A Regularization-Based Approach For Unsupervised Image Segmentation
ELEKTROTEHNISKI VESTNIK-ELECTROCHEMICAL REVIEW(2016)
摘要
We propose a novel unsupervised image-segmentation algorithm aiming at segmenting an image into several coherent parts. It requires neither user input nor supervised learning phase and assumes an unknown number of segments. It achieves this by over-segmenting the image into several hundred superpixels iteratively joined on the basis of a discriminative classifier trained on color and texture information obtained from each superpixel. The output of the classifier is regularized by a Markov random field that lends more influence to the neighbouring superpixels that are more similar. In each iteration, the similar superpixels fall under the same label, until only a few coherent regions remain in the image. The algorithm is tested on a standard evaluation data set, where it performs on par with the state-of-the-art algorithms in terms of precision and greatly outperforms the them by reducing the oversegmentation of the object of interest.
更多查看译文
关键词
image segmentation, Markov random field, computer vision
AI 理解论文
溯源树
样例
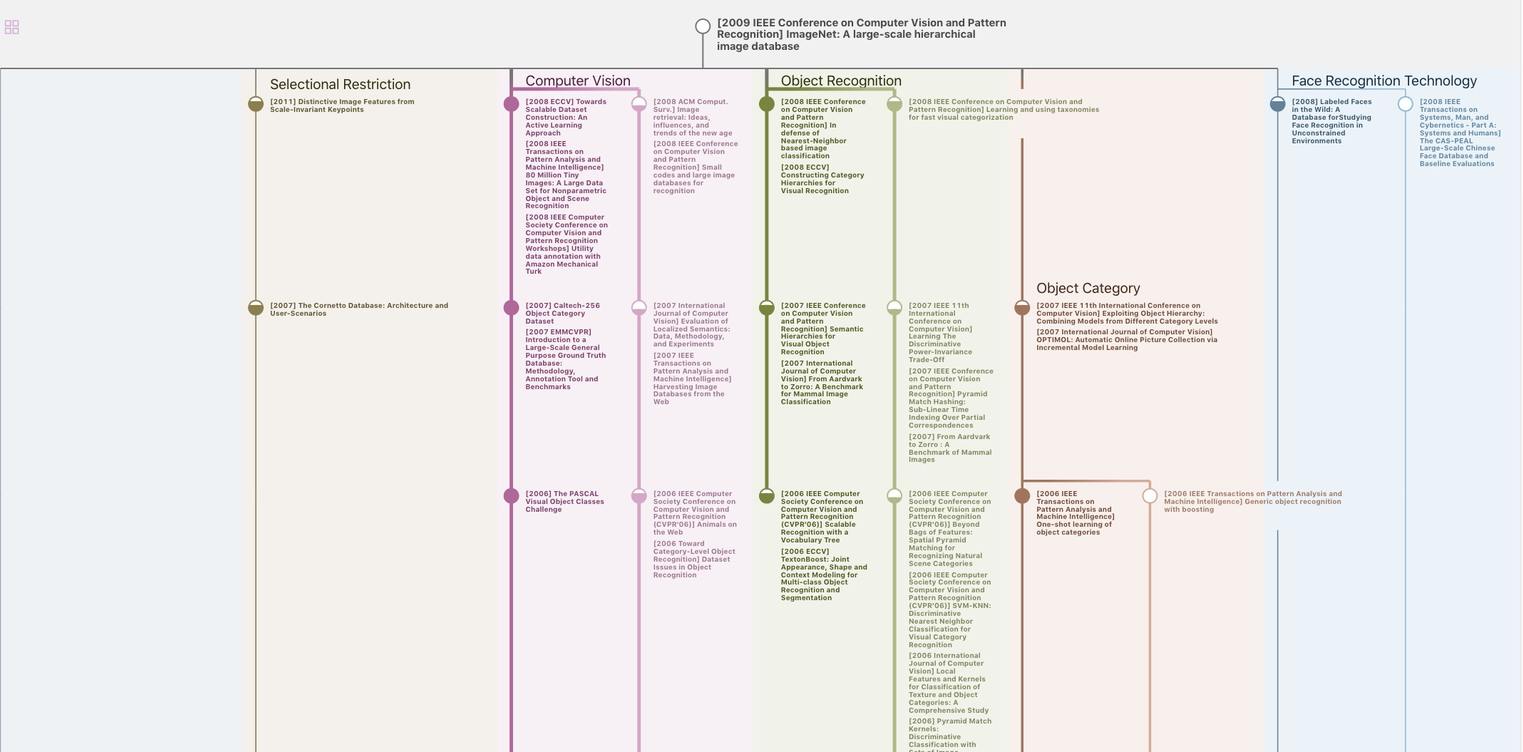
生成溯源树,研究论文发展脉络
Chat Paper
正在生成论文摘要