Reconstruction Of Time-Series Soil Moisture From Amsr2 And Smos Data By Using Recurrent Nonlinear Autoregressive Neural Networks
2015 IEEE International Geoscience and Remote Sensing Symposium (IGARSS)(2015)
摘要
Soil moisture (SM) is a key variable in describing land surface characteristics. However, most passive microwave sensed soil moisture products are spatially and temporally discontinuous. In this study, a recurrent autoregressive neural network was investigated for its capability to reconstruct time-series soil moisture. The train dataset was collected from the observations of AMSR2 and SMOS, along with the daily NDVI, land surface temperature (LST), precipitation (PRC) and DEM information. Then, the trained neural network was used to predict time-series soil moisture at a spatial resolution of 0.25 degrees. Result shows that this approach is promising in providing time-series soil moisture. Moreover, compared to ground soil moisture measurements, the predicted dataset tends to have lower root-mean-square error (rmse) and higher correlation coefficient (R) than the original soil moisture product of AMSR2 and SMOS.
更多查看译文
关键词
soil moisture,neural networks,passive microwave,AMSR2,SMOS
AI 理解论文
溯源树
样例
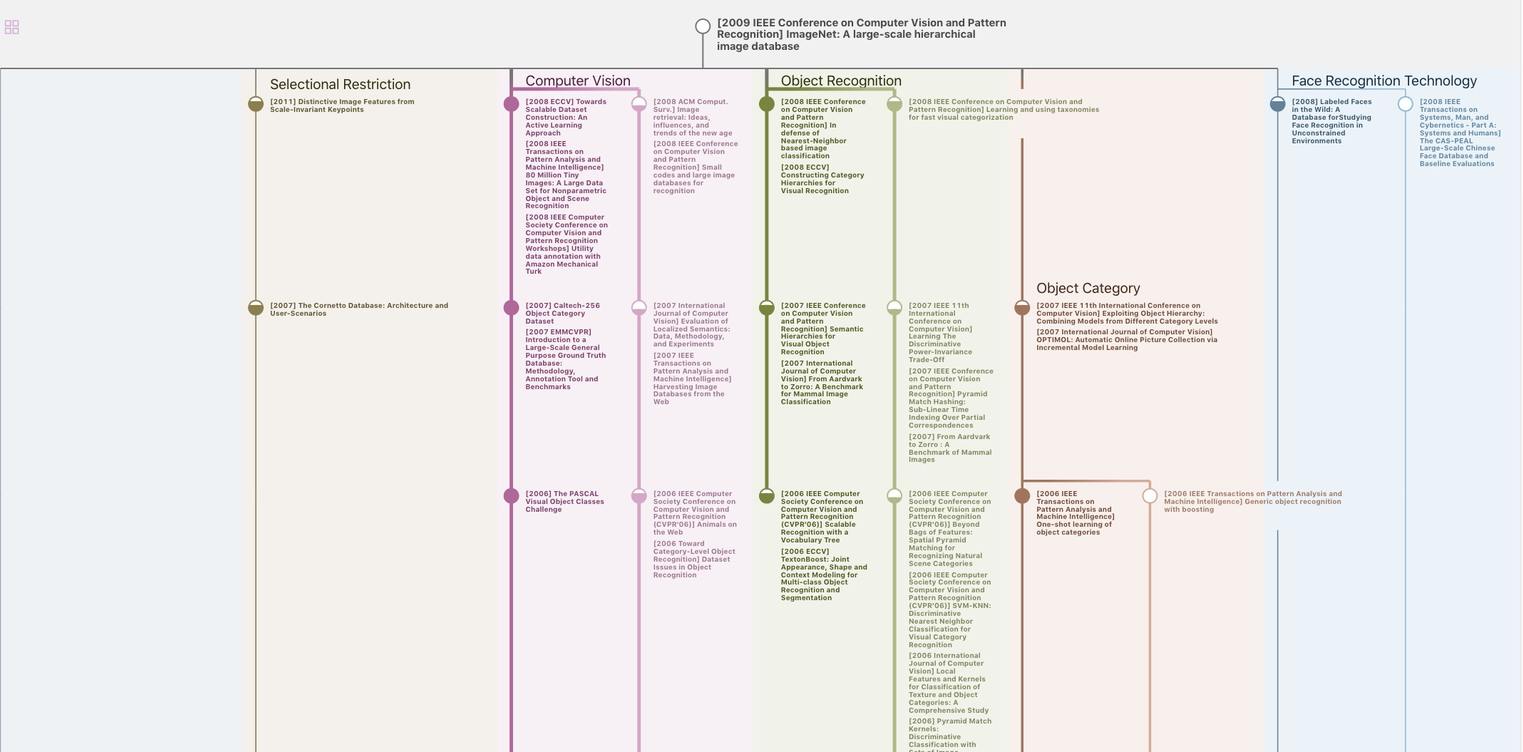
生成溯源树,研究论文发展脉络
Chat Paper
正在生成论文摘要