FlowNet: Learning Optical Flow with Convolutional Networks.
ICCV(2015)
摘要
Convolutional neural networks (CNNs) have recently been very successful in a variety of computer vision tasks, especially on those linked to recognition. Optical flow estimation has not been among the tasks CNNs succeeded at. In this paper we construct CNNs which are capable of solving the optical flow estimation problem as a supervised learning task. We propose and compare two architectures: a generic architecture and another one including a layer that correlates feature vectors at different image locations. Since existing ground truth data sets are not sufficiently large to train a CNN, we generate a large synthetic Flying Chairs dataset. We show that networks trained on this unrealistic data still generalize very well to existing datasets such as Sintel and KITTI, achieving competitive accuracy at frame rates of 5 to 10 fps.
更多查看译文
关键词
FlowNet,optical flow learning,convolutional neural network,CNN,computer vision,opticalflow estimation problem,supervised learning,generic architecture,ground truth data,synthetic flying chair dataset
AI 理解论文
溯源树
样例
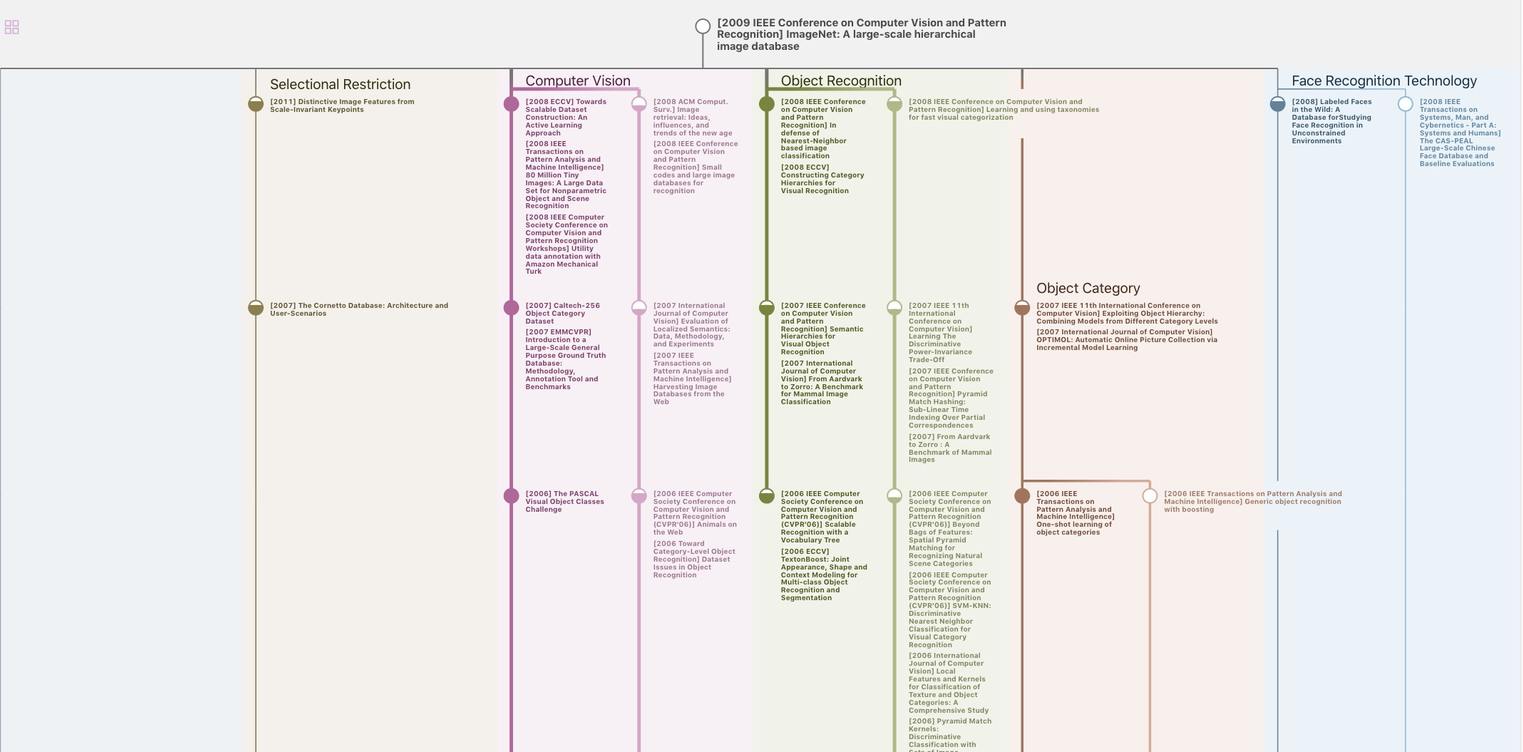
生成溯源树,研究论文发展脉络
Chat Paper
正在生成论文摘要