Probabilistic combination of classification rules and its application to medical diagnosis
Machine Learning(2015)
摘要
Application of machine learning to medical diagnosis entails facing two major issues, namely, a necessity of learning comprehensible models and a need of coping with imbalanced data phenomenon. The first one corresponds to a problem of implementing interpretable models, e.g., classification rules or decision trees. The second issue represents a situation in which the number of examples from one class (e.g., healthy patients) is significantly higher than the number of examples from the other class (e.g., ill patients). Learning algorithms which are prone to the imbalance data return biased models towards the majority class. In this paper, we propose a probabilistic combination of soft rules , which can be seen as a probabilistic version of the classification rules, by introducing new latent random variable called conjunctive feature . The conjunctive features represent conjunctions of values of attribute variables (features) and we assume that for given conjunctive feature the object and its label (class) become independent random variables. In order to deal with the between class imbalance problem, we present a new estimator which incorporates the knowledge about data imbalanceness into hyperparameters of initial probability of objects with fixed class labels. Additionally, we propose a method for aggregating sufficient statistics needed to estimate probabilities in a graph-based structure to speed up computations. At the end, we carry out two experiments: (1) using benchmark datasets, (2) using medical datasets. The results are discussed and the conclusions are drawn.
更多查看译文
关键词
Probabilistic combination,Classification rules,Imbalanced data,Medical diagnosis
AI 理解论文
溯源树
样例
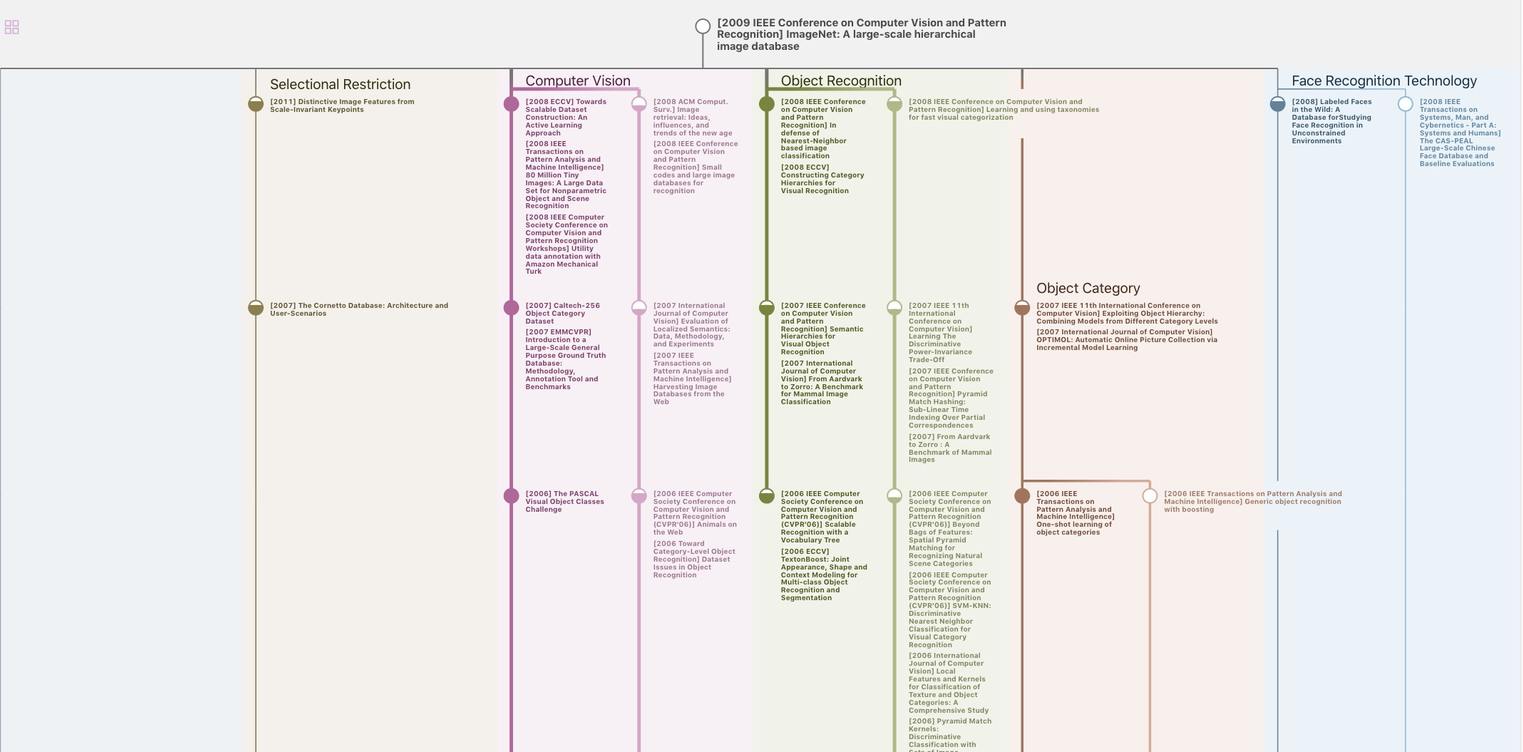
生成溯源树,研究论文发展脉络
Chat Paper
正在生成论文摘要