Visualizing Deep Convolutional Neural Networks Using Natural Pre-images
International Journal of Computer Vision(2016)
摘要
Image representations, from SIFT and bag of visual words to convolutional neural networks (CNNs) are a crucial component of almost all computer vision systems. However, our understanding of them remains limited. In this paper we study several landmark representations, both shallow and deep, by a number of complementary visualization techniques. These visualizations are based on the concept of “natural pre-image”, namely a natural-looking image whose representation has some notable property. We study in particular three such visualizations: inversion, in which the aim is to reconstruct an image from its representation, activation maximization, in which we search for patterns that maximally stimulate a representation component, and caricaturization, in which the visual patterns that a representation detects in an image are exaggerated. We pose these as a regularized energy-minimization framework and demonstrate its generality and effectiveness. In particular, we show that this method can invert representations such as HOG more accurately than recent alternatives while being applicable to CNNs too. Among our findings, we show that several layers in CNNs retain photographically accurate information about the image, with different degrees of geometric and photometric invariance.
更多查看译文
关键词
Visualization,Convolutional neural networks,Pre-image problem
AI 理解论文
溯源树
样例
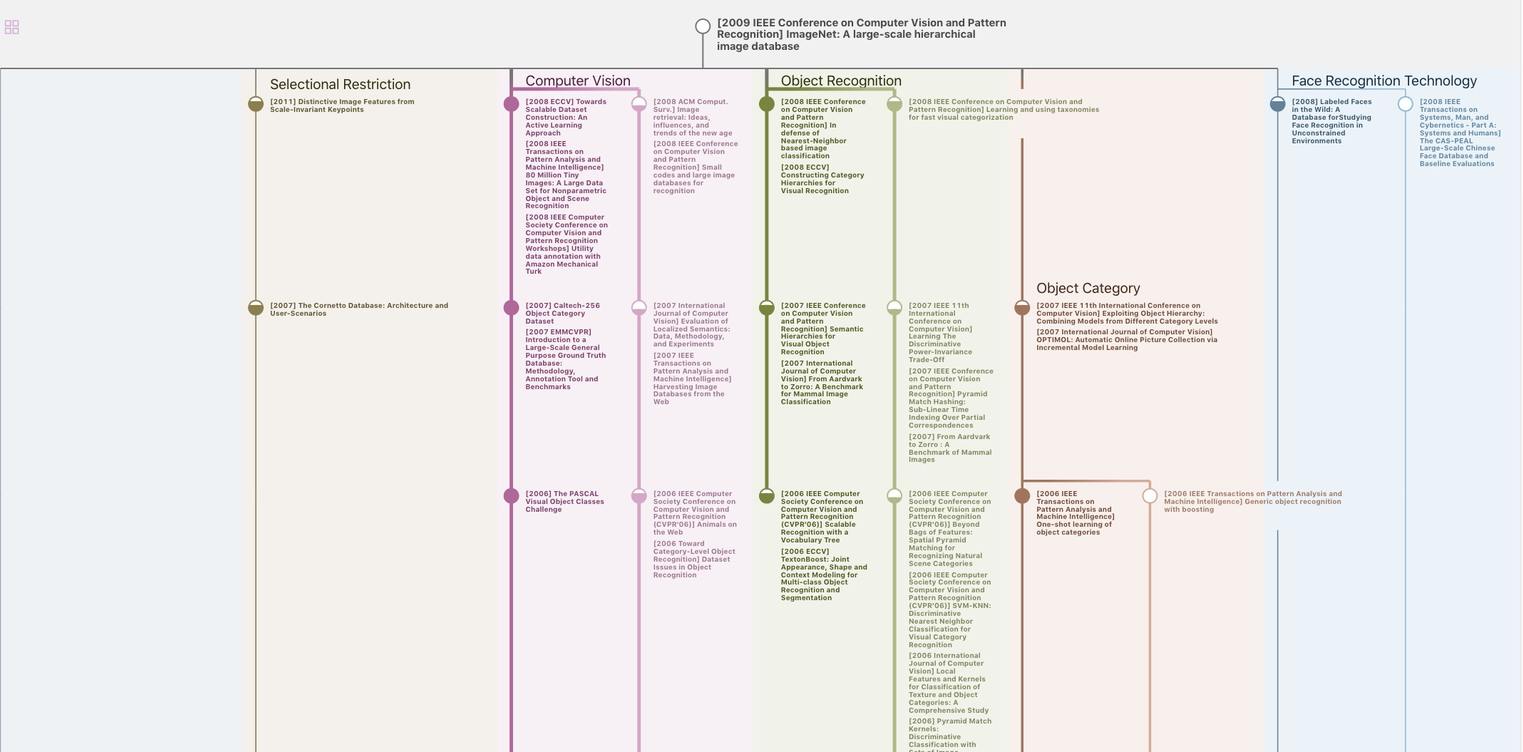
生成溯源树,研究论文发展脉络
Chat Paper
正在生成论文摘要