Mondrian Forests for Large-Scale Regression when Uncertainty Matters
JMLR Workshop and Conference Proceedings(2016)
摘要
Many real-world regression problems demand a measure of the uncertainty associated with each prediction. Standard decision forests deliver efficient state-of-the-art predictive performance, but high-quality uncertainty estimates are lacking. Gaussian processes (GPs) deliver uncertainty estimates, but scaling GPs to large-scale data sets comes at the cost of approximating the uncertainty estimates. We extend Mondrian forests, first proposed by Lakshminarayanan et al. (2014) for classification problems, to the large-scale non-parametric regression setting. Using a novel hierarchical Gaussian prior that dovetails with the Mondrian forest framework, we obtain principled uncertainty estimates, while still retaining the computational advantages of decision forests. Through a combination of illustrative examples, real-world large-scale datasets, and Bayesian optimization benchmarks, we demonstrate that Mondrian forests outperform approximate GPs on large-scale regression tasks and deliver better-calibrated uncertainty assessments
更多查看译文
关键词
mondrian forests,uncertainty,large-scale
AI 理解论文
溯源树
样例
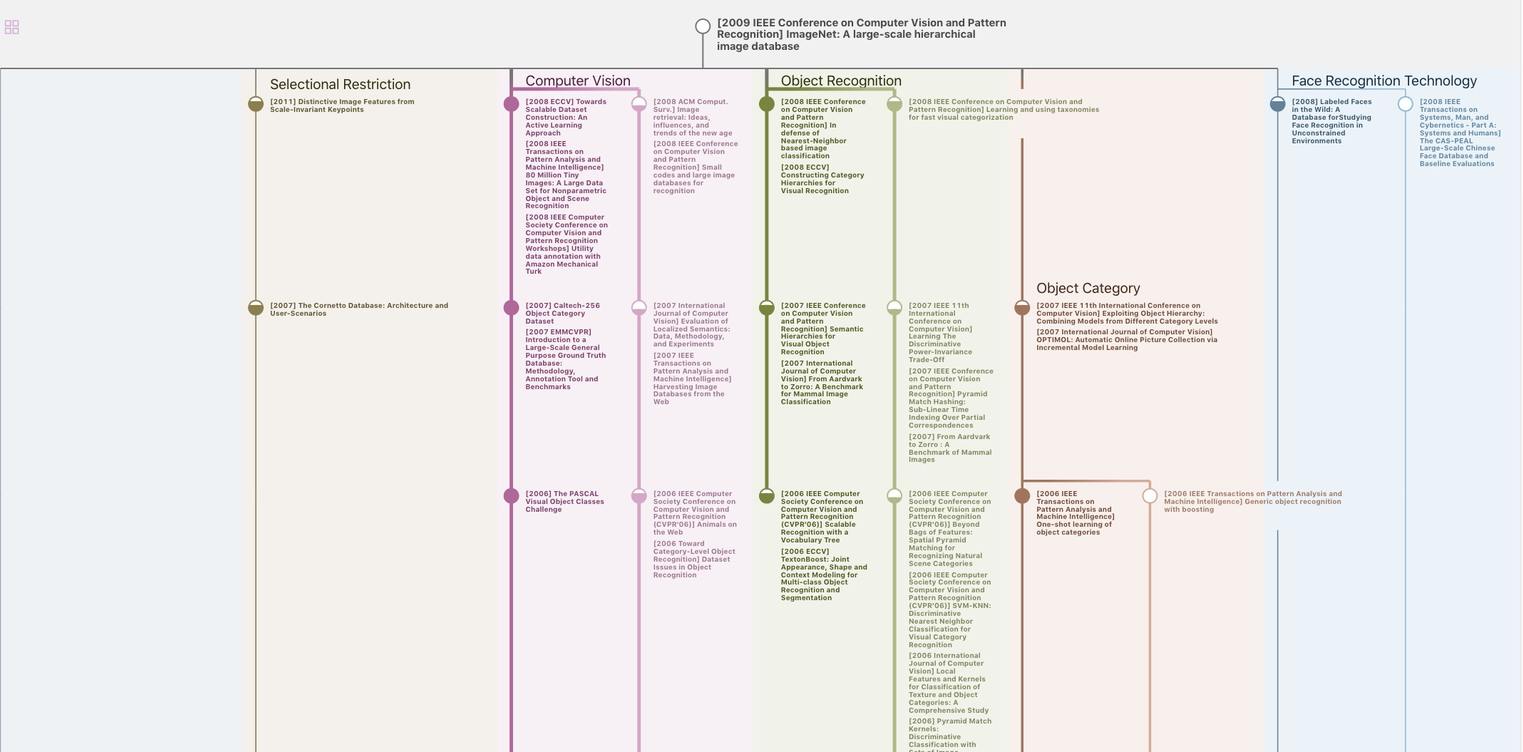
生成溯源树,研究论文发展脉络
Chat Paper
正在生成论文摘要