Learning Spatiotemporal Features with 3D Convolutional Networks
2015 IEEE International Conference on Computer Vision (ICCV)(2015)
Abstract
We propose a simple, yet effective approach for spatiotemporal feature learning using deep 3-dimensional convolutional networks (3D ConvNets) trained on a large scale supervised video dataset. Our findings are three-fold: 1) 3D ConvNets are more suitable for spatiotemporal feature learning compared to 2D ConvNets; 2) A homogeneous architecture with small 3x3x3 convolution kernels in all layers is among the best performing architectures for 3D ConvNets; and 3) Our learned features, namely C3D (Convolutional 3D), with a simple linear classifier outperform state-of-the-art methods on 4 different benchmarks and are comparable with current best methods on the other 2 benchmarks. In addition, the features are compact: achieving 52.8% accuracy on UCF101 dataset with only 10 dimensions and also very efficient to compute due to the fast inference of ConvNets. Finally, they are conceptually very simple and easy to train and use.
MoreTranslated text
Key words
spatiotemporal feature learning,3D convolutional networks,deep 3-dimensional convolutional networks,3D ConvNets,large scale supervised video dataset,homogeneous architecture,convolution kernels,C3D features,convolutional 3D,linear classifier,UCF101 dataset
AI Read Science
Must-Reading Tree
Example
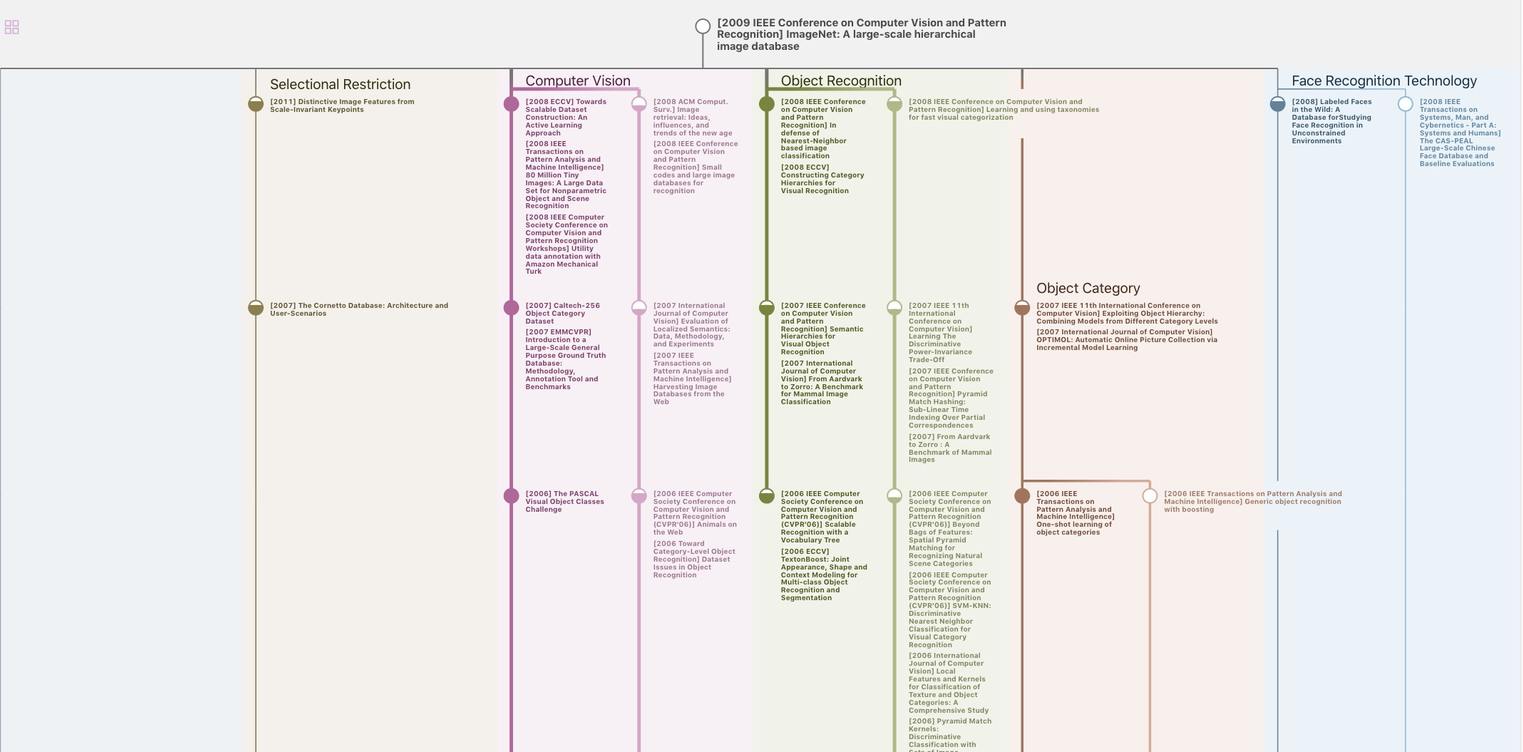
Generate MRT to find the research sequence of this paper
Chat Paper
Summary is being generated by the instructions you defined