A Hybrid Dynamic Time Warping-Deep Neural Network Architecture For Unsupervised Acoustic Modeling
16TH ANNUAL CONFERENCE OF THE INTERNATIONAL SPEECH COMMUNICATION ASSOCIATION (INTERSPEECH 2015), VOLS 1-5(2015)
Abstract
We report on an architecture for the unsupervised discovery of talker-invariant subword embeddings. It is made out of two components: a dynamic-time warping based spoken term discovery (STD) system and a Siamese deep neural network (DNN). The STD system clusters word-sized repeated fragments in the acoustic streams while the DNN is trained to minimize the distance between time aligned frames of tokens of the same cluster, and maximize the distance between tokens of different clusters. We use additional side information regarding the average duration of phonemic units, as well as talker identity tags. For evaluation we use the datasets and metrics of the Zero Resource Speech Challenge. The model shows improvement over the baseline in subword unit modeling.
MoreTranslated text
Key words
zero resource speech challenge, feature extraction, deep learning
AI Read Science
Must-Reading Tree
Example
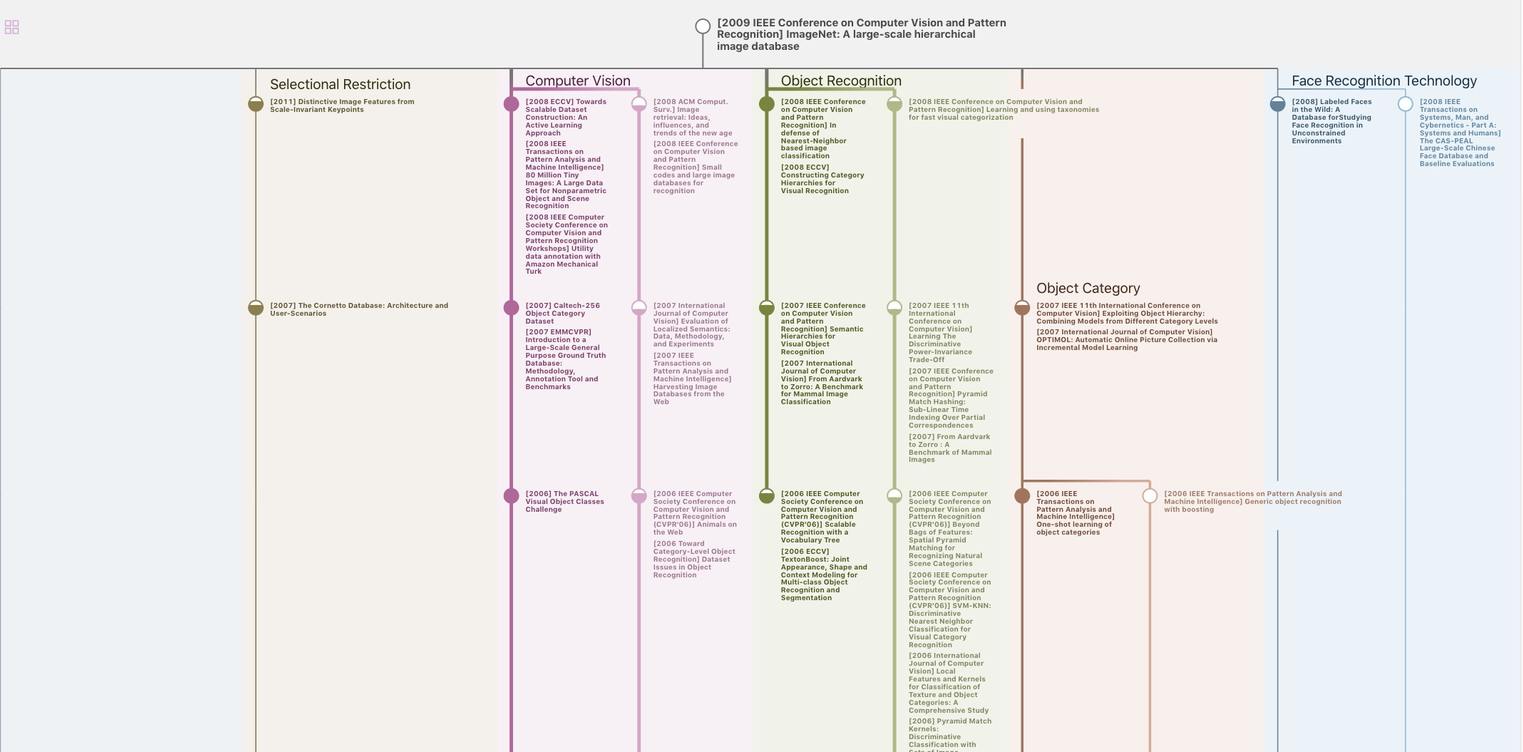
Generate MRT to find the research sequence of this paper
Chat Paper
Summary is being generated by the instructions you defined