Automatically Estimating Isax Parameters
Intelligent Data Analysis(2015)
摘要
The Symbolic Aggregate Approximation (iSAX) is widely used in time series data mining. Its popularity arises from the fact that it largely reduces time series size, it is symbolic, allows lower bounding and is space efficient. However, it requires setting two parameters: the symbolic length and alphabet size, which limits the applicability of the technique. The optimal parameter values are highly application dependent. Typically, they are either set to a fixed value or experimentally probed for the best configuration. In this work we propose an approach to automatically estimate iSAX's parameters. The approach - AutoiSAX - not only discovers the best parameter setting for each time series in the database, but also finds the alphabet size for each iSAX symbol within the same word. It is based on simple and intuitive ideas from time series complexity and statistics. The technique can be smoothly embedded in existing data mining tasks as an efficient sub-routine. We analyze its impact in visualization interpretability, classification accuracy and motif mining. Our contribution aims to make iSAX a more general approach as it evolves towards a parameter-free method.
更多查看译文
关键词
Time series,data mining,representation,iSAX,parameters
AI 理解论文
溯源树
样例
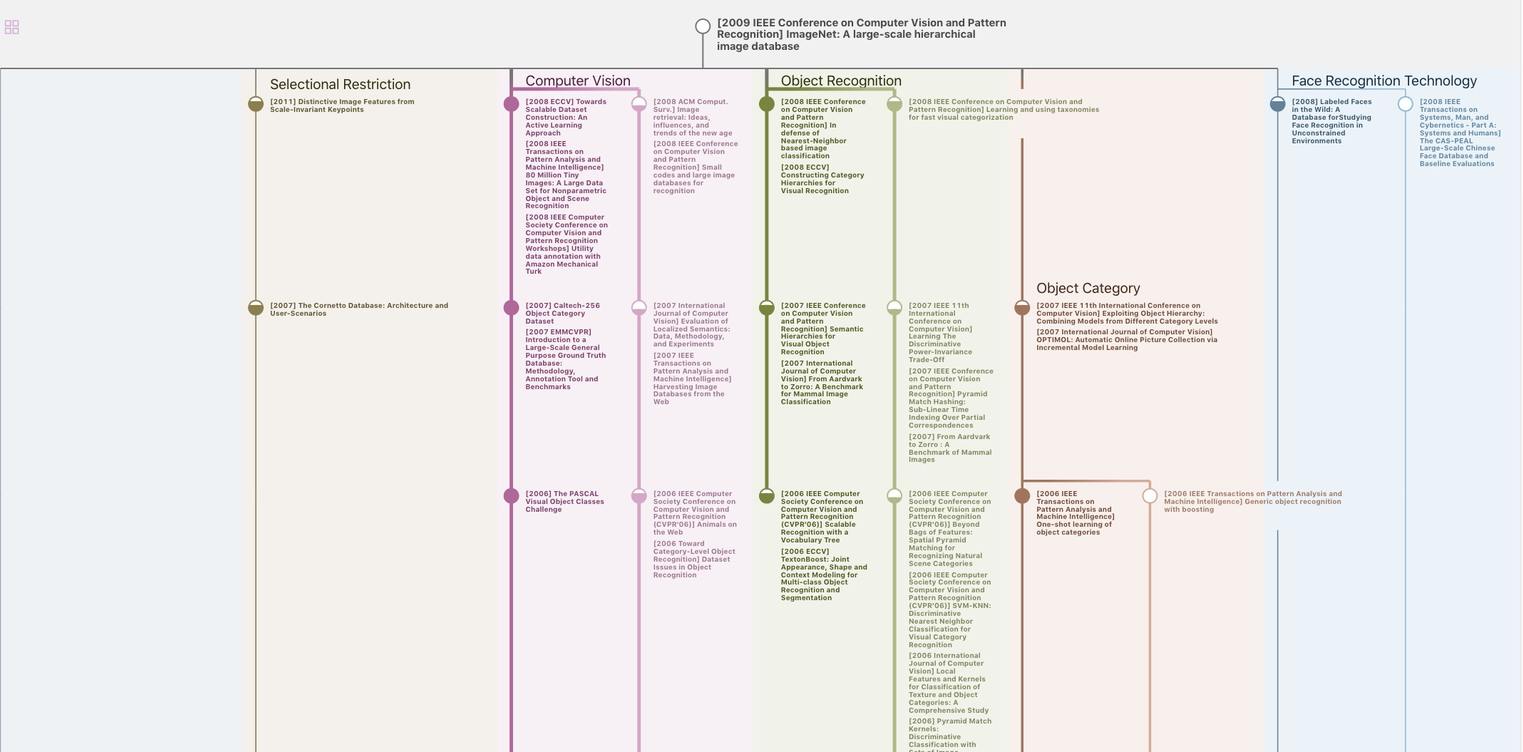
生成溯源树,研究论文发展脉络
Chat Paper
正在生成论文摘要