Resource Constrained Data Stream Clustering With Concept Drifting For Processing Sensor Data
International Journal of Data Warehousing and Mining(2015)
摘要
Wireless sensors and mobile devices have been widely deployed as data collecting devices for monitoring real world systems. A large amount of stream data is generated in real-time, which has to be processed in real-time as well. One of the common processing operations is clustering that automatically groups the elements of a stream into a number of clusters in general. Elements of the same cluster have maximum similarity and elements of different clusters have minimum similarity. This paper proposes an on-demand framework (SRAStream) based on the concept drifting detection mechanism. The concept drifting detection algorithm is used to measure the distance of the new clusters for the current data and that of the existing clusters. Only when a concept drifting occurs will the re-clustering be performed to identify new clusters. SRAStream thus avoids the unnecessary computation intensive re-clustering calculation. Experiments suggest that the proposed framework does work well and improve the processing speed greatly in data streams clustering.
更多查看译文
关键词
Concept Drifting,Data Mining,Stream Data
AI 理解论文
溯源树
样例
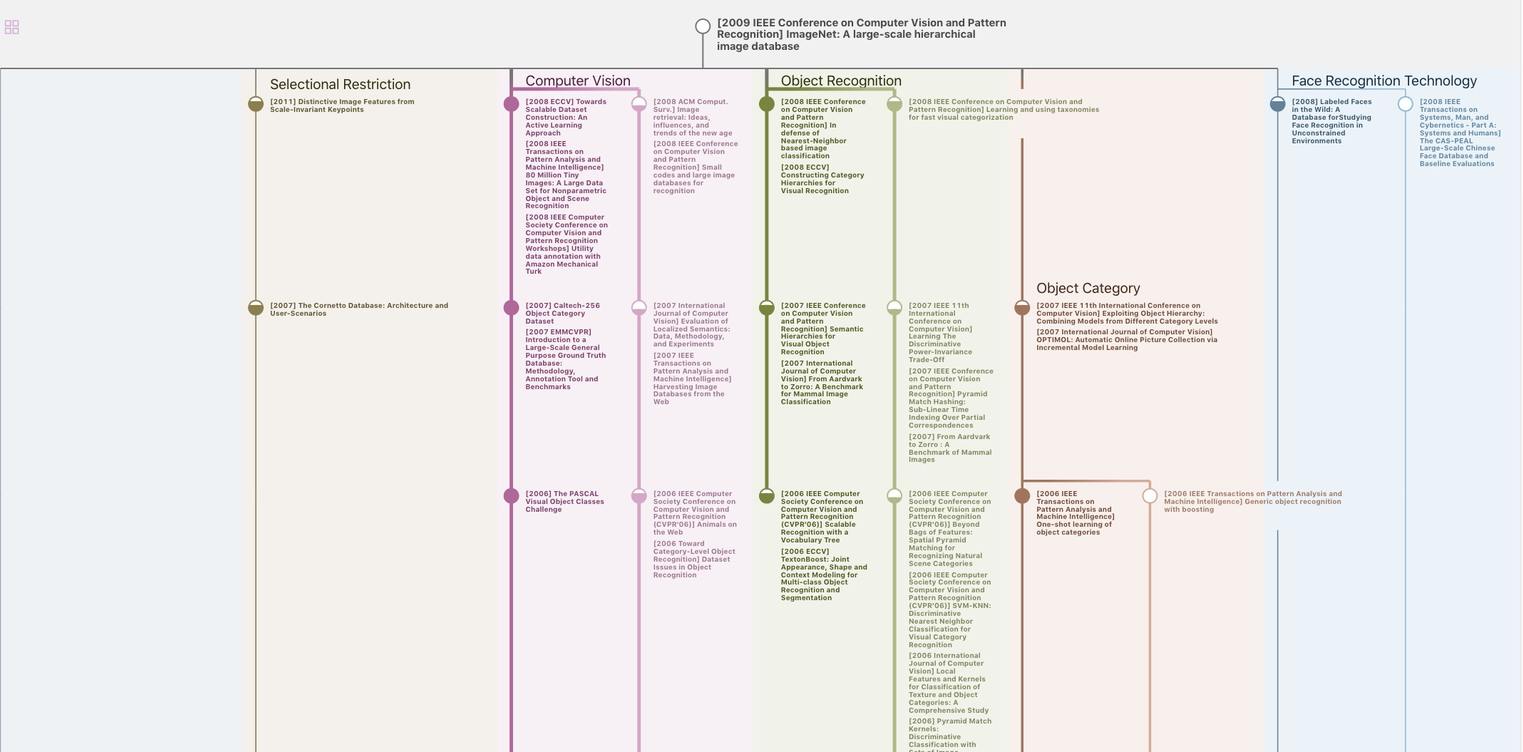
生成溯源树,研究论文发展脉络
Chat Paper
正在生成论文摘要