A Comparison Of Neural Network Methods For Unsupervised Representation Learning On The Zero Resource Speech Challenge
16TH ANNUAL CONFERENCE OF THE INTERNATIONAL SPEECH COMMUNICATION ASSOCIATION (INTERSPEECH 2015), VOLS 1-5(2015)
摘要
The success of supervised deer neural networks (DNNs) in speech recognition cannot be transferred to zero-resource languages where the requisite transcriptions arc unavailable. We investigate unsupervised neural network based methods for learning frame-level representations. Good frame representations eliminate differences in accent, gender, channel characteristics, and other factors to model subword units for within- and across speaker phonetic discrimination. We enhance the correspondence autoencoder (cAE) and show that it can transform Mel Frequency Cepstral Coefficients (MFCCs) into more effective frame representations given a set of matched word pairs from an unsupervised term discovery (UTD) system. The cAE combines the feature extraction power of autoencoders with the weak supervision signal from MD pairs to better approximate the extrinsic task's objective during training. We use the Zero Resource Speech Challenge's minimal triphone pair ABX discrimination task to evaluate our methods. Optimizing a cAE architecture on English and applying it to a zero-resource language, Xitsonga, we obtain a relative error rate reduction of 35% compared to the original MFCCs. We also show that Xitsonga frame representations extracted from the bottleneck layer of a supervised DNN trained on English can be further enhanced by the cAE, yielding a relative error rate reduction of 39%.
更多查看译文
关键词
unsupervised speech processing, representation learning, zero-resources, neural networks, autoencoders
AI 理解论文
溯源树
样例
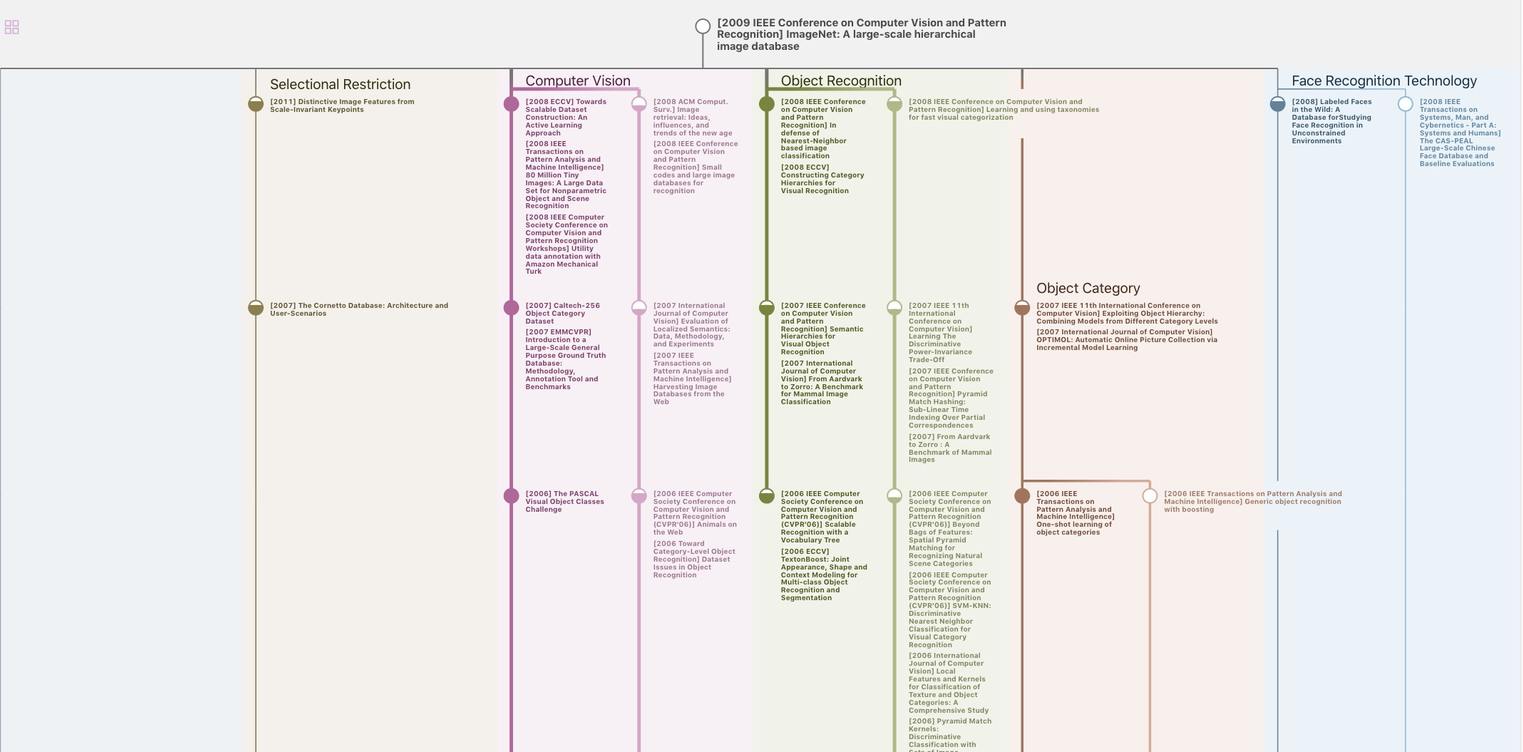
生成溯源树,研究论文发展脉络
Chat Paper
正在生成论文摘要