Probabilistic Self-Organizing Map For Clustering And Visualizing Non-I.I.D Data
INTERNATIONAL JOURNAL OF COMPUTATIONAL INTELLIGENCE AND APPLICATIONS(2015)
摘要
We present a generative approach to train a new probabilistic self-organizing map (PrSOMS) for dependent and nonidentically distributed data sets. Our model defines a low-dimensional manifold allowing friendly visualizations. To yield the topology preserving maps, our model has the SOM like learning behavior with the advantages of probabilistic models. This new paradigm uses hidden Markov models (HMM) formalism and introduces relationships between the states. This allows us to take advantage of all the known classical views associated to topographic map. The objective function optimization has a clear interpretation, which allows us to propose expectation-maximization (EM) algorithm, based on the forward-backward algorithm, to train the model. We demonstrate our approach on two data sets: The real-world data issued from the "French National Audiovisual Institute" and handwriting data captured using a WACOM tablet.
更多查看译文
关键词
Mixture model, self-organizing map, hidden Markov model, visualization, EM algorithm, non-i.i.d data
AI 理解论文
溯源树
样例
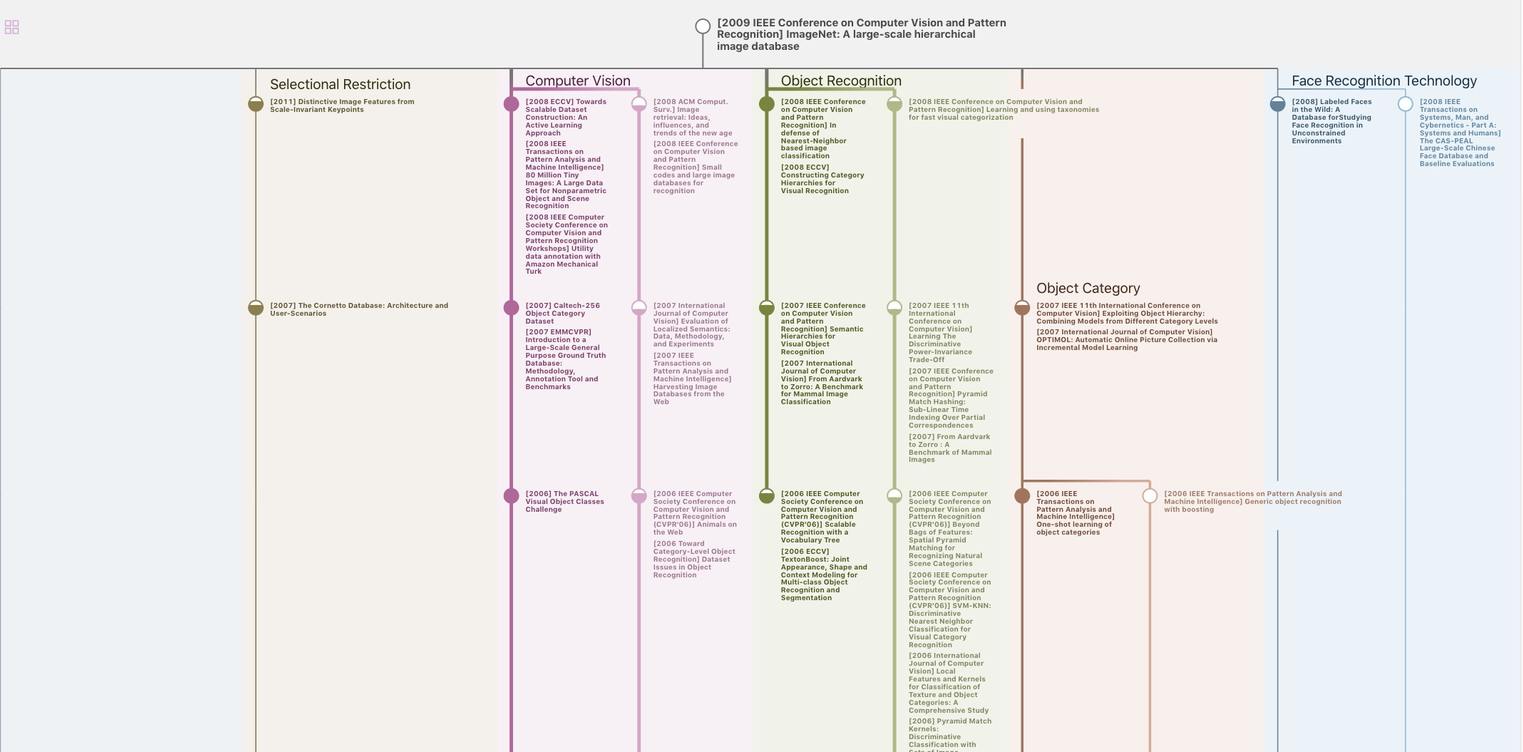
生成溯源树,研究论文发展脉络
Chat Paper
正在生成论文摘要