Refinement of Probabilistic Stabilizing Programs Using Genetic Algorithms.
SSS(2015)
摘要
In this paper, we evaluate the role of genetic algorithms GAs for identifying optimal probabilities in probabilistic self-stabilizing algorithms. Although it is known that the use of probabilistic actions is beneficial for reducing the state space requirements and solving problems that are unsolvable in the deterministic manner, identifying the﾿ideal probabilities is often difficult. This is especially the case where several independent probability values need to be selected. We analyze two token ring protocols proposed by Herman ---an asymmetric program where one process is distinguished and a symmetric program where all processes are identical anonymous. We find that for the asymmetric program, unequal probabilities are preferred for smaller rings. Moreover, the size of the ring for which equal probability is desirable increases with the increase in the states available to individual processes. By contrast, unequal probabilities are preferred for the symmetric token ring when the number of processes increases. We also consider the case where the symmetric protocol is modified to allow each process to choose the probabilities independently. We find that making a few processes almost deterministic reduces the expected convergence time. Finally, we note that the analysis in the paper can also be used to identify the increased cost of randomization when compared to a corresponding deterministic solution.
更多查看译文
关键词
Genetic Algorithm, Convergence Time, Optimal Probability, Ring Size, Nondominated Solution
AI 理解论文
溯源树
样例
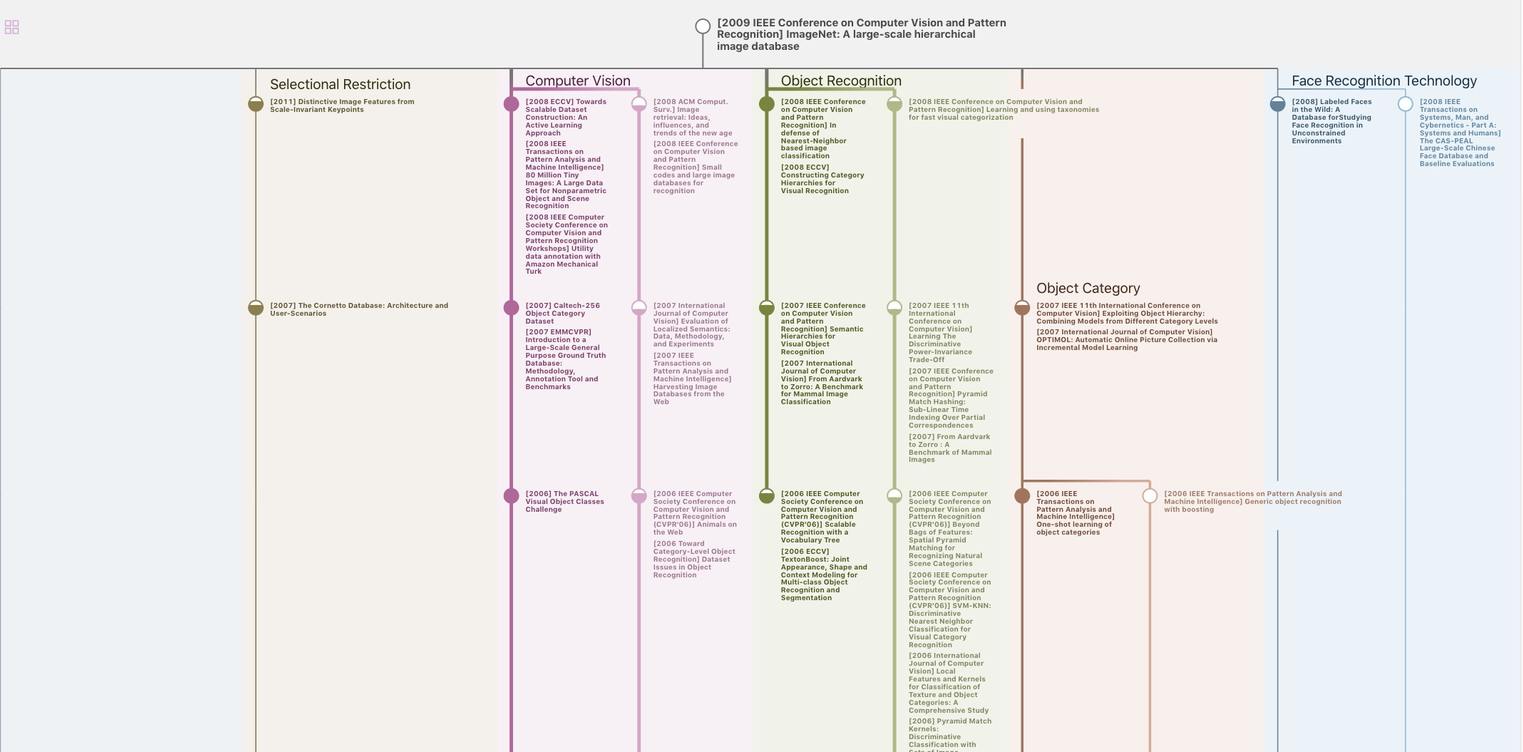
生成溯源树,研究论文发展脉络
Chat Paper
正在生成论文摘要