Learning Continuous Word Embedding With Metadata For Question Retrieval In Community Question Answering
PROCEEDINGS OF THE 53RD ANNUAL MEETING OF THE ASSOCIATION FOR COMPUTATIONAL LINGUISTICS AND THE 7TH INTERNATIONAL JOINT CONFERENCE ON NATURAL LANGUAGE PROCESSING, VOL 1(2015)
摘要
Community question answering (cQA) has become an important issue due to the popularity of cQA archives on the web. This paper is concerned with the problem of question retrieval. Question retrieval in cQA archives aims to find the existing questions that are semantically equivalent or relevant to the queried questions. However, the lexical gap problem brings about new challenge for question retrieval in cQA. In this paper, we propose to learn continuous word embeddings with metadata of category information within cQA pages for question retrieval. To deal with the variable size of word embedding vectors, we employ the framework of fisher kernel to aggregated them into the fixed-length vectors. Experimental results on large-scale real world cQA data set show that our approach can significantly outperform state-of-the-art translation models and topic-based models for question retrieval in cQA.
更多查看译文
AI 理解论文
溯源树
样例
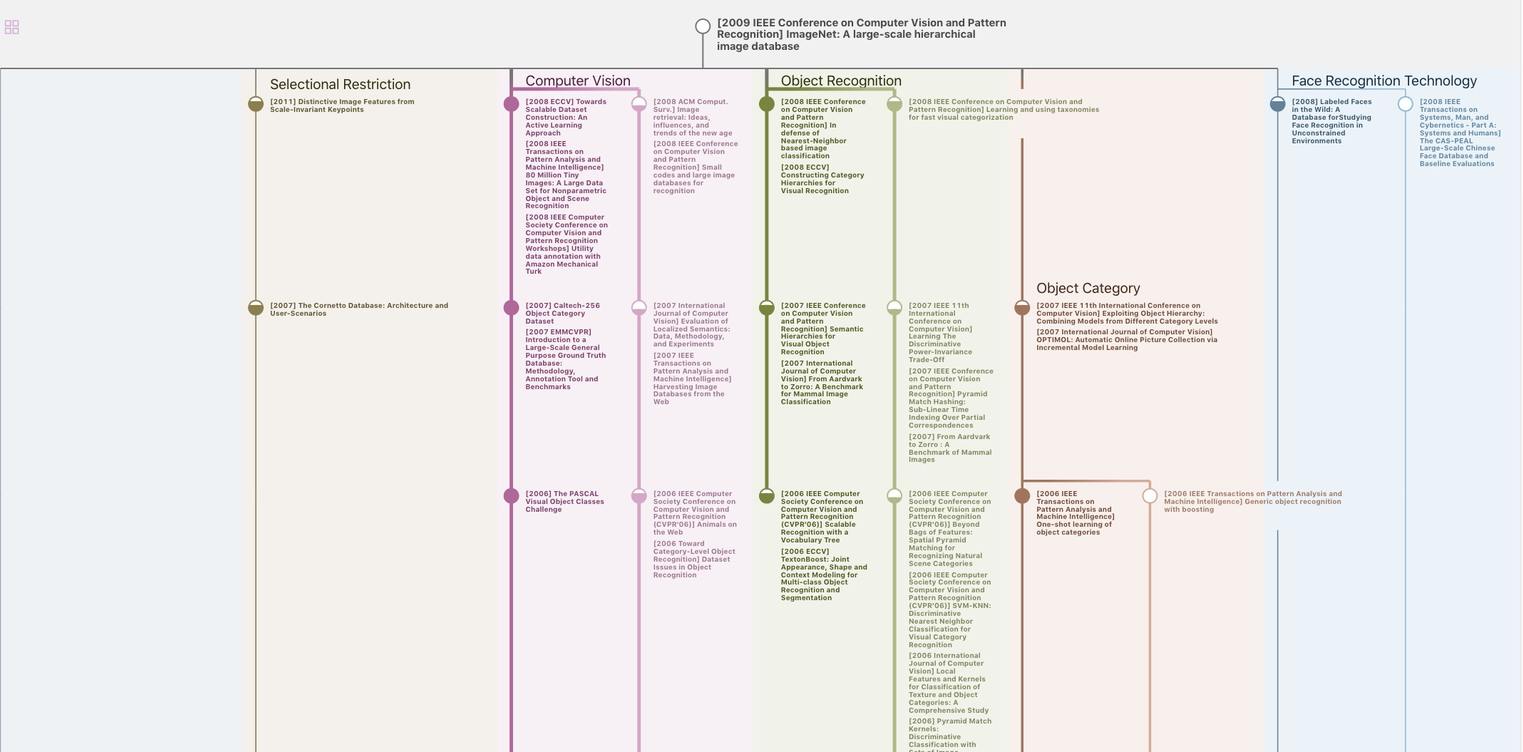
生成溯源树,研究论文发展脉络
Chat Paper
正在生成论文摘要