KNET: A General Framework for Learning Word Embedding Using Morphological Knowledge
ACM Trans. Inf. Syst.(2015)
摘要
Neural network techniques are widely applied to obtain high-quality distributed representations of words (i.e., word embeddings) to address text mining, information retrieval, and natural language processing tasks. Most recent efforts have proposed several efficient methods to learn word embeddings from context such that they can encode both semantic and syntactic relationships between words. However, it is quite challenging to handle unseen or rare words with insufficient context. Inspired by the study on the word recognition process in cognitive psychology, in this article, we propose to take advantage of seemingly less obvious but essentially important morphological knowledge to address these challenges. In particular, we introduce a novel neural network architecture called KNET that leverages both words’ contextual information and morphological knowledge to learn word embeddings. Meanwhile, this new learning architecture is also able to benefit from noisy knowledge and balance between contextual information and morphological knowledge. Experiments on an analogical reasoning task and a word similarity task both demonstrate that the proposed KNET framework can greatly enhance the effectiveness of word embeddings.
更多查看译文
关键词
Neural network,word embedding,morphological knowledge
AI 理解论文
溯源树
样例
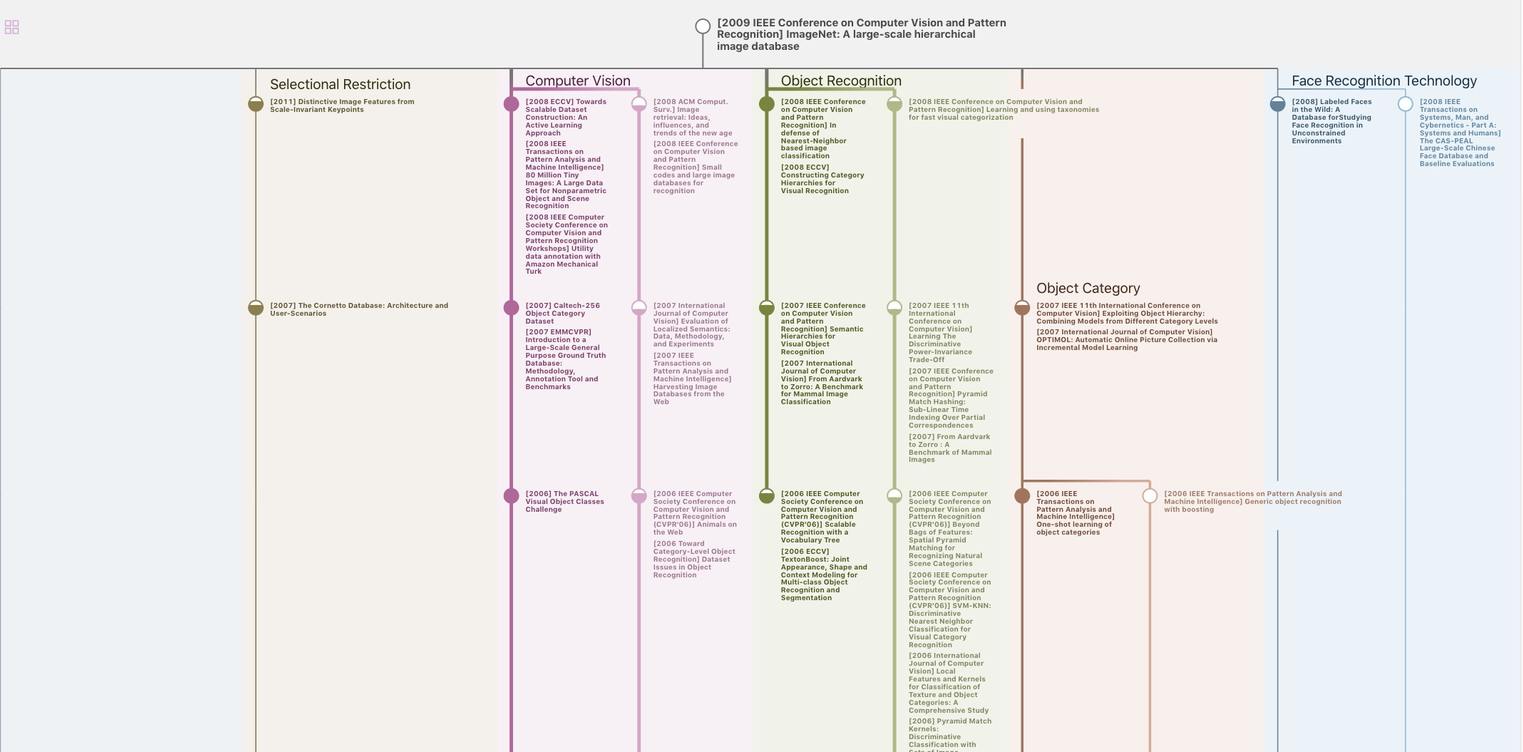
生成溯源树,研究论文发展脉络
Chat Paper
正在生成论文摘要