Implicit Trust and Distrust Prediction for Recommender Systems.
WISE(2015)
Abstract
Many trust-aware recommender systems TARSs have explored the value of explicit trust which is specified by users with binary values. But existing works of TARSs suffer from the problem of the lack of explicit trust which may not always be available in online social networks. In order to solve this issue, some implicit trust based TARSs are proposed. However, these methods generally predict implicit trust scores between users based on the interpersonal aspect, i.e., propagated trust, similarity obtained by user ratings on co-rated items, while ignore the personal aspects, i.e., trust bias and the impersonal aspects, i.e., local-topology-based features in trust networks, which are also important for implicit trust prediction. In this paper, we attempt to propose a classification approach to address the trust/distrust prediction problem. First, we obtain an extensive set of relevant features derived from the personal aspects, interpersonal aspects and impersonal aspects of trust. Then a logistic regression model is developed and trained by accommodating these factors, and applied to predict continuous values of users' trust and distrust. We conduct an empirical study to evaluate the accuracy of the predicted implicit trust. The experimental results on real-world data sets demonstrate the effectiveness of our proposed model in employing implicit trust/distrust into existing trust-aware recommendation approaches.
MoreTranslated text
Key words
Implicit trust/distrust, Logistic regression, Recommender systems
AI Read Science
Must-Reading Tree
Example
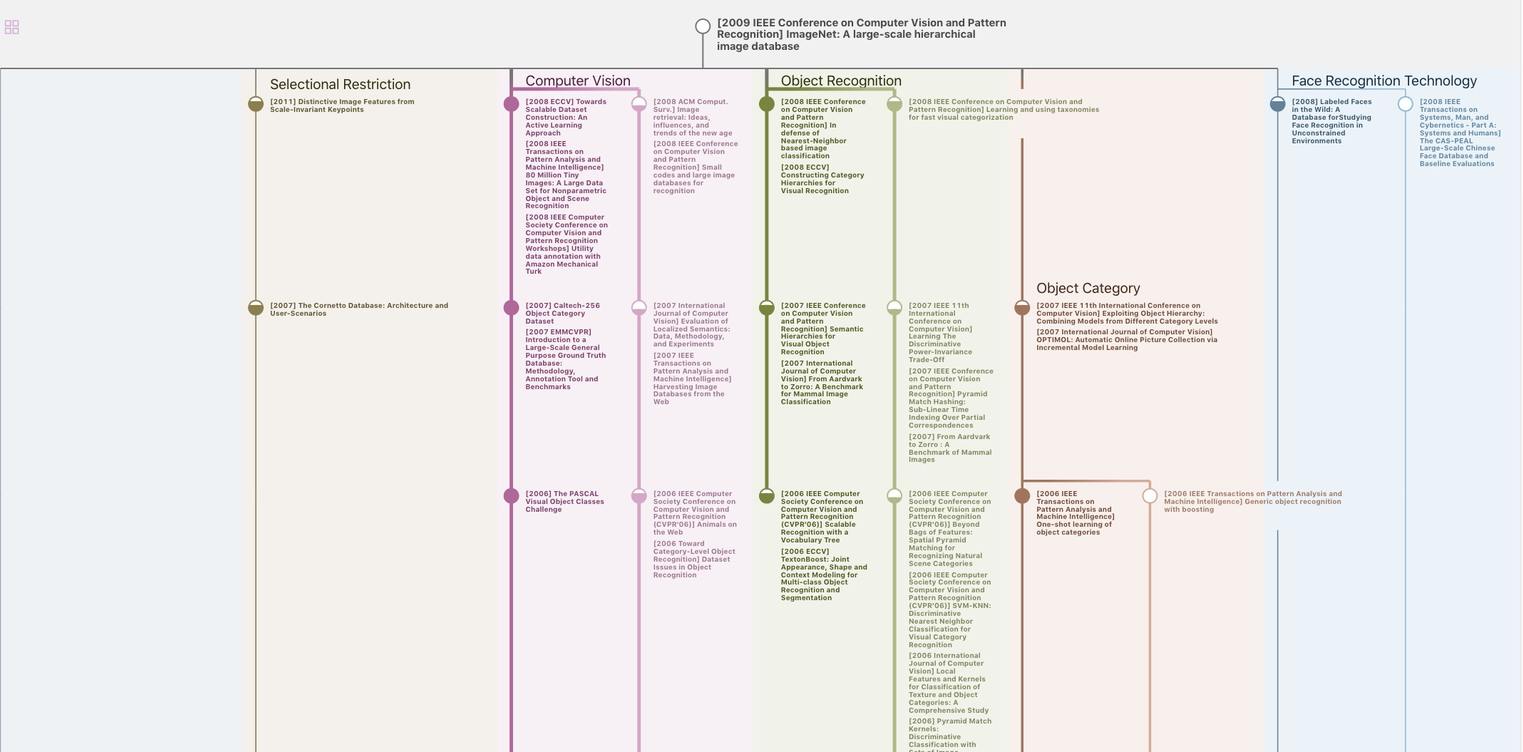
Generate MRT to find the research sequence of this paper
Chat Paper
Summary is being generated by the instructions you defined