A Semi-Supervised Learning Algorithm For Data Classification
INTERNATIONAL JOURNAL OF PATTERN RECOGNITION AND ARTIFICIAL INTELLIGENCE(2015)
Abstract
In this study, a semi-supervised learning algorithm for data classification and defect type recognition system for a 25 kV cross-linked polyethylene (XLPE) underground power cable joint is proposed. The proposed algorithm integrates the fuzzy-rough set and shared nearest neighbors (SNN) method for the assignment of labels to unlabeled data. As such, the data set is divided into two subsets: one is labeled and the other is unlabeled. The SNN is adopted for measuring the similarity between unlabeled data and labeled data subsets. Then, according to the levels of similarity, the fuzzy-rough set algorithm is adopted for assigning labels to the unlabeled data. A defect type recognition system XLPE cable classification problem is proposed in order to test the proposed algorithm. To demonstrate the performance of the proposed method, the proposed algorithm is applied to two well-known data sets. The experimental results show that the proposed algorithm can obtain outstanding levels of performance.
MoreTranslated text
Key words
Semi-supervised clustering algorithm, shared nearest neighbors, fuzzy-rough set, defect type recognition system, cross-linked polyethylene
AI Read Science
Must-Reading Tree
Example
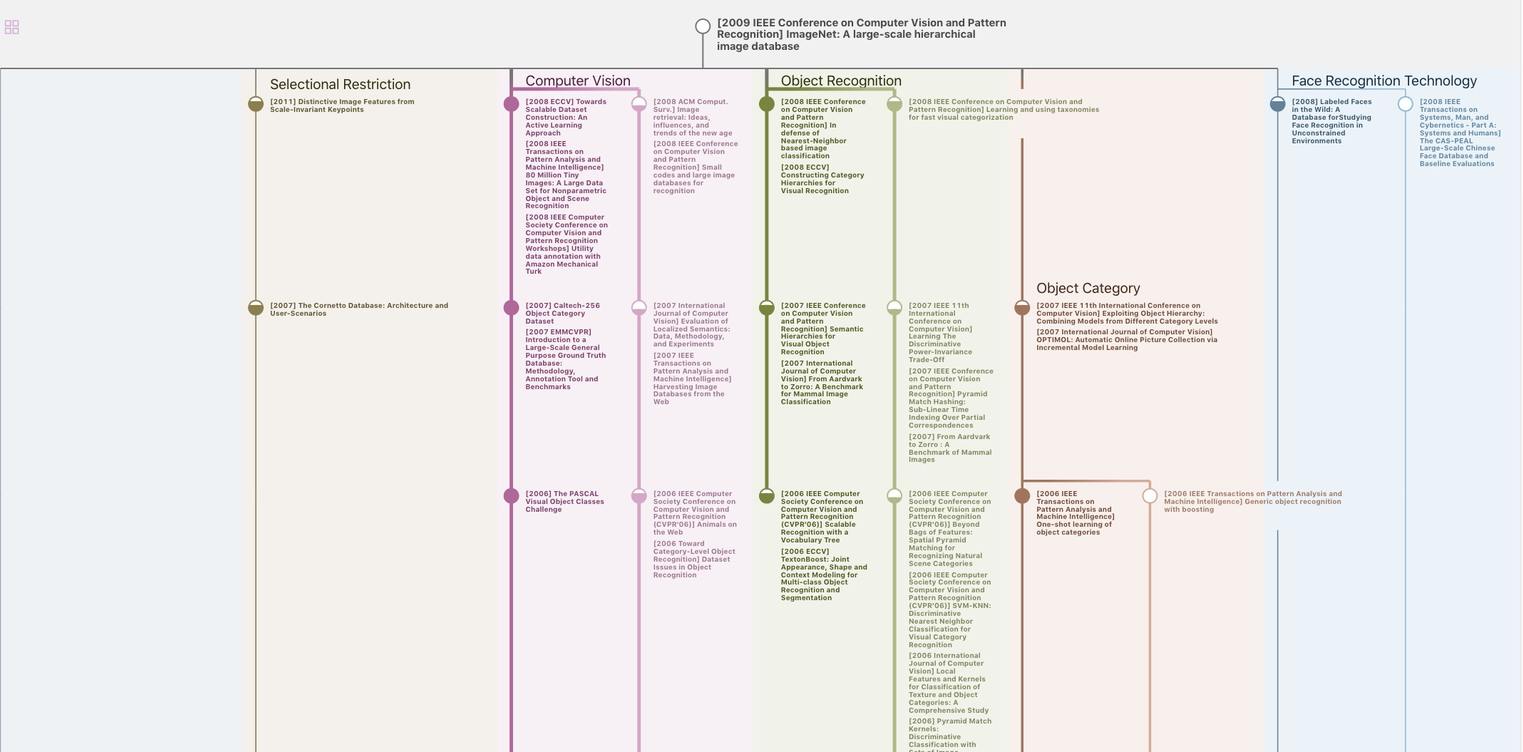
Generate MRT to find the research sequence of this paper
Chat Paper
Summary is being generated by the instructions you defined