Learning To Track: Online Multi-Object Tracking By Decision Making
2015 IEEE International Conference on Computer Vision (ICCV)(2015)
摘要
Online Multi-Object Tracking (MOT) has wide applications in time-critical video analysis scenarios, such as robot navigation and autonomous driving. In tracking-by-detection, a major challenge of online MOT is how to robustly associate noisy object detections on a new video frame with previously tracked objects. In this work, we formulate the online MOT problem as decision making in Markov Decision Processes (MDPs), where the lifetime of an object is modeled with a MDP. Learning a similarity function for data association is equivalent to learning a policy for the MDP, and the policy learning is approached in a reinforcement learning fashion which benefits from both advantages of offline-learning and online-learning for data association. Moreover, our framework can naturally handle the birth/death and appearance/disappearance of targets by treating them as state transitions in the MDP while leveraging existing online single object tracking methods. We conduct experiments on the MOT Benchmark [24] to verify the effectiveness of our method.
更多查看译文
关键词
learning-to-track,online multiobject tracking,decision making,time-critical video analysis scenarios,robot navigation,autonomous driving,tracking-by-detection,video frame,noisy object detections,online MOT problem,Markov decision processes,MDP,data association,policy learning,reinforcement learning fashion,birth handling,death handling,target appearance handling,target disappearance handling,MOT Benchmark,similarity function,offline-learning
AI 理解论文
溯源树
样例
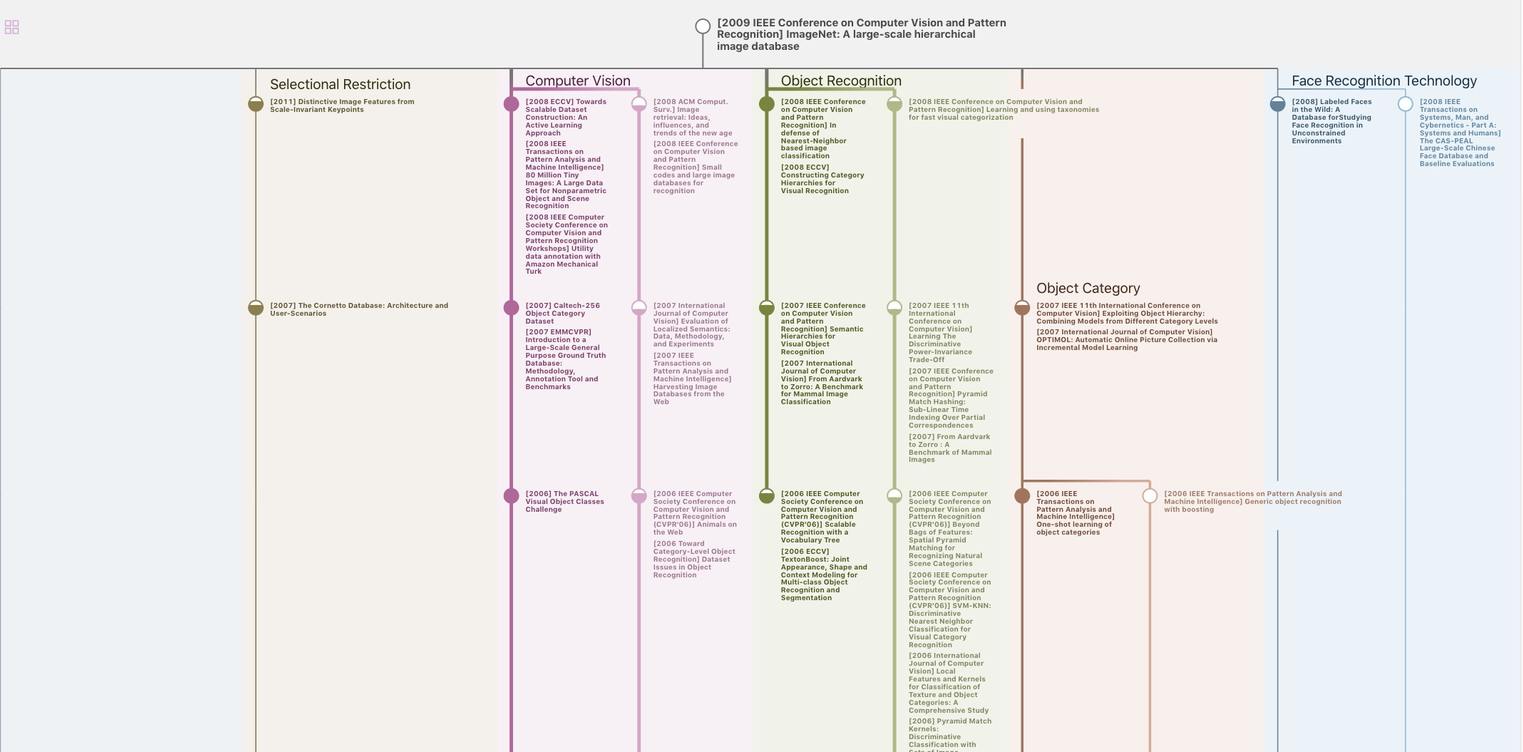
生成溯源树,研究论文发展脉络
Chat Paper
正在生成论文摘要