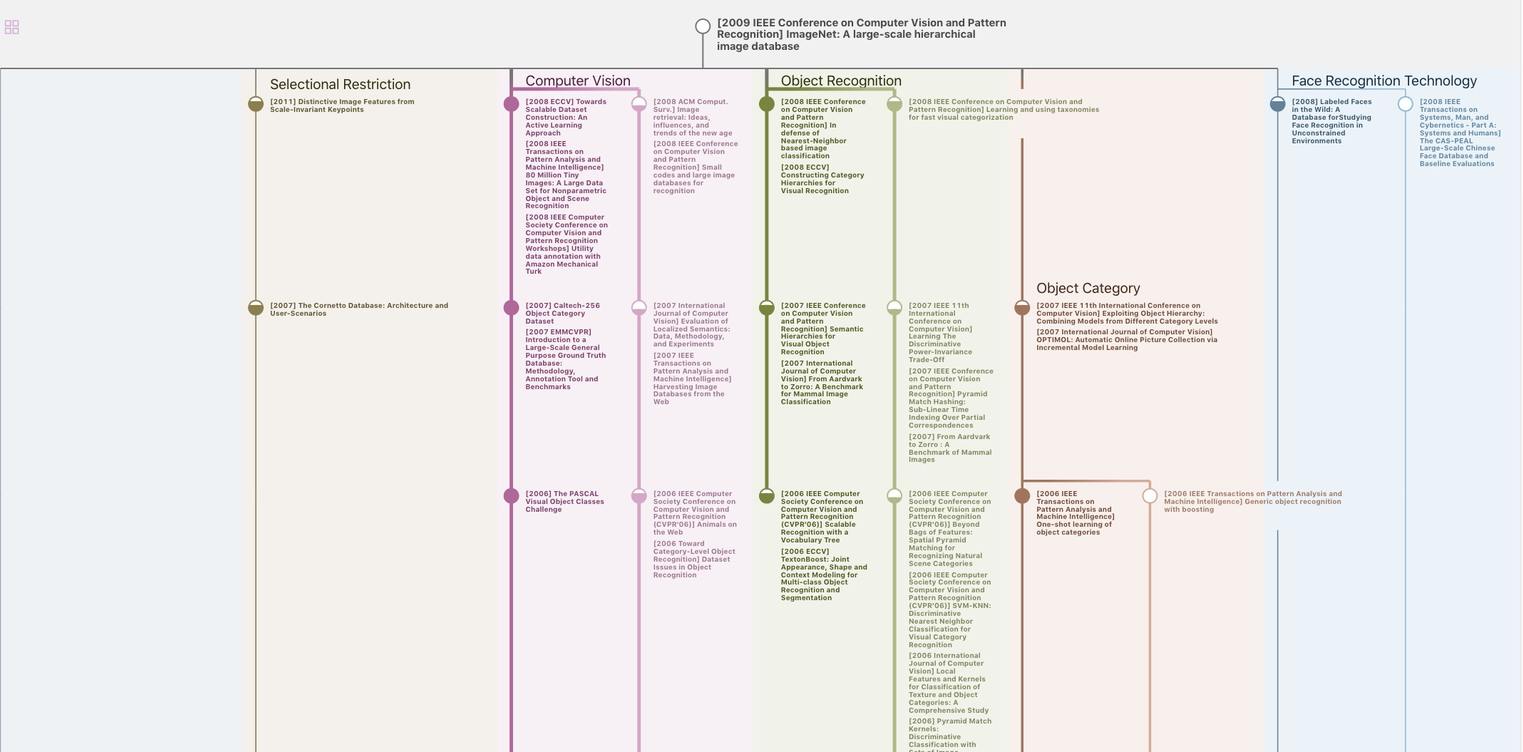
生成溯源树,研究论文发展脉络
Chat Paper
正在生成论文摘要