Distinct Triphone Acoustic Modeling Using Deep Neural Networks.
Interspeech(2015)
Key words
distinct triphone acoustic modeling,multi-task learning,deep neural networks
AI Read Science
Must-Reading Tree
Example
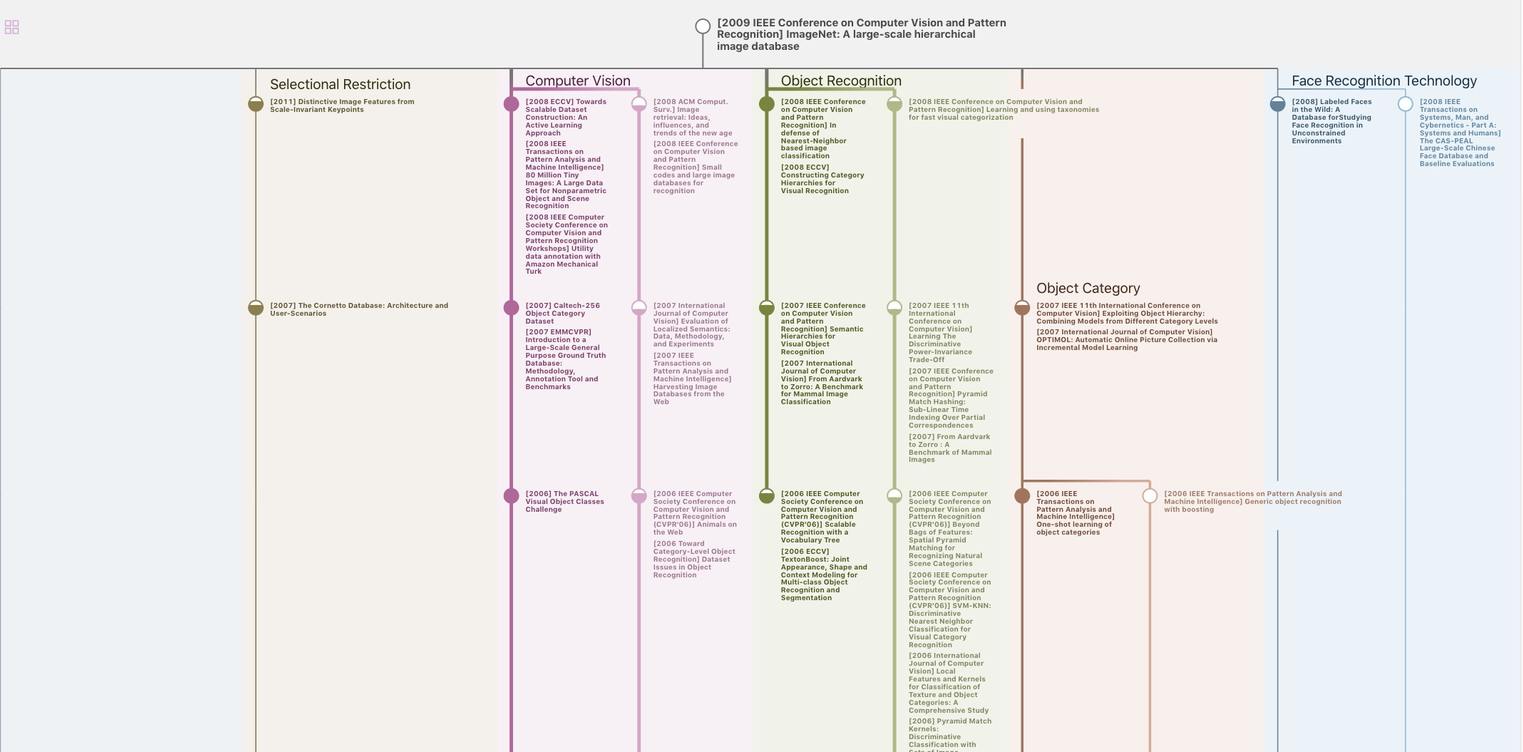
Generate MRT to find the research sequence of this paper
Chat Paper
Summary is being generated by the instructions you defined