Loss Functions for Top-k Error: Analysis and Insights
2016 IEEE Conference on Computer Vision and Pattern Recognition (CVPR)(2016)
摘要
In order to push the performance on realistic computer vision tasks, the number of classes in modern benchmark datasets has significantly increased in recent years. This increase in the number of classes comes along with increased ambiguity between the class labels, raising the question if top-1 error is the right performance measure. In this paper, we provide an extensive comparison and evaluation of established multiclass methods comparing their top-k performance both from a practical as well as from a theoretical perspective. Moreover, we introduce novel top-k loss functions as modifications of the softmax and the multiclass SVM losses and provide efficient optimization schemes for them. In the experiments, we compare on various datasets all of the proposed and established methods for top-k error optimization. An interesting insight of this paper is that the softmax loss yields competitive top-k performance for all k simultaneously. For a specific top-k error, our new top-k losses lead typically to further improvements while being faster to train than the softmax.
更多查看译文
关键词
computer vision,class labels,top-k loss functions,multiclass SVM losses,top-k error optimization,softmax loss,top-k performance,support vector machine
AI 理解论文
溯源树
样例
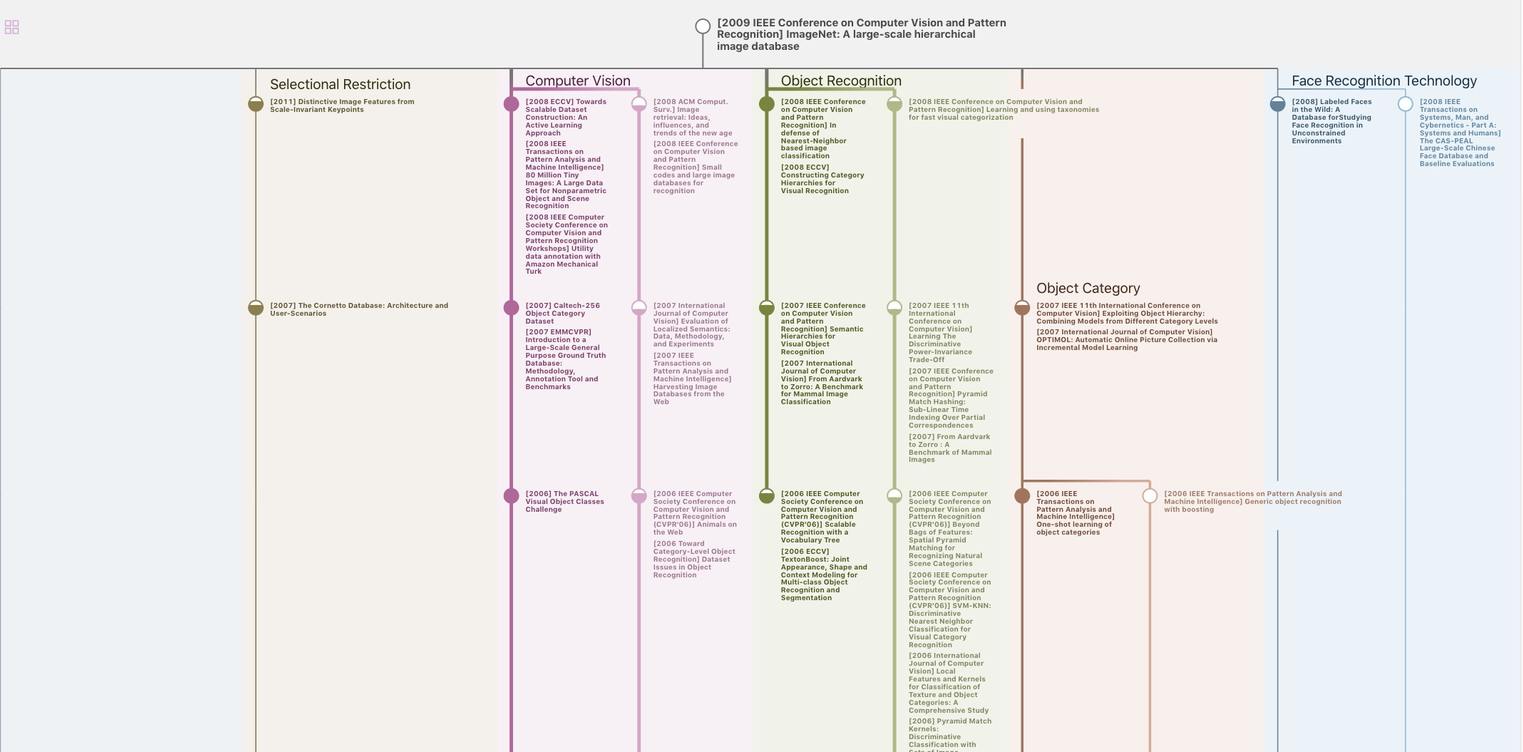
生成溯源树,研究论文发展脉络
Chat Paper
正在生成论文摘要