A Memory Capacity Model For High Performing Data-Filtering Applications In Samza Framework
2015 IEEE International Conference on Big Data (Big Data)(2015)
摘要
Data quality is essential in big data paradigm as poor data can have serious consequences when dealing with large volumes of data. While it is trivial to spot poor data for small-scale and offline use cases, it is challenging to detect and fix data inconsistency in large-scale and online (real-time or near-real time) big data context. An example of such scenario is spotting and fixing poor data using Apache Samza, a stream processing framework that has been increasingly adopted to process near-real-time data at LinkedIn.To optimize the deployment of Samza processing and reduce business cost, in this work we propose a memory capacity model for Apache Samza to allow denser deployments of high performing data-filtering applications built on Samza. The model can be used to provision just-enough memory resource to applications by tightening the bounds on the memory allocations. We apply our memory capacity model on LinkedIn's real use cases in production, which significantly increases the deployment density and saves business costs. We will share key learning in this paper.
更多查看译文
关键词
Apache Samza,capacity model,data filtering,performance
AI 理解论文
溯源树
样例
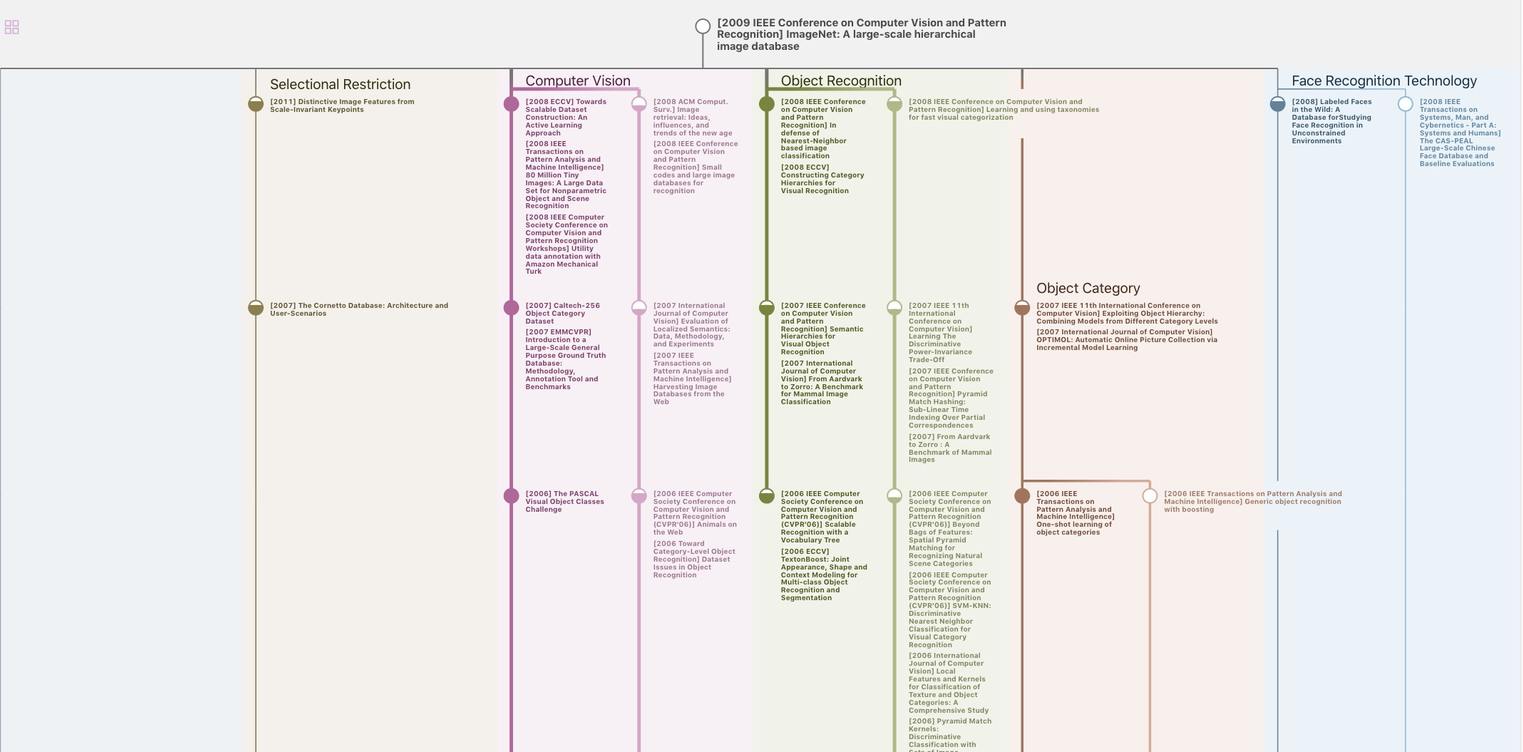
生成溯源树,研究论文发展脉络
Chat Paper
正在生成论文摘要