Zero-Shot Image Tagging By Hierarchical Semantic Embedding
IR(2015)
摘要
Given the difficulty of acquiring labeled examples for many fine-grained visual classes, there is an increasing interest in zero-shot image tagging, aiming to tag images with novel labels that have no training examples present. Using a semantic space trained by a neural language model, the current state-of-the-art embeds both images and labels into the space, wherein cross-media similarity is computed. However, for labels of relatively low occurrence, its similarity to images and other labels can be unreliable. This paper proposes Hierarchical Semantic Embedding (HierSE), a simple model that exploits the WordNet hierarchy to improve label embedding and consequently image embedding. Moreover, we identify two good tricks, namely training the neural language model using Flickr lags instead of web documents, and using partial match instead of full match for vectorizing a WordNet node. All this lets us outperform the state-of-the-art. OH a test set of over 1,500 visual object classes and 1.3 million images, the proposed model beats the current best results (18.3% versus 9.4% in hit@1).
更多查看译文
关键词
Image tagging,zero shot learning,semantic embedding
AI 理解论文
溯源树
样例
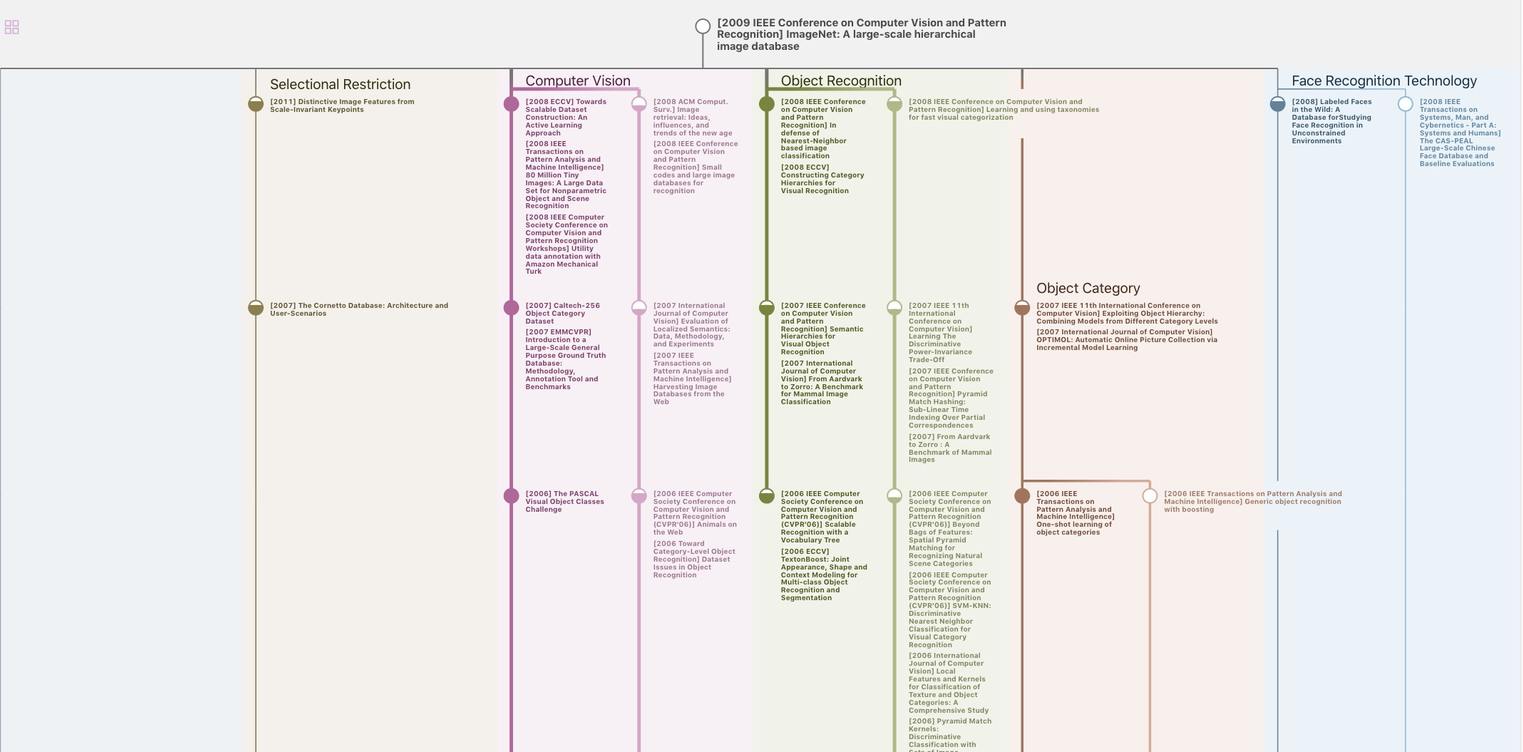
生成溯源树,研究论文发展脉络
Chat Paper
正在生成论文摘要