Using biologically inspired computing to effectively improve prediction models.
International Journal of Hybrid Intelligent Systems(2016)
Abstract
The complexity of certain problems causes that classical
methods for finding exact solutions have some limitations. In this paper we
propose an incremental heterogeneous ensemble model for time series
prediction where biologically inspired algorithms offer a suitable
alternative. Ensemble learning techniques are advantageously used for
improving performance of various prediction methods. The quality of this
kind of machine learning approaches depends on proper combination of used
methods. The influence of each of the used method can change on the fly and
is determined by proper choice of its weights. Finding optimal weights in
prediction methods represents typical optimization problem with objective
function reflecting error minimization, where biologically inspired
algorithms can be used. In the proposed paper, we study several biologically
inspired algorithms in the process of weights optimization. We investigate
and compare ensembles using base models and ensembles optimized by
biologically inspired algorithms. We demonstrate that the ensemble learning
prediction models optimized by biologically inspired algorithms outperformed
the base prediction methods. We present performance and accuracy results of
proposed ensemble models that were evaluated on power load datasets with
concept drifts.
MoreTranslated text
Key words
Ensemble learning,time series prediction,biologically inspired algorithms,power load prediction
AI Read Science
Must-Reading Tree
Example
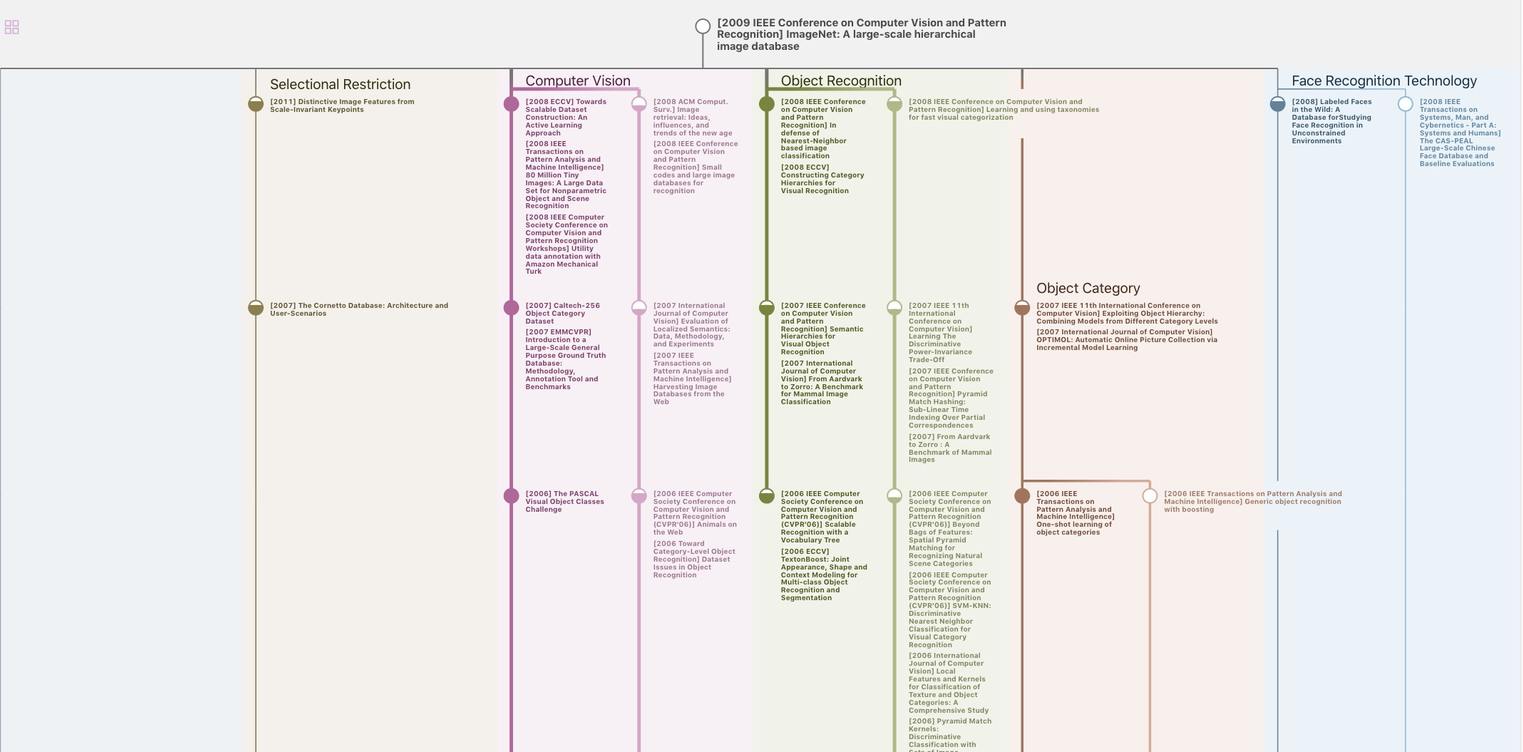
Generate MRT to find the research sequence of this paper
Chat Paper
Summary is being generated by the instructions you defined