Shapely Value Based Random Subspace Selection for Hyperspectral Image Classification
IEEE International Geoscience and Remote Sensing Symposium(2015)
Abstract
In this paper, an algorithm to randomly select feature sub-spaces for hyperspectral image classification using the principle of coalition game theory is presented. The feature selection algorithms associated with non-linear kernel based Support Vector Machines (SVM) are either NP-hard or greedy and hence, not very optimal. To deal with this problem, a metric based on the principles of coalition game theory called Shapely value and a sampling approximation is used to determine the contributions of individual features towards the classification task. Feature subsets are randomly drawn from a probability distribution function generated using normalized Shapely values of the individual features. These feature subsets are then used to build kernels corresponding to individual weak classifiers in the Sparse Kernel-based Ensemble Learning (SKEL) framework. By weighting the kernels optimally and sparsely, a small number of useful subsets of features are selected which improve the generalization performance of the ensemble classifier. The algorithm is applied on real hyper-spectral datasets and the results are presented in the paper.
MoreTranslated text
Key words
coalition game theory,sampling approximation,probability distribution function,normalized Shapely values,sparse kernel-based ensemble learning,SKEL framework,feature subset selection,ensemble classifier,random subspace selection,hyperspectral image classification
AI Read Science
Must-Reading Tree
Example
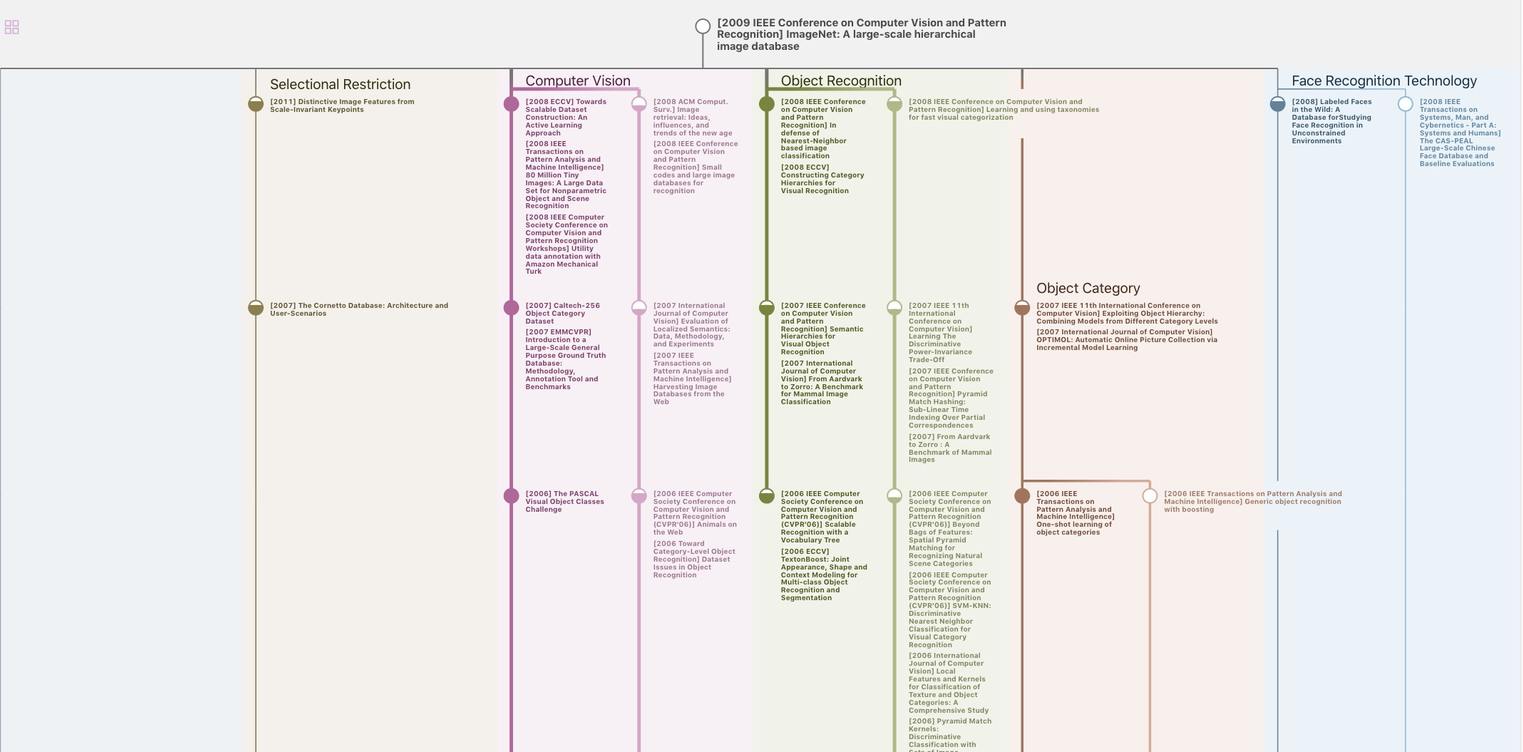
Generate MRT to find the research sequence of this paper
Chat Paper
Summary is being generated by the instructions you defined