New a priori and a posteriori probabilistic bounds for robust counterpart optimization: II. A priori bounds for known symmetric and asymmetric probability distributions.
Computers & Chemical Engineering(2017)
摘要
Abstract When optimization problems contain uncertain parameters, their nominal solutions may prove to be overly optimistic or even rendered infeasible given the actual parameter realizations. The application of probabilistic bounds in constructing the robust counterpart formulation of a model under uncertainty can greatly reduce the conservatism of traditional worst-case robust optimization. In Part I, we derived new a priori and a posteriori bounds on the probability of constraint violation for constraints with uncertain parameters whose distributions were unknown. Here, we first present new a priori bounds applicable to uncertain constraints with linearly participating uncertain parameters whose distributions are known or conservatively approximated. We then extend the robust counterpart optimization methodology by allowing attributed known distributions to be symmetric or asymmetric. The new methods greatly reduce the conservatism and significantly augment the performance and applicability of robust counterpart optimization. A mixed-integer linear optimization example and a multiperiod planning problem demonstrate the improvements of the new a priori bounds relative to existing bounds.
更多查看译文
关键词
Robust counterpart optimization,Optimization under uncertainty,Probabilistic bounds,Mathematical modeling
AI 理解论文
溯源树
样例
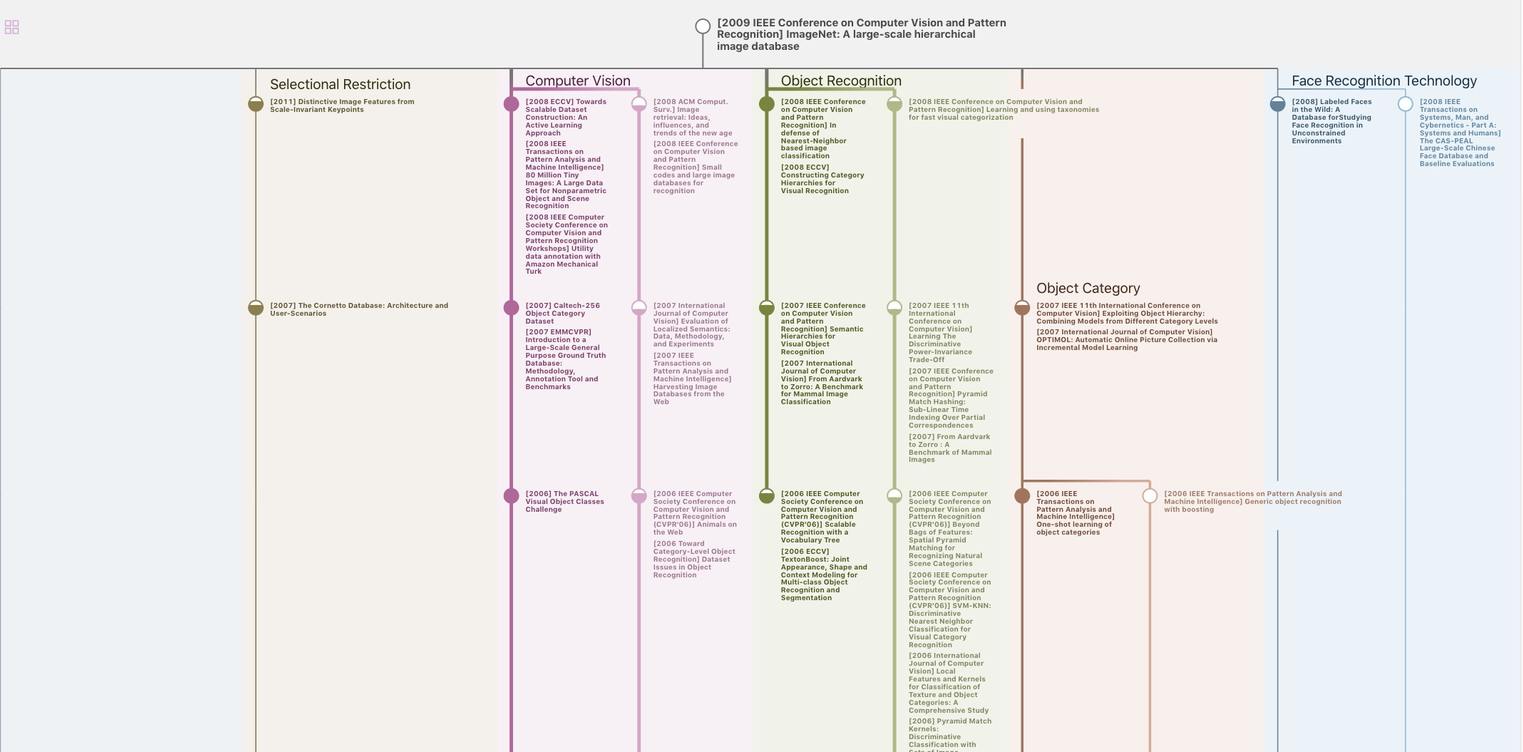
生成溯源树,研究论文发展脉络
Chat Paper
正在生成论文摘要