Joint Source Selection and Data Extrapolation in Social Sensing for Disaster Response.
arXiv: Social and Information Networks(2015)
摘要
This paper complements the large body of social sensing literature by developing means for augmenting sensing data with inference results that fill-in missing pieces. It specifically explores the synergy between (i) inference techniques used for filling-in missing pieces and (ii) source selection techniques used to determine which pieces to retrieve in order to improve inference results. We focus on prediction in disaster scenarios, where disruptive trend changes occur. We first discuss our previous conference study that compared a set of prediction heuristics and developed a hybrid prediction algorithm. We then enhance the prediction scheme by considering algorithms for sensor selection that improve inference quality. Our proposed source selection and extrapolation algorithms are tested using data collected during the New York City crisis in the aftermath of Hurricane Sandy in November 2012. The evaluation results show that consistently good predictions are achieved. The work is notable for addressing the bi-modal nature of damage propagation in complex systems subjected to stress, where periods of calm are interspersed with periods of severe change. It is novel in offering a new solution to the problem that jointly leverages source selection and extrapolation components thereby improving the results.
更多查看译文
AI 理解论文
溯源树
样例
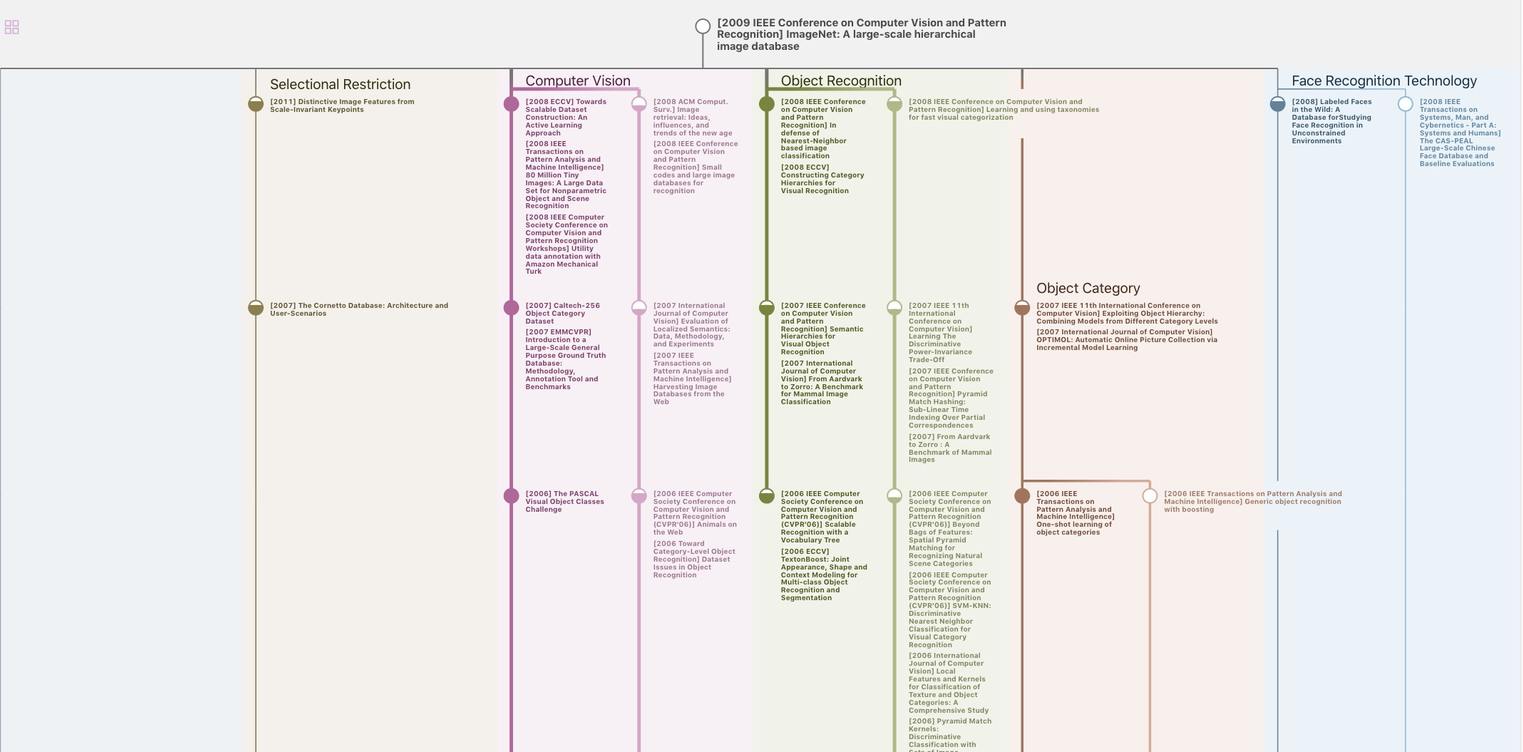
生成溯源树,研究论文发展脉络
Chat Paper
正在生成论文摘要