Object-Proposal Evaluation Protocol is ‘Gameable’
2016 IEEE Conference on Computer Vision and Pattern Recognition (CVPR)(2016)
摘要
Object proposals have quickly become the de-facto preprocessing step in a number of vision pipelines (for object detection, object discovery, and other tasks). Their performance is usually evaluated on partially annotated datasets. In this paper, we argue that the choice of using a partially annotated dataset for evaluation of object proposals is problematic - as we demonstrate via a thought experiment, the evaluation protocol is 'gameable', in the sense that progress under this protocol does not necessarily correspond to a "better" category independent object proposal algorithm. To alleviate this problem, we: (1) Introduce a nearly-fully annotated version of PASCAL VOC dataset, which serves as a test-bed to check if object proposal techniques are overfitting to a particular list of categories. (2) Perform an exhaustive evaluation of object proposal methods on our introduced nearly-fully annotated PASCAL dataset and perform cross-dataset generalization experiments, and (3) Introduce a diagnostic experiment to detect the bias capacity in an object proposal algorithm. This tool circumvents the need to collect a densely annotated dataset, which can be expensive and cumbersome to collect. Finally, we have released an easy-to-use toolbox which combines various publicly available implementations of object proposal algorithms which standardizes the proposal generation and evaluation so that new methods can be added and evaluated on different datasets. We hope that the results presented in the paper will motivate the community to test the category independence of various object proposal methods by carefully choosing the evaluation protocol.
更多查看译文
关键词
object-proposal evaluation protocol,Gameable protocol,vision pipelines,object detection,object discovery,partially annotated datasets,PASCAL VOC dataset,cross-dataset generalization experiments,easy-to-use toolbox,publicly available implementations
AI 理解论文
溯源树
样例
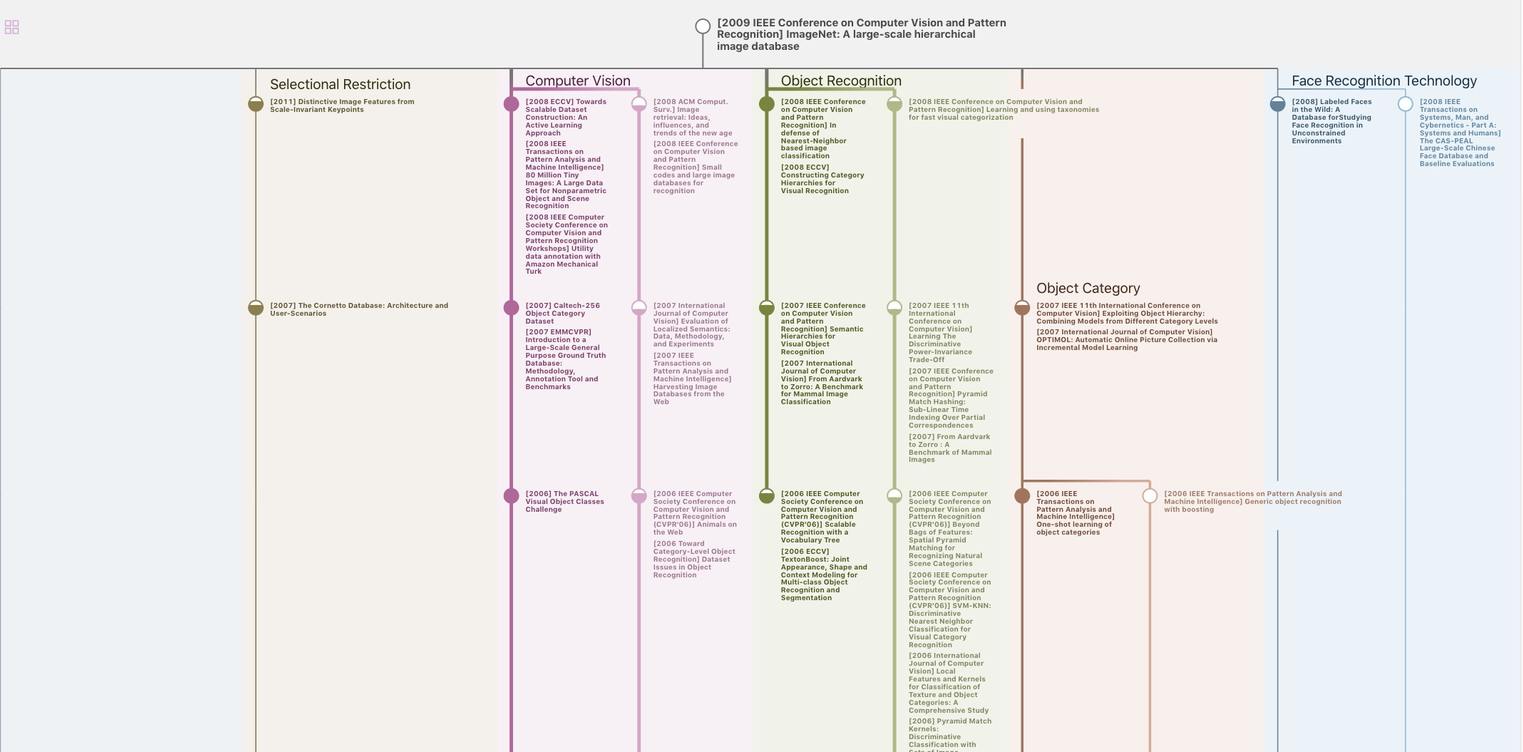
生成溯源树,研究论文发展脉络
Chat Paper
正在生成论文摘要