Analyzing simulation-based PRA data through traditional and topological clustering: A BWR station blackout case study
Reliability Engineering & System Safety(2016)
摘要
Dynamic probabilistic risk assessment (DPRA) methodologies couple system simulator codes (e.g., RELAP and MELCOR) with simulation controller codes (e.g., RAVEN and ADAPT). Whereas system simulator codes model system dynamics deterministically, simulation controller codes introduce both deterministic (e.g., system control logic and operating procedures) and stochastic (e.g., component failures and parameter uncertainties) elements into the simulation. Typically, a DPRA is performed by sampling values of a set of parameters and simulating the system behavior for that specific set of parameter values. For complex systems, a major challenge in using DPRA methodologies is to analyze the large number of scenarios generated, where clustering techniques are typically employed to better organize and interpret the data. In this paper, we focus on the analysis of two nuclear simulation datasets that are part of the risk-informed safety margin characterization (RISMC) boiling water reactor (BWR) station blackout (SBO) case study. We provide the domain experts a software tool that encodes traditional and topological clustering techniques within an interactive analysis and visualization environment, for understanding the structures of such high-dimensional nuclear simulation datasets. We demonstrate through our case study that both types of clustering techniques complement each other for enhanced structural understanding of the data.
更多查看译文
关键词
Probabilistic risk assessment,Computational topology,Clustering,High-dimensional data analysis
AI 理解论文
溯源树
样例
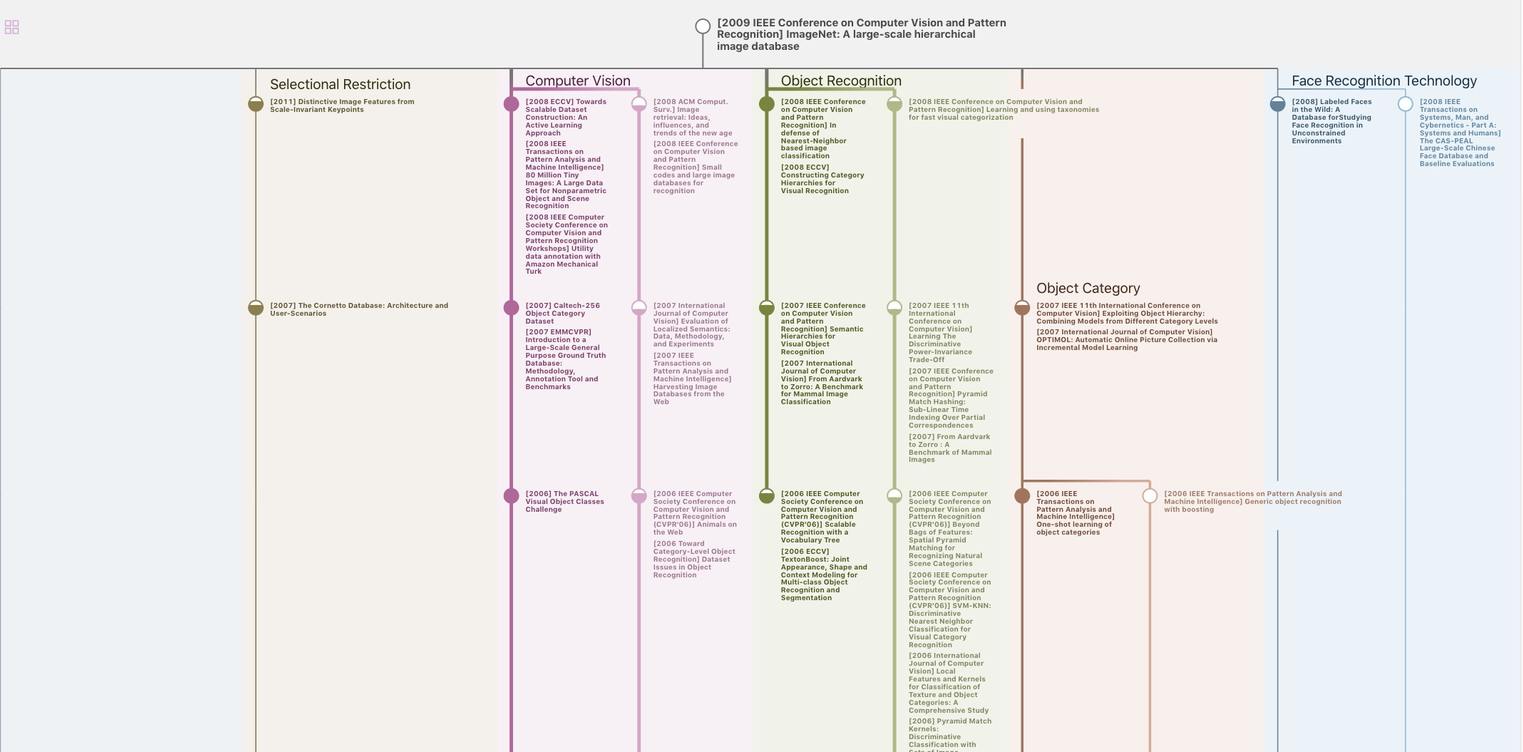
生成溯源树,研究论文发展脉络
Chat Paper
正在生成论文摘要