A New Framework For Distributed Submodular Maximization
2016 IEEE 57th Annual Symposium on Foundations of Computer Science (FOCS)(2016)
摘要
A wide variety of problems in machine learning, including exemplar clustering, document summarization, and sensor placement, can be cast as constrained submodular maximization problems. A lot of recent effort has been devoted to developing distributed algorithms for these problems. However, these results suffer from high number of rounds, suboptimal approximation ratios, or both. We develop a framework for bringing existing algorithms in the sequential setting to the distributed setting, achieving near optimal approximation ratios for many settings in only a constant number of MapReduce rounds. Our techniques also give a fast sequential algorithm for non-monotone maximization subject to a matroid constraint.
更多查看译文
关键词
Distributed submodular maximization,MapReduce,approximation algorithms
AI 理解论文
溯源树
样例
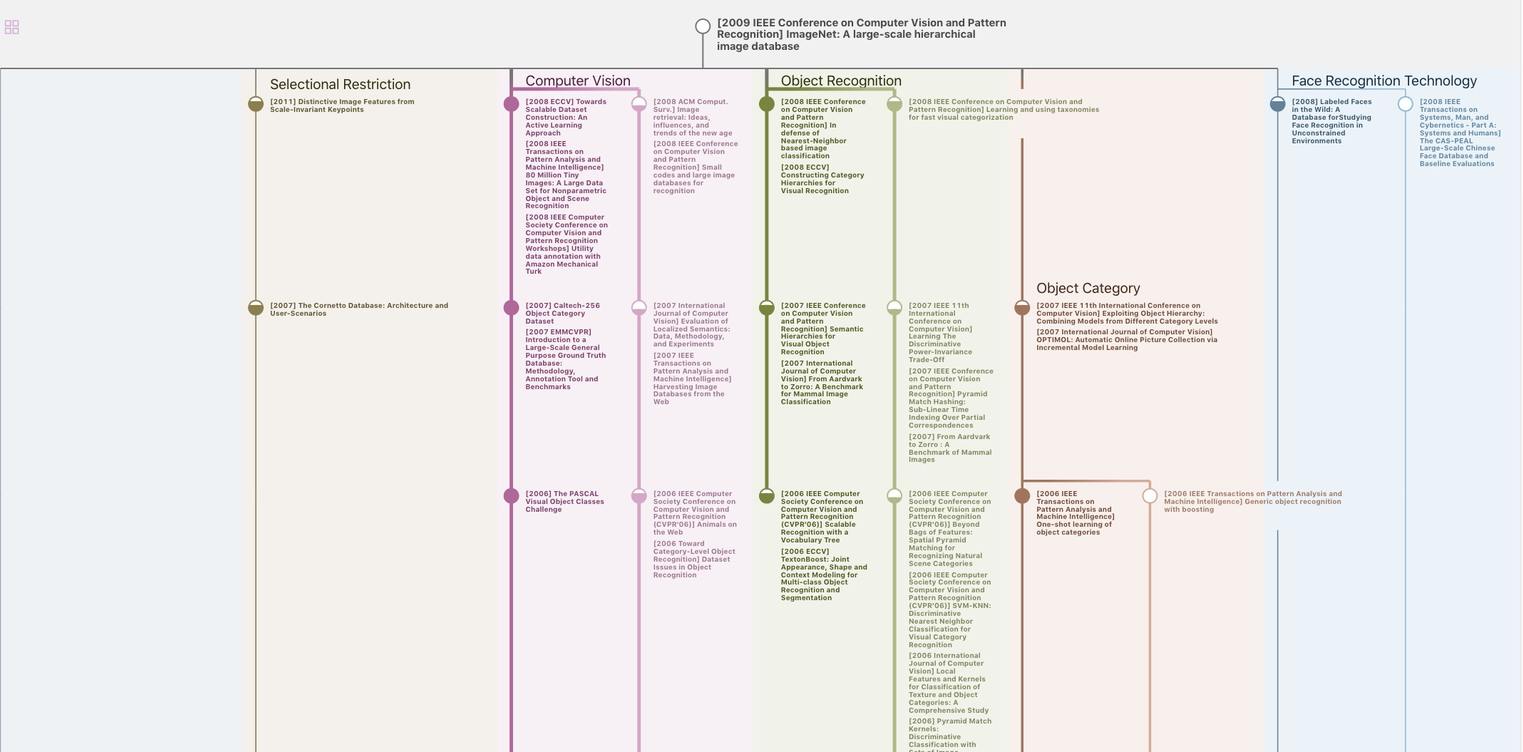
生成溯源树,研究论文发展脉络
Chat Paper
正在生成论文摘要