Reinforcement Learning from Demonstration through Shaping.
IJCAI(2015)
摘要
Reinforcement learning describes how a learning agent can achieve optimal behaviour based on interactions with its environment and reward feedback. A limiting factor in reinforcement learning as employed in artificial intelligence is the need for an often prohibitively large number of environment samples before the agent reaches a desirable level of performance. Learning from demonstration is an approach that provides the agent with demonstrations by a supposed expert, from which it should derive suitable behaviour. Yet, one of the challenges of learning from demonstration is that no guarantees can be provided for the quality of the demonstrations, and thus the learned behavior. In this paper, we investigate the intersection of these two approaches, leveraging the theoretical guarantees provided by reinforcement learning, and using expert demonstrations to speed up this learning by biasing exploration through a process called reward shaping. This approach allows us to leverage human input without making an erroneous assumption regarding demonstration optimality. We show experimentally that this approach requires significantly fewer demonstrations, is more robust against suboptimality of demonstrations, and achieves much faster learning than the recently developed HAT algorithm.
更多查看译文
AI 理解论文
溯源树
样例
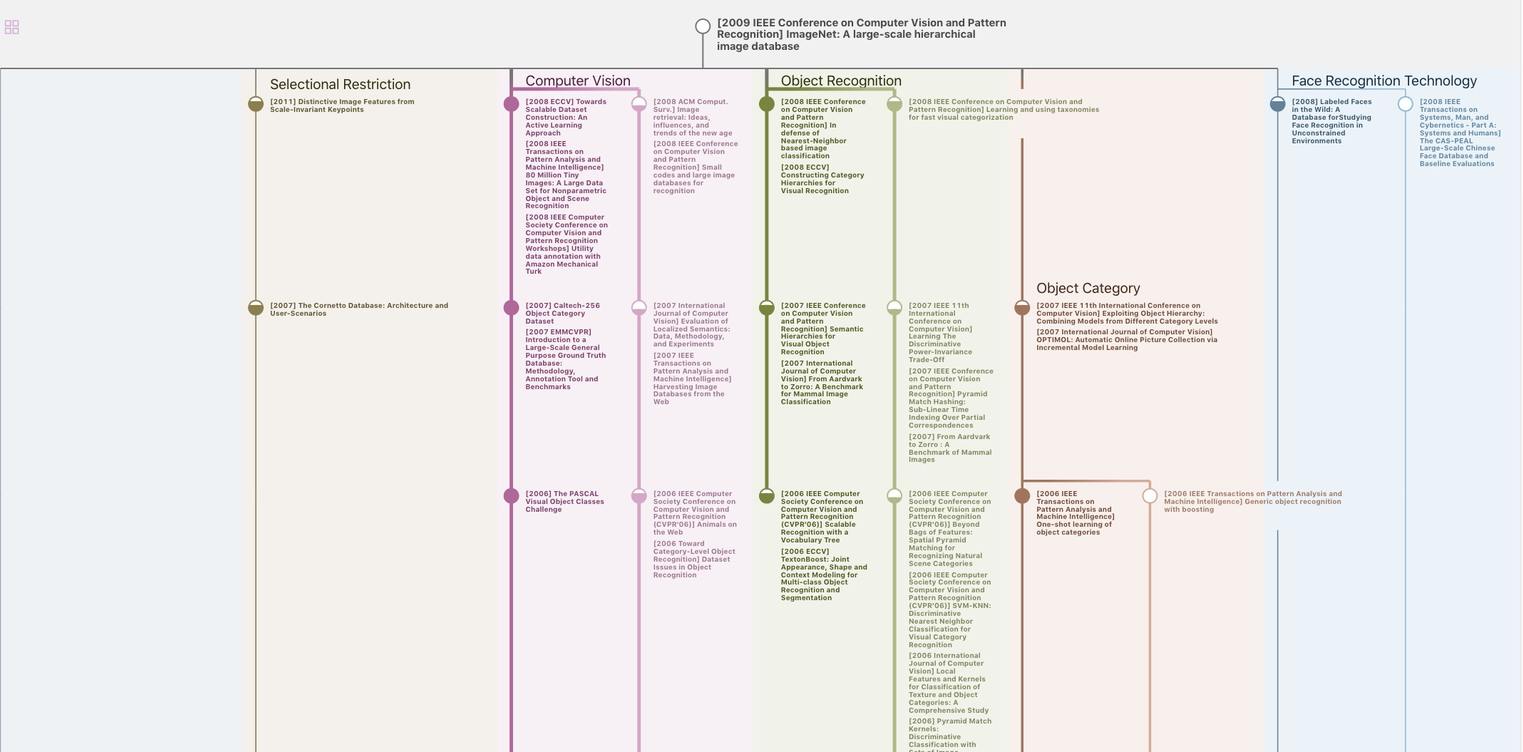
生成溯源树,研究论文发展脉络
Chat Paper
正在生成论文摘要